Learning via Social Preference - A Coarse-to-Fine Training Strategy for Style Transfer Systems.
ICDM Workshops(2018)
摘要
Neural style transfer represented a paradigm shift in image artistic rendering and applications in digital entertainment. Generative adversarial networks are considered as a general solution for style transfer problems. Researchers have explored multiple learning algorithms to increase the learning ability of style transfer networks. However, such research has overlooked an important outside motivator: social preference. Classical style transfer networks are trained offline and worked as a stationary model to transfer photos into stylized images, without any interaction with the environment. Based on the ideas from online training, we propose a new coarse-to-fine training strategy for neural style transfer systems to adapt to social preference change. In coarse stage, a primary model is learned via normal training method. In fine stage, the model is updated with online learning approach and sequentially added new data. We show that our approach exhibits improved performance compared to stationary model from visual effect and reflection of social preference. We conclude that the coarse-to-fine training strategy can improve the output of the generative model in social media environment.
更多查看译文
关键词
Gallium nitride,Training,Data models,Adaptation models,Training data,Generators,Social networking (online)
AI 理解论文
溯源树
样例
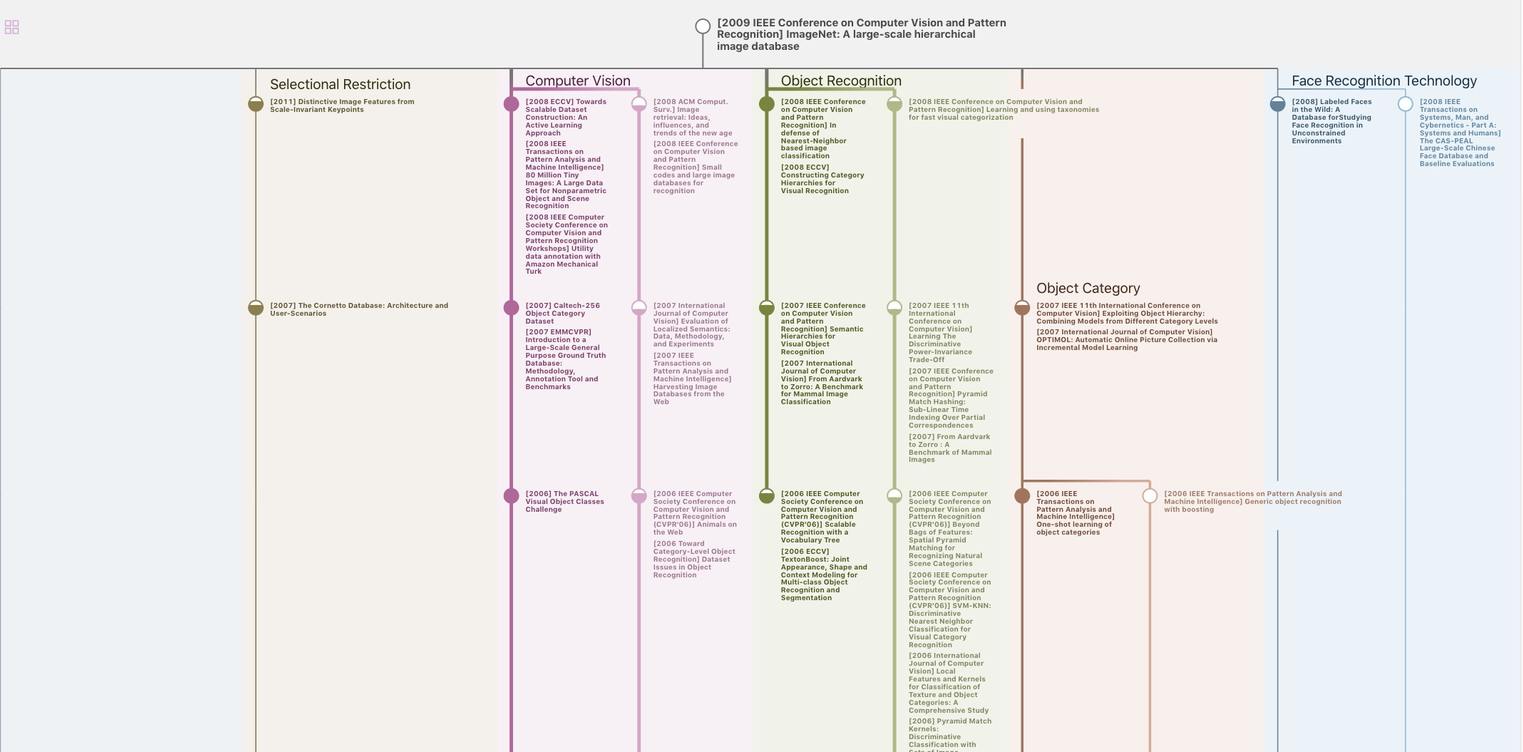
生成溯源树,研究论文发展脉络
Chat Paper
正在生成论文摘要