Sentiment Lexicon Construction With Hierarchical Supervision Topic Model
IEEE/ACM Transactions on Audio, Speech, and Language Processing(2019)
摘要
In this paper, we propose a novel hierarchical supervision topic model to construct a topic-adaptive sentiment lexicon (TaSL) for higher-level classification tasks. It is widely recognized that sentiment lexicon as a useful prior knowledge is crucial in sentiment analysis or opinion mining. However, many existing sentiment lexicons are constructed ignoring the variability of the sentiment polarities of words in different topics or domains. For example, the word “amazing” can refer to causing great surprise or wonder but can also refer to very impressive and excellent. In TaSL, we solve this issue by jointly considering the topics and sentiments of words. Documents are represented by multiple pairs of topics and sentiments, where each pair is characterized by a multinomial distribution over words. Meanwhile, this generating process is supervised under hierarchical supervision information of documents and words. The main advantage of TaSL is that the sentiment polarity of each word in different topics can be sufficiently captured. This model is beneficial to construct a domain-specific sentiment lexicon and then effectively improve the performance of sentiment classification. Extensive experimental results on four publicly available datasets,
MR
,
OMD
,
semEval13A
, and
semEval16B
were presented to demonstrate the usefulness of the proposed approach. The results have shown that TaSL performs better than the existing manual sentiment lexicon (MPQA), the topic model based domain-specific lexicon (ssLDA), the expanded lexicons(Weka-ED, Weka-STS, NRC, Liu's), and deep neural network based lexicons (nnLexicon, HIT, HSSWE).
更多查看译文
关键词
Speech processing,Sentiment analysis,Modeling,Probabilistic logic,Sun,Neural networks,Motion pictures
AI 理解论文
溯源树
样例
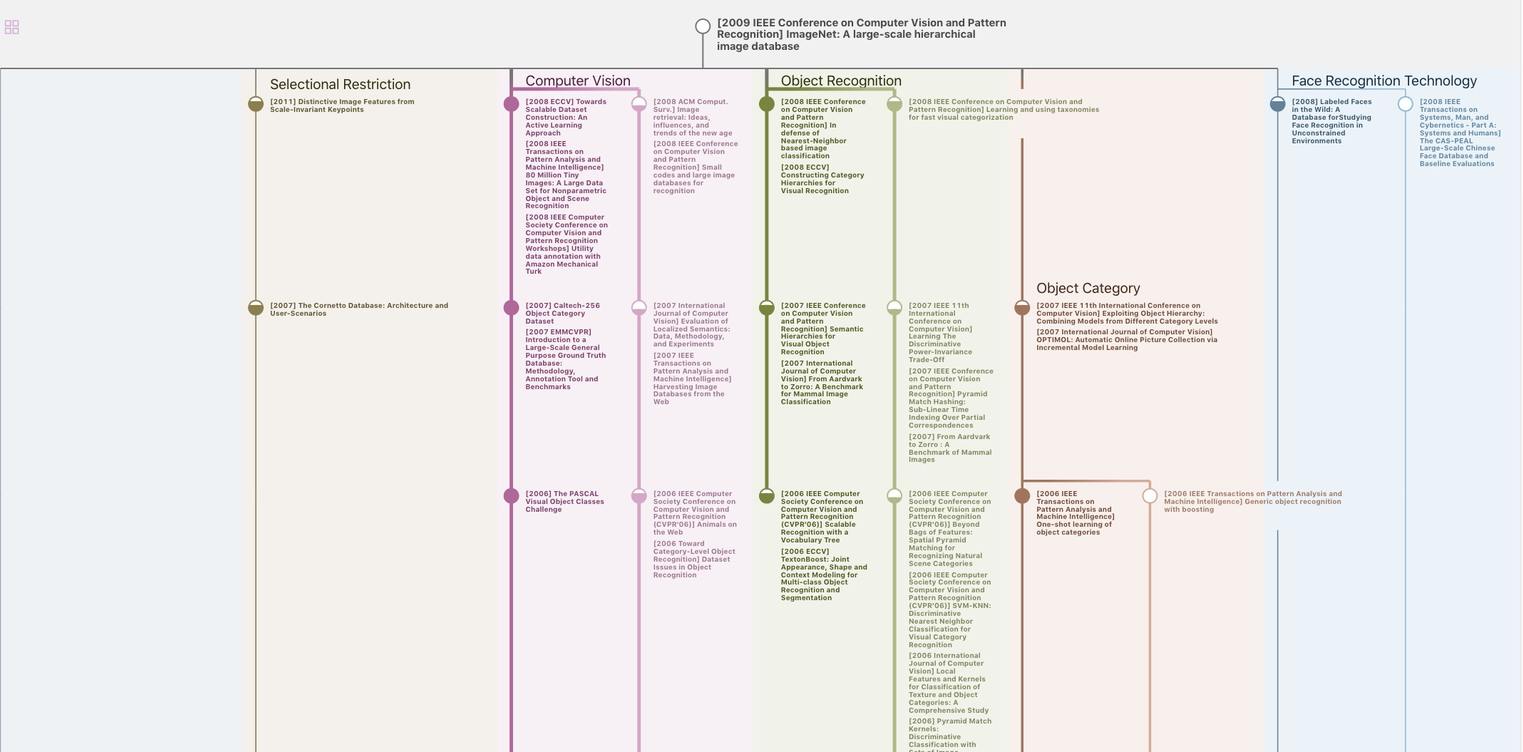
生成溯源树,研究论文发展脉络
Chat Paper
正在生成论文摘要