Two-sample testing in high dimensions
JOURNAL OF THE ROYAL STATISTICAL SOCIETY SERIES B-STATISTICAL METHODOLOGY(2017)
摘要
We propose new methodology for two-sample testing in high dimensional models. The methodology provides a high dimensional analogue to the classical likelihood ratio test and is applicable to essentially any model class where sparse estimation is feasible. Sparse structure is used in the construction of the test statistic. In the general case, testing then involves non-nested model comparison, and we provide asymptotic results for the high dimensional setting. We put forward computationally efficient procedures based on data splitting, including a variant of the permutation test that exploits sparse structure. We illustrate the general approach in two-sample comparisons of high dimensional regression models (differential regression') and graphical models (differential network'), showing results on simulated data as well as data from two recent cancer studies.
更多查看译文
关键词
Differential network,Differential regression,Gaussian graphical models,High dimensional regression,High dimensional two-sample testing,Non-nested hypotheses,Restricted log-likelihood-ratio statistic,Sparsity
AI 理解论文
溯源树
样例
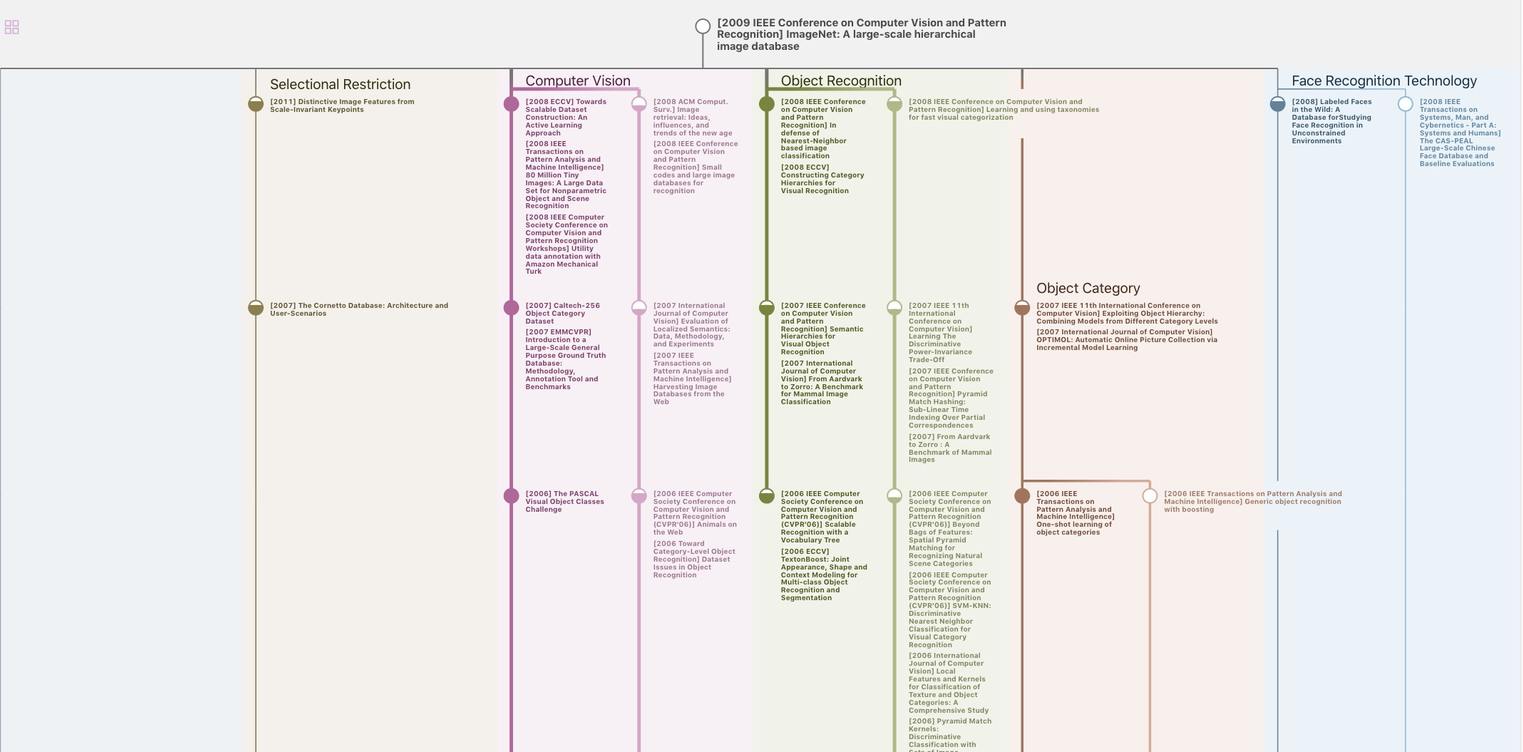
生成溯源树,研究论文发展脉络
Chat Paper
正在生成论文摘要