Learning Network Representations With Neighboring Affinity
2018 18TH IEEE INTERNATIONAL CONFERENCE ON DATA MINING WORKSHOPS (ICDMW)(2018)
摘要
This paper studies the problem of representing networks in a low-dimensional vector space, which is critical in network node classification, link prediction, and recommender systems. We observe that neighbors of a given node often contain rich contextual information which reveals the shared patterns that could be used to learn sparse network representations. Under this observation, we define a new similarity function to describe the similarity between nodes in networks for utilizing the aggregated effect of neighboring information. Furthermore, we propose Neighboring Affinity based neTwork Embedding (NATE), which explicitly incorporates the new similarity into a loss function for network embedding learning by effectively representing the node relationships in networks. NATE efficiently represents the node relationship in both homogeneous and heterogeneous networks. Experimental results demonstrate that. NATE outperforms the state-of-the-art network embedding techniques on several types of real-world networks in terms of rating prediction and multi label classification, yet with lower computational complexity.
更多查看译文
关键词
Graph representation, Network embedding, Dimension Reduction
AI 理解论文
溯源树
样例
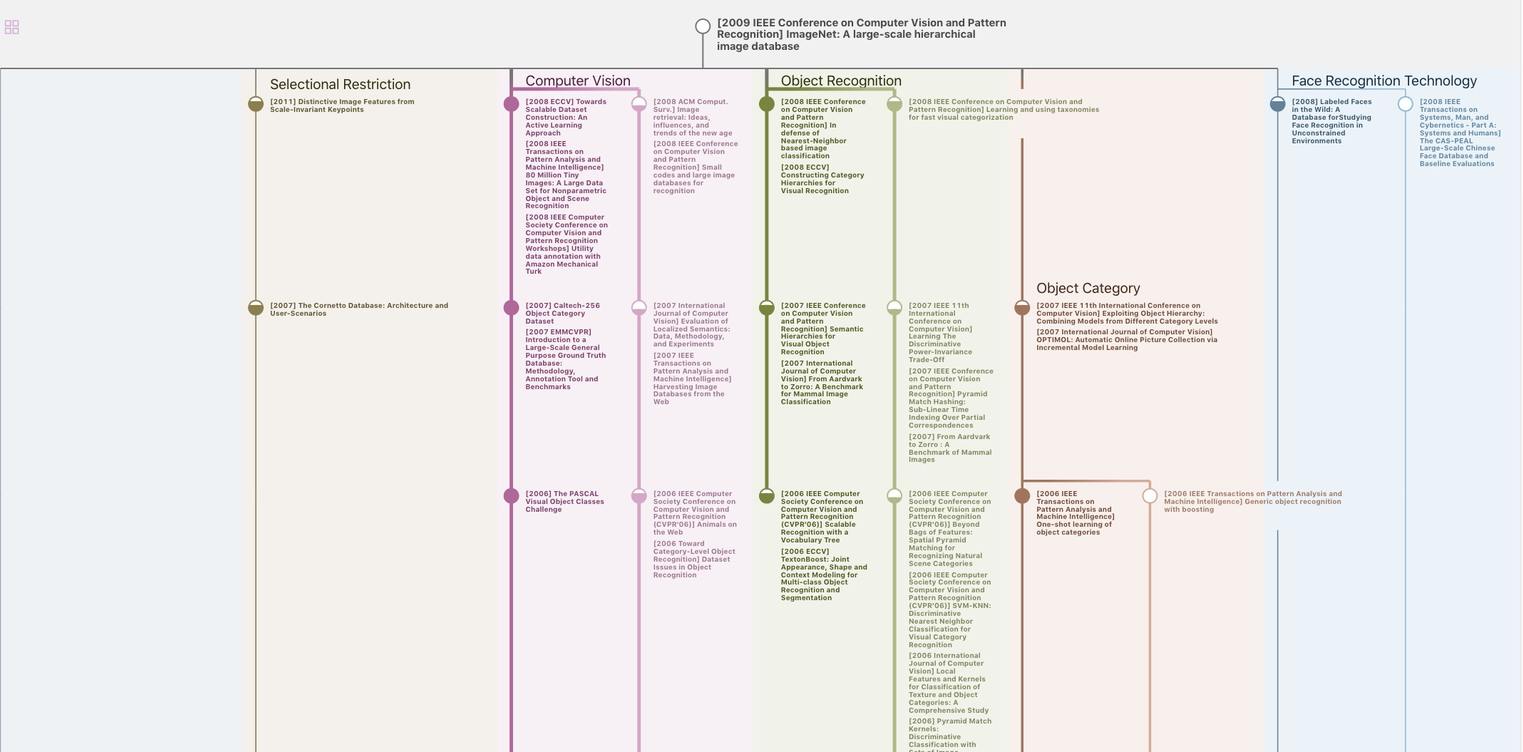
生成溯源树,研究论文发展脉络
Chat Paper
正在生成论文摘要