Mending the big-data missing information
2016 IEEE International Conference on the Science of Electrical Engineering (ICSEE)(2016)
摘要
Consider a high-dimensional data set, in which for every data-point there is incomplete information. Each object in the data set represents a real entity, which is described by a point in high-dimensional space. We model the lack of information for a given object as an affine subspace in R
d
whose dimension k is the number of missing features. Our goal in this study is to find clusters of objects where the main problem is to cope with partial information and high dimension. Assuming the data set is separable, namely, its emergence from clusters that can be modeled as a set of disjoint ball in R
d
, we develop a simple data clustering algorithm. Our suggested algorithm use the affine subspaces minimum distance and calculates pair-wise projection of the data achieving poly-logarithmic time complexity. We use probabilistic considerations to prove the algorithm's correctness. These probabilistic results are of independent interest, and can serve to better understand the geometry of high dimensional objects.
更多查看译文
关键词
data clustering,poly-logarithmic time complexity,probabilistic considerations,high dimensional objects,high-dimensional data set,Big-Data missing information
AI 理解论文
溯源树
样例
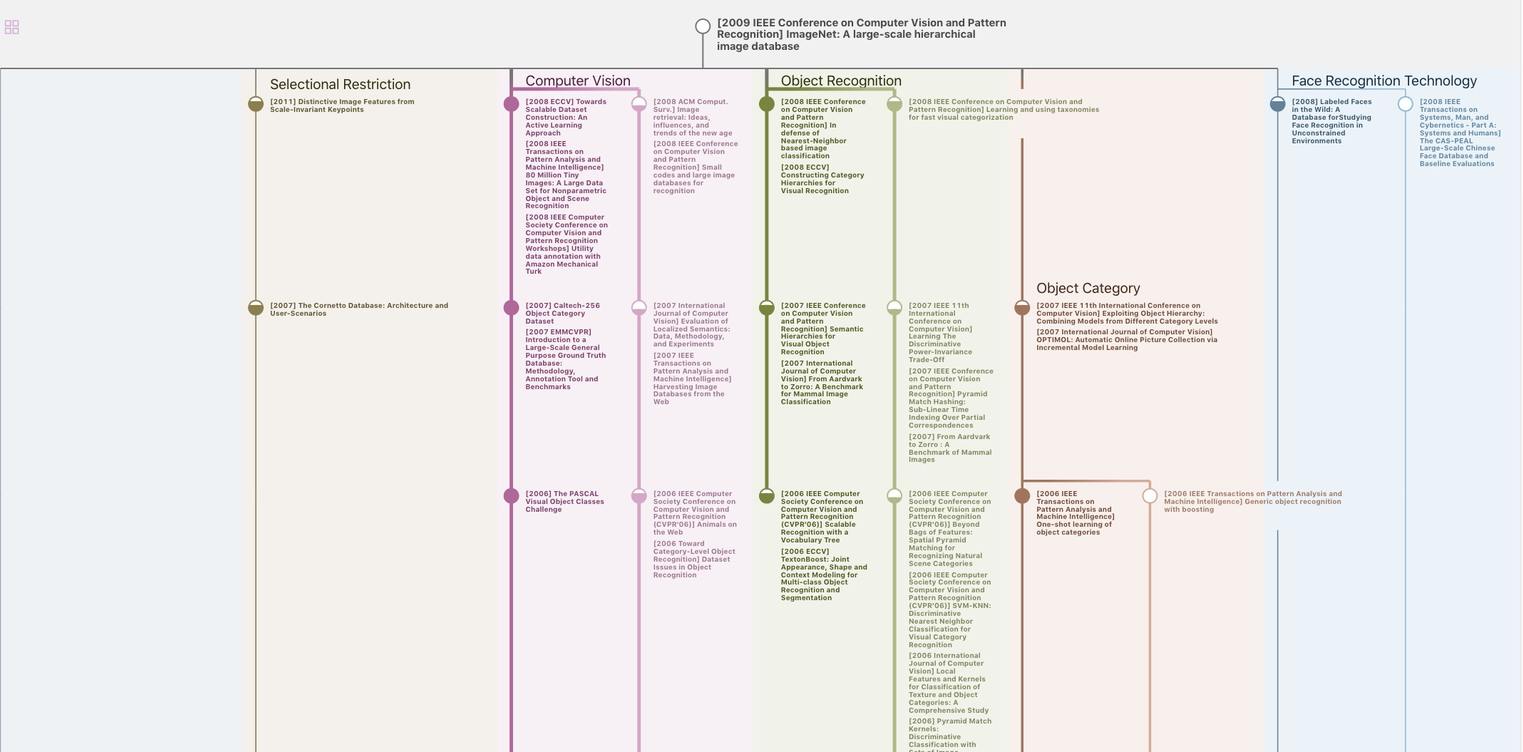
生成溯源树,研究论文发展脉络
Chat Paper
正在生成论文摘要