THE FINITE SAMPLE PERFORMANCE OF DYNAMIC MODE DECOMPOSITION
2018 IEEE Global Conference on Signal and Information Processing (GlobalSIP)(2018)
摘要
We analyze the Dynamic Mode Decomposition (DMD) algorithm as applied to multivariate time-series data. Our analysis reveals the critical role played by the lag-one cross-correlation, or cross-covariance, terms. We show that when the rows of the multivariate time series matrix can be modeled as linear combinations of lag-one uncorrelated latent time series that have a non-zero lag-one autocorrelation, then in the large sample limit, DMD perfectly recovers, up to a column-wise scaling, the mixing matrix, and thus the latent time series. We validate our findings with numerical simulations, and demonstrate how DMD can be used to unmix mixed audio signals.
更多查看译文
关键词
Multivariate Time Series,DMD
AI 理解论文
溯源树
样例
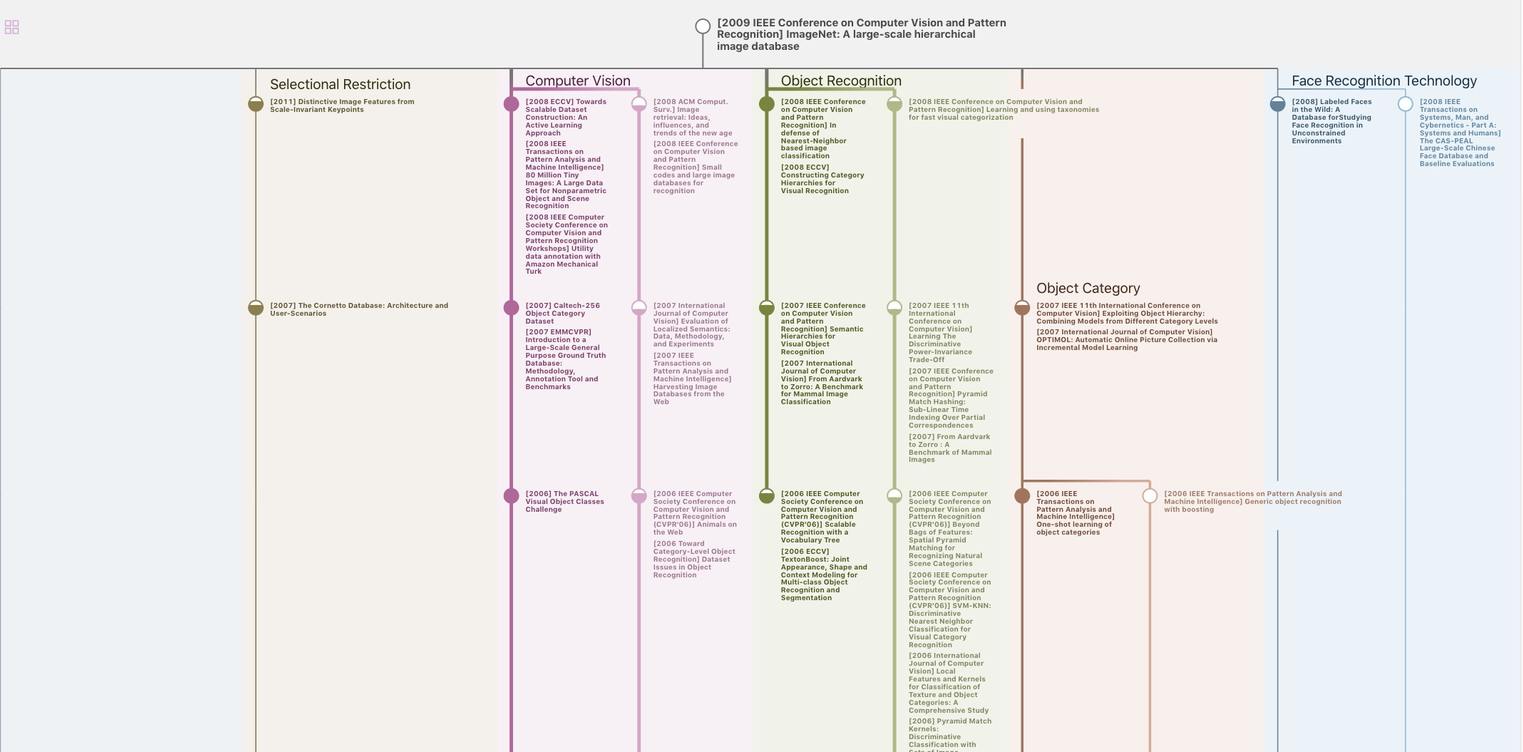
生成溯源树,研究论文发展脉络
Chat Paper
正在生成论文摘要