Multi-Information Source Optimization with General Model Discrepancies
arXiv: Machine Learning(2016)
摘要
In the multi-information source optimization problem our goal is to optimize a complex design. However, we only have indirect access to the objective value of any design via information sources that are subject to model discrepancy, i.e. whose internal model inherently deviates from reality. We present a novel algorithm that is based on a rigorous mathematical treatment of the uncertainties arising from the model discrepancies. Its optimization decisions rely on a stringent value of information analysis that trades off the predicted benefit and its cost. We conduct an experimental evaluation that demonstrates that the method consistently outperforms other state-of-the-art techniques: it finds designs of considerably higher objective value and additionally inflicts less cost in the exploration process.
更多查看译文
AI 理解论文
溯源树
样例
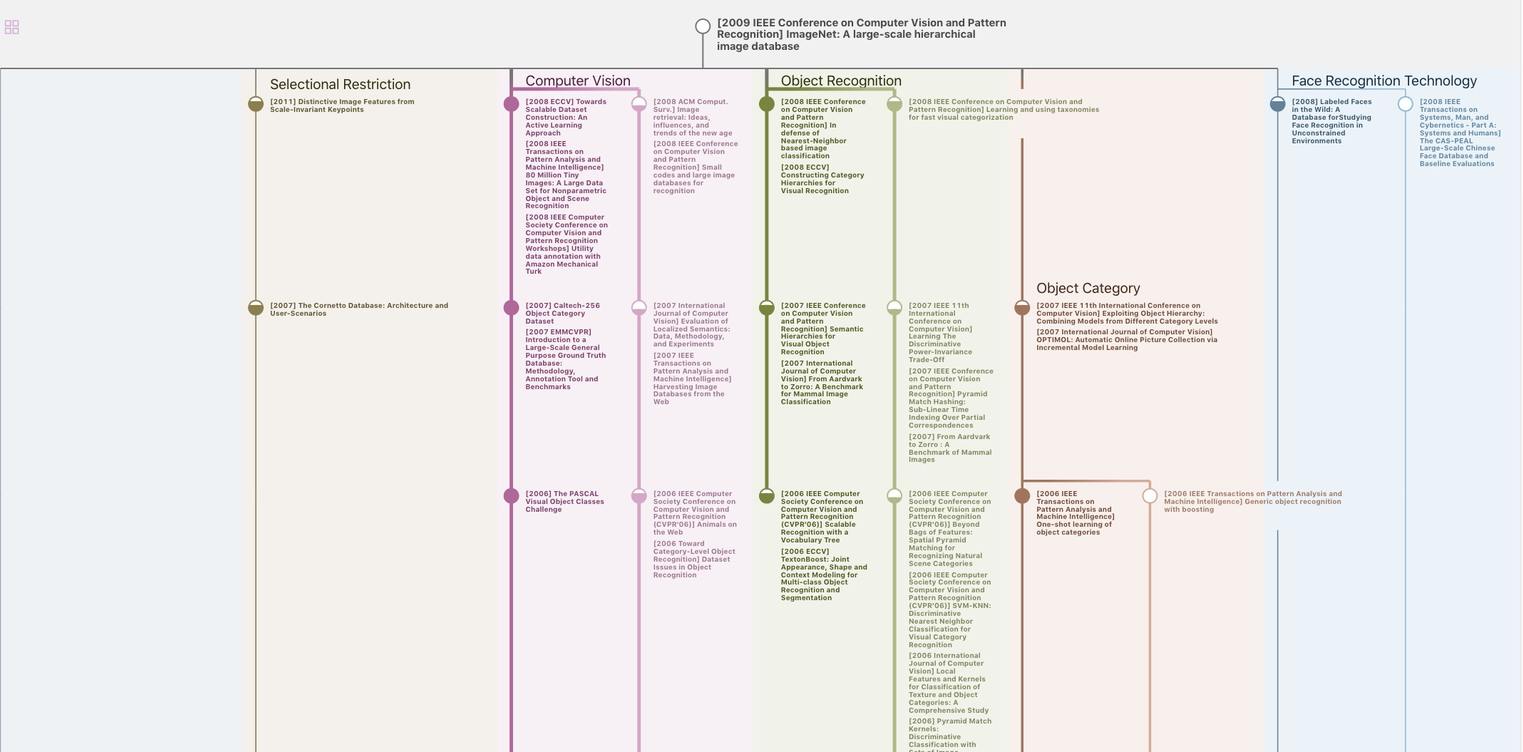
生成溯源树,研究论文发展脉络
Chat Paper
正在生成论文摘要