Beyond Photometric Loss for Self-Supervised Ego-Motion Estimation
2019 INTERNATIONAL CONFERENCE ON ROBOTICS AND AUTOMATION (ICRA)(2019)
摘要
Accurate relative pose is one of the key components in visual odometry (VO) and simultaneous localization and mapping (SLAM). Recently, the self-supervised learning framework that jointly optimizes the relative pose and target image depth has attracted the attention of the community. Previous works rely on the photometric error generated from depths and poses between adjacent frames, which contains large systematic error under realistic scenes due to reflective surfaces and occlusions. In this paper, we bridge the gap between geometric loss and photometric loss by introducing the matching loss constrained by epipolar geometry in a self-supervised framework. Evaluated on the KITTI dataset, our method outperforms the state-of-the-art unsupervised ego-motion estimation methods by a large margin.
更多查看译文
关键词
photometric loss,self-supervised ego-motion estimation,accurate relative pose,SLAM,self-supervised learning framework,image depth,photometric error,systematic error,realistic scenes,geometric loss,matching loss,self-supervised framework,unsupervised egomotion estimation methods
AI 理解论文
溯源树
样例
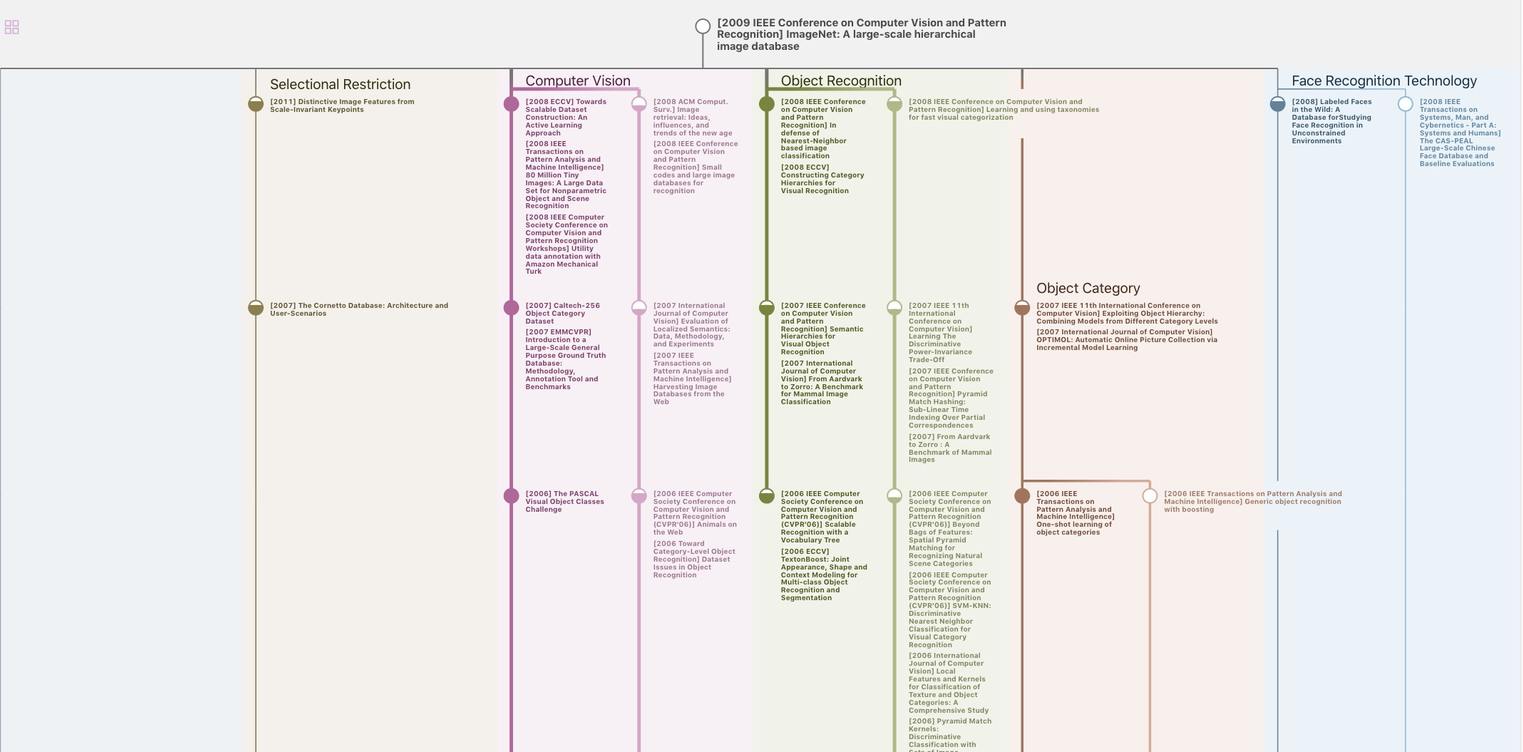
生成溯源树,研究论文发展脉络
Chat Paper
正在生成论文摘要