Motion Sickness Prediction in Stereoscopic Videos using 3D Convolutional Neural Networks.
IEEE transactions on visualization and computer graphics(2019)
摘要
In this paper, we propose a three-dimensional (3D) convolutional neural network (CNN)-based method for predicting the degree of motion sickness induced by a 360° stereoscopic video. We consider the user's eye movement as a new feature, in addition to the motion velocity and depth features of a video used in previous work. For this purpose, we use saliency, optical flow, and disparity maps of an input video, which represent eye movement, velocity, and depth, respectively, as the input of the 3D CNN. To train our machine-learning model, we extend the dataset established in the previous work using two data augmentation techniques: frame shifting and pixel shifting. Consequently, our model can predict the degree of motion sickness more precisely than the previous method, and the results have a more similar correlation to the distribution of ground-truth sickness.
更多查看译文
关键词
Videos,Stereo image processing,Three-dimensional displays,Optical imaging,Machine learning,Optical saturation,Optical computing
AI 理解论文
溯源树
样例
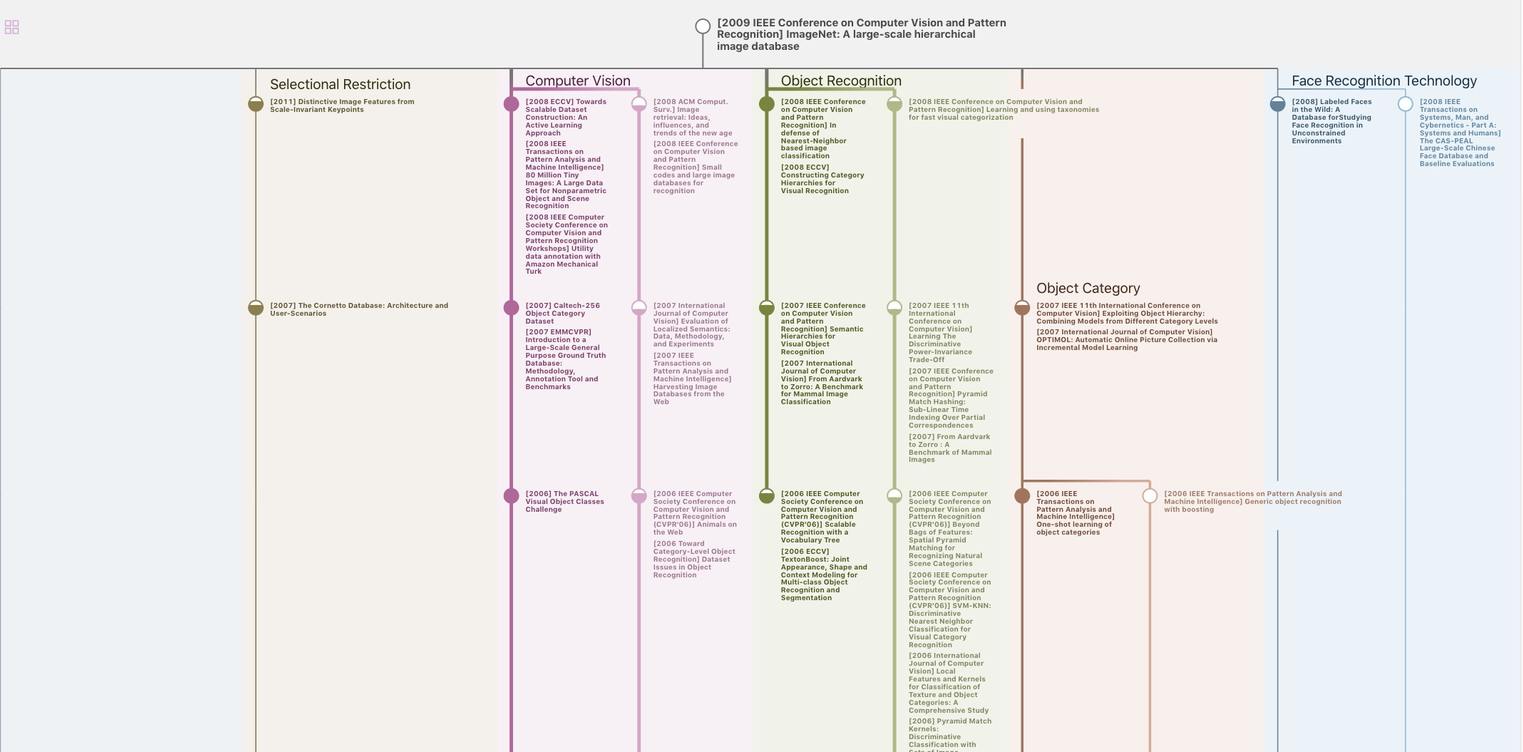
生成溯源树,研究论文发展脉络
Chat Paper
正在生成论文摘要