Elastic-net regularized latent factor analysis-based models for recommender systems.
Neurocomputing(2019)
摘要
Latent factor analysis (LFA)-based models are highly efficient in recommender systems. The problem of LFA is defined on high-dimensional and sparse (HiDS) matrices corresponding to relationships among numerous entities in industrial applications. It is ill-posed without a unique and optimal solution, making regularization vital in improving the generality of an LFA-based model. Current models mostly adopt l2-norm-based regularization, which cannot regularize the latent factor distributions. For addressing this issue, this work applies the elastic-net-based regularization to an LFA-based model, thereby achieving an elastic-net regularized latent factor analysis-based (ERLFA) model. We further adopt two efficient learning algorithms, i.e., forward-looking sub-gradients and forward-backward splitting and stochastic proximal gradient descent, to train desired latent factors in an ERLFA-based model, resulting in two novel ERLFA-based models relying on different learning schemes. Experimental results on four large industrial datasets show that by regularizing the latent factor distribution, the proposed ERLFA-based models are able to achieve high prediction accuracy for missing data of an HiDS matrix without additional computational burden.
更多查看译文
关键词
Big data,Recommender systems,Collaborative filtering,Latent factor analysis,Elastic-net,Regularization,Latent factor distribution
AI 理解论文
溯源树
样例
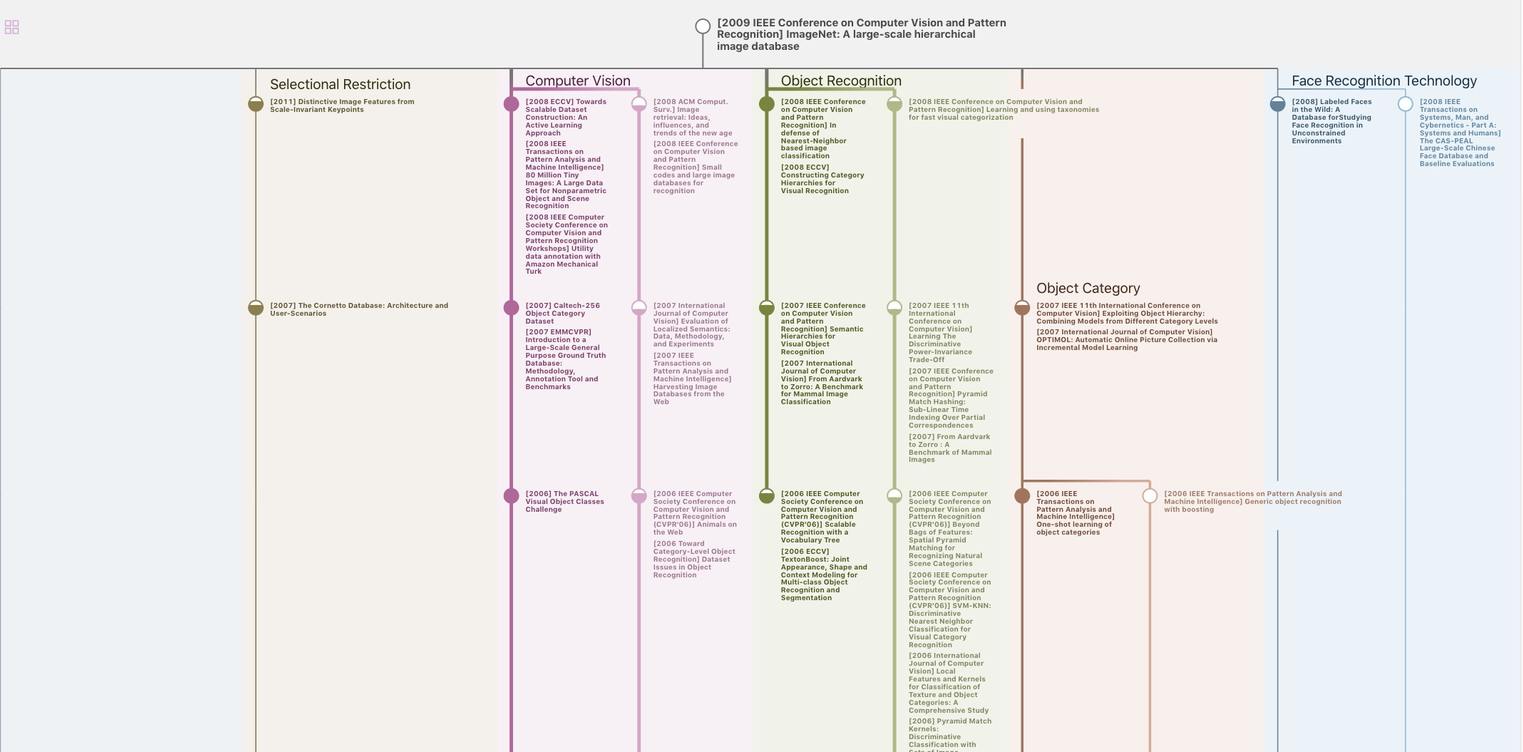
生成溯源树,研究论文发展脉络
Chat Paper
正在生成论文摘要