Efficiency Optimization of Deep Workout Recognition with Accelerometer Sensor for a Mobile Environment
TENCON 2018 - 2018 IEEE Region 10 Conference(2018)
摘要
Recent advancements in deep learning have created numerous possibilities for real-world application, among which the recognition of human motions with sensors. We employed a convolutional neural network (CNN) to process workout motion data to solve the segmentation and recognition problem. We focused on deploying the network architecture in a mobile environment characterized by limited resources. Our experimental results were promising in terms of both segmentation and recognition. Furthermore, we analyzed the performance correlation between sampling the rate and recognition rate. This result indicated 55Hz to provide an appropriate amount of information for workout motion. Then we investigated the computational cost and memory space usage of the hyper-parameter selection. The experimental results implied that appropriate hyper-parameter selection could reduce the computational burden on the mobile environment. Subsequently, we suggest an efficiency score regarding the computational cost, memory usage, and the recognition rate. Our suggested efficiency score shows that our method could find hyper-parameters that offer minimum loss of accuracy and are computationally inexpensive with optimal utilization of memory space.
更多查看译文
关键词
Motion segmentation,Presses,Tracking,Legged locomotion,Deep learning,Computational efficiency,Training
AI 理解论文
溯源树
样例
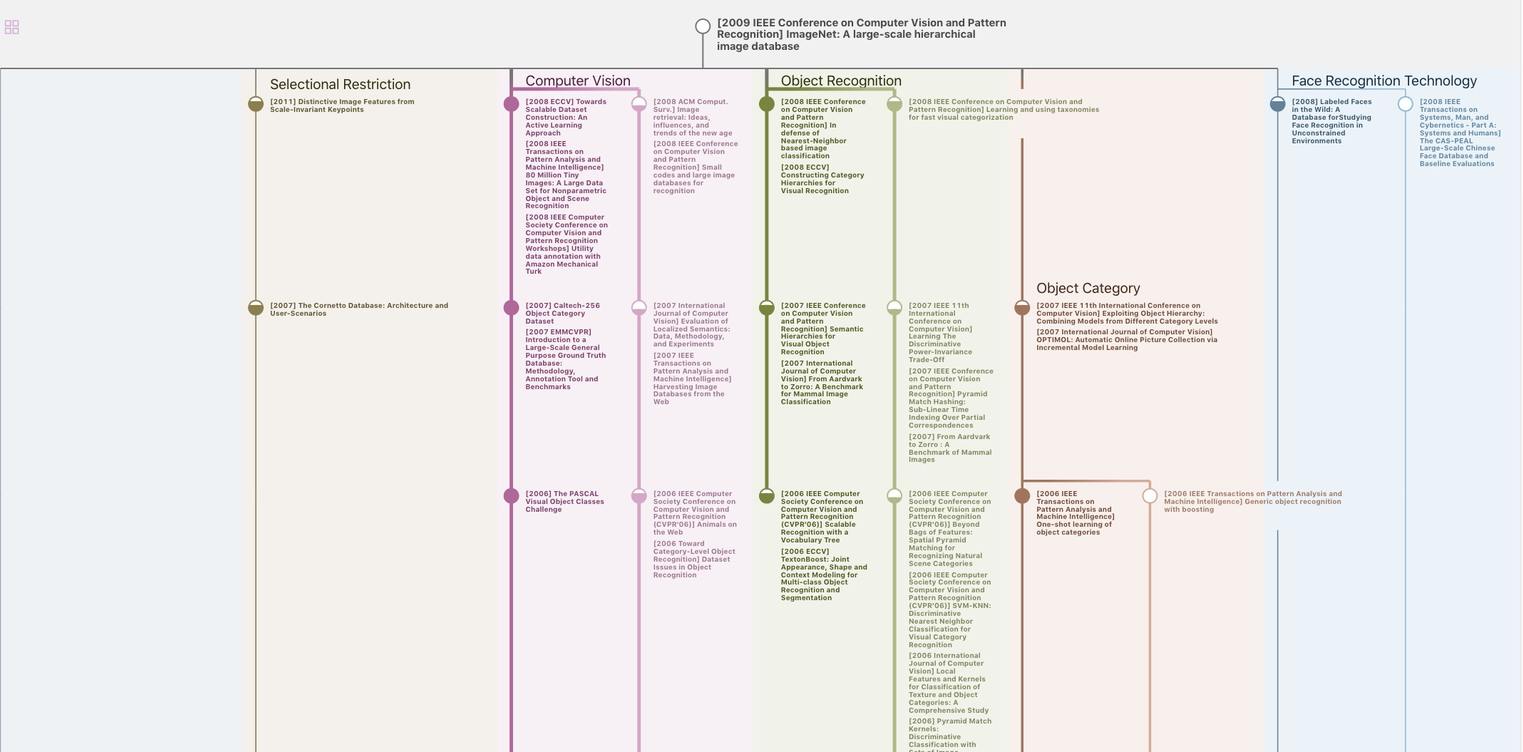
生成溯源树,研究论文发展脉络
Chat Paper
正在生成论文摘要