A Scalable and Low Power DCNN for Multimodal Data Classification
2018 International Conference on ReConFigurable Computing and FPGAs (ReConFig)(2018)
摘要
This paper presents SensorNet which is a scalable and low power embedded Deep Convolutional Neural Network (DCNN), designed to classify multimodal time series signals. Time series signals generated by different sensor modalities with different sampling rates are first converted to images (2-D signals) and then DCNN is utilized to automatically learn shared features in the images and perform the classification. SensorNet is scalable with respect to different types of multi-channel time series data, and does not require expert knowledge for extracting features for each sensor data. Additionally, it can achieve very high detection accuracy for different case studies, and has a very efficient architecture which makes it suitable to be employed at IoT and wearable devices. A custom low power hardware architecture is also designed for the efficient deployment of SensorNet at embedded real-time systems. SensorNet performance is evaluated using three different case studies including Physical Activity Monitoring, stand-alone Tongue Drive System (sdTDS) and Stress Detection and it achieves an average detection accuracy of 98%, 96.2% and 94% for each case study, respectively. We implement SensorNet using our custom hardware architecture on Xilinx FPGA (Artix-7) which on average consumes 0.3 mJ energy per classification while meeting all applications time requirements. To further reduce the power consumption, SensorNet is implemented using ASIC at the post layout level in 65-nm CMOS technology which consumes approximately 8$\times$ lower power compared to the FPGA.
更多查看译文
AI 理解论文
溯源树
样例
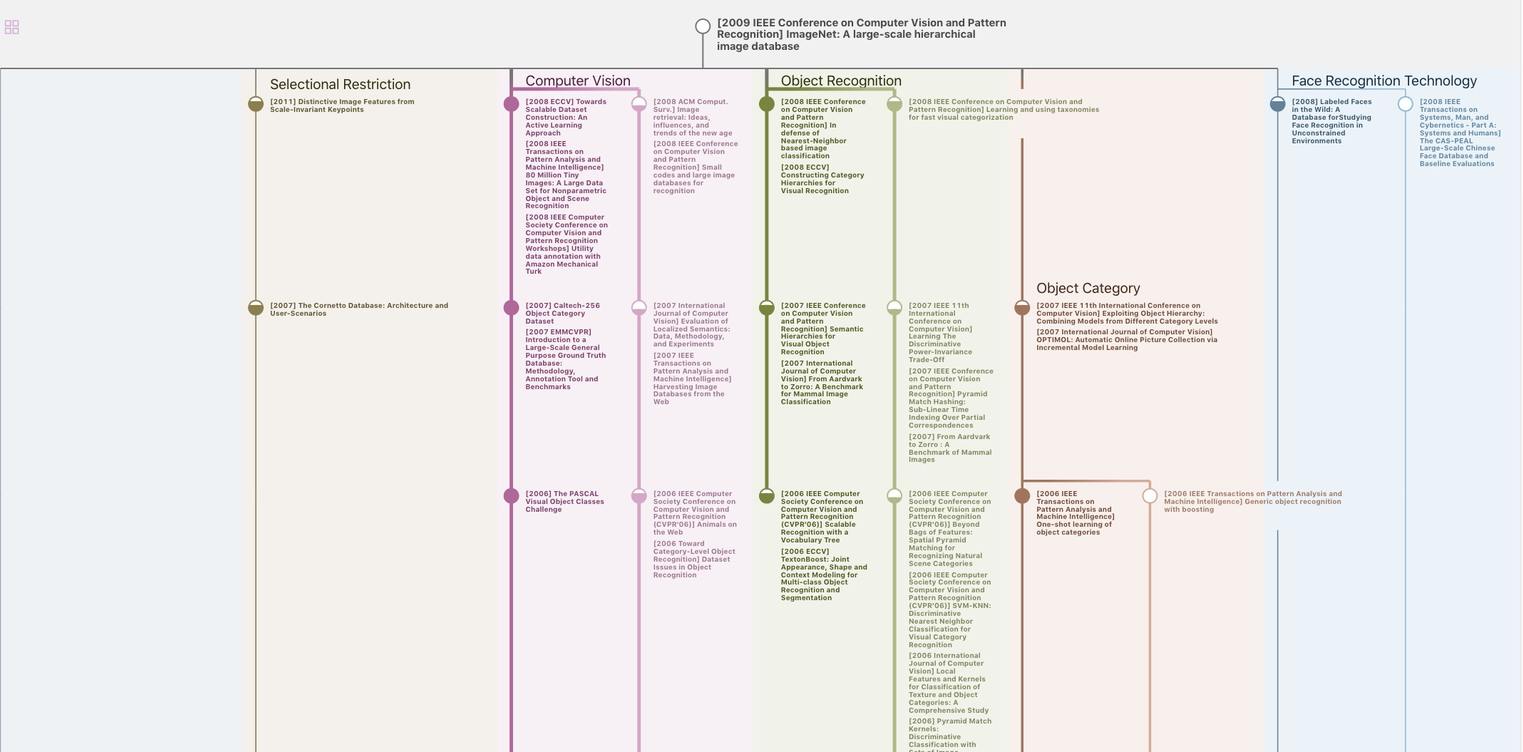
生成溯源树,研究论文发展脉络
Chat Paper
正在生成论文摘要