Deep Reinforcement Learning in Strategic Board Game Environments.
EUMAS(2018)
摘要
In this paper we propose a novel Deep Reinforcement Learning (DRL) algorithm that uses the concept of “action-dependent state features”, and exploits it to approximate the Q-values locally, employing a deep neural network with parallel Long Short Term Memory (LSTM) components, each one responsible for computing an action-related Q-value. As such, all computations occur simultaneously, and there is no need to employ “target” networks and experience replay, which are techniques regularly used in the DRL literature. Moreover, our algorithm does not require previous training experiences, but trains itself online during game play. We tested our approach in the Settlers Of Catan multi-player strategic board game. Our results confirm the effectiveness of our approach, since it outperforms several competitors, including the state-of-the-art jSettler heuristic algorithm devised for this particular domain.
更多查看译文
关键词
Deep Reinforcement Learning, Strategic board games
AI 理解论文
溯源树
样例
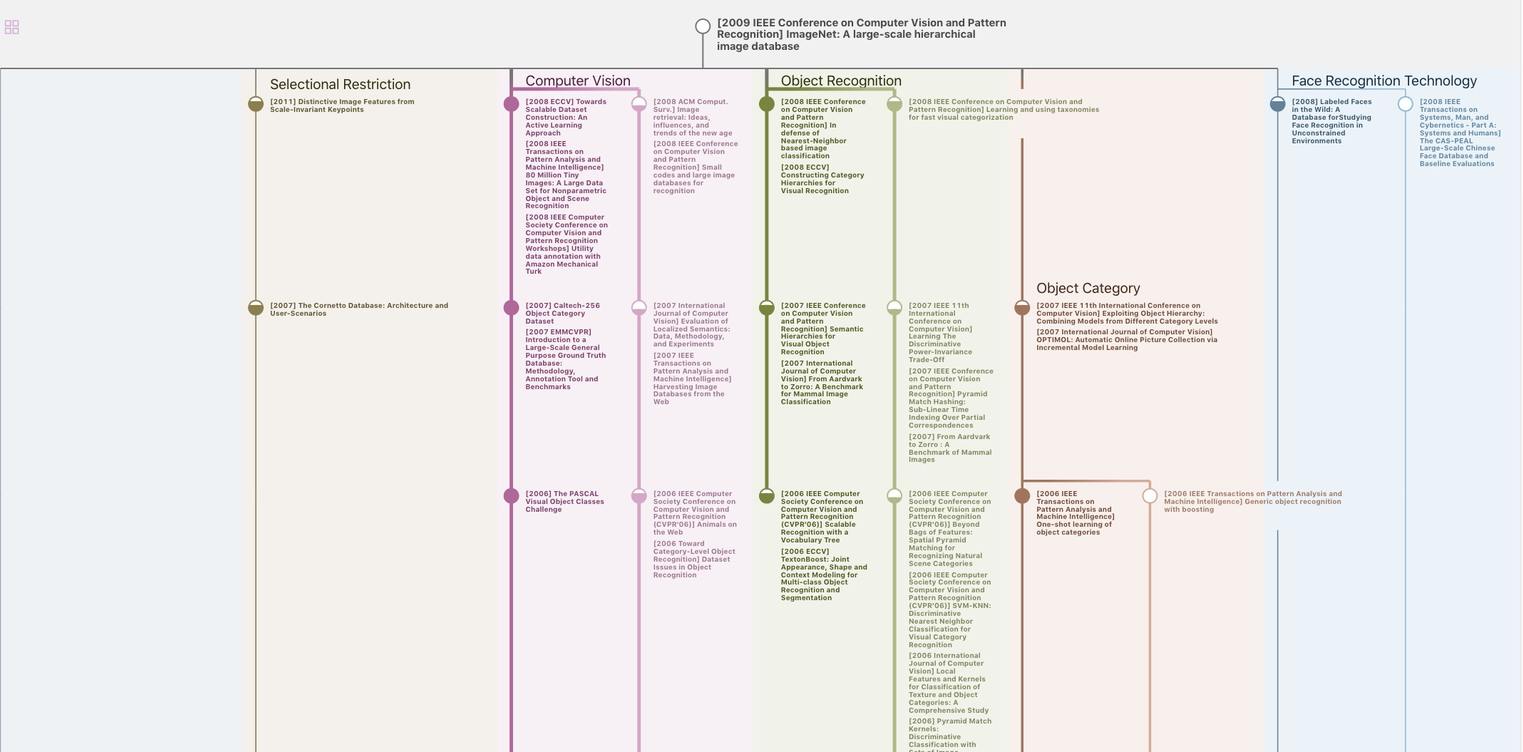
生成溯源树,研究论文发展脉络
Chat Paper
正在生成论文摘要