Segmental Spatio-Temporal CNNs for Fine-grained Action Segmentation and Classification
arXiv: Computer Vision and Pattern Recognition(2016)
摘要
Joint segmentation and classification of fine-grained actions is important for applications in human-robot interaction, video surveillance, and human skill evaluation. However, despite substantial recent progress in large scale action classification, the performance of state-of-the-art fine-grained action recognition approaches remains low. In this paper, we propose a new spatio-temporal CNN model for fine-grained action classification and segmentation, which combines (1) a spatial CNN to represent objects in the scene and their spatial relationships; (2) a temporal CNN that captures how object relationships within an action change over time; and (3) a semi-Markov model that captures transitions from one action to another. In addition, we introduce an efficient segmental inference algorithm for joint segmentation and classification of actions that is orders of magnitude faster than state-of-the-art approaches. We highlight the effectiveness of our approach on cooking and surgical action datasets for which we observe substantially improved performance relative to recent baseline methods.
更多查看译文
AI 理解论文
溯源树
样例
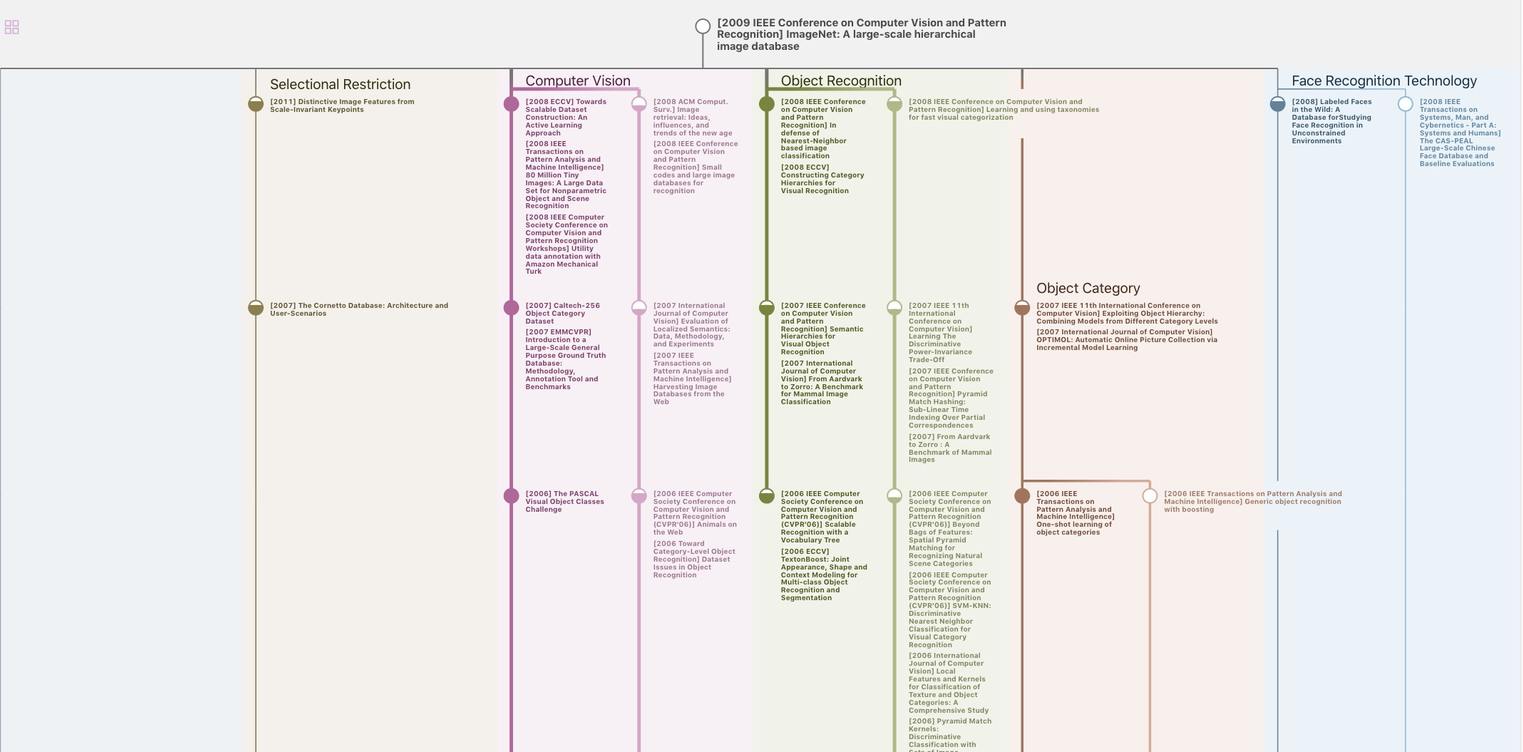
生成溯源树,研究论文发展脉络
Chat Paper
正在生成论文摘要