NIMG-59PREDICTING THE RESPONSE TO TREATMENT IN GBM: MACHINE LEARNING ON CLINICAL IMAGES
Neuro-oncology(2015)
摘要
NIMG-59. PREDICTING THE RESPONSE TO TREATMENT IN GBM: MACHINE LEARNING ON CLINICAL IMAGES Corbin Rayfield and Kristin Swanson; Mayo Clinic, Scottsdale, AZ, USA Patients suffering from glioblastoma exhibit a wide response to therapy. Studies relying on genetic/phenotypic markers to predict treatment response struggle to deliver clinically useful information in an actionable timeframe which underscores the need for a predictive measure produced by pretreatment clinical information. Our group has previously described the Days Gained (DG) metric of response which is capable of stratifying patients by overall and progression free survival (Neal 2013). We sought to explore whether a predictive algorithm could be used to predict patients’ response to therapy as measured by the DG metric. We studied 52 patients with newly diagnosed glioblastoma who had at least 2 pre-treatment and 1 post radiation therapy MRIs. The group was divided into a training (n 1⁄4 31) and a test set (n 1⁄4 21). A decision tree calculated through multiple iterations of linear regressions was used to generate variables that were correlated with the DG score and cluster the training patients according to clinically available variables. A random forest algorithm was used to search for the optimal model that would differentiate those patients who had a significant response to treatment (DG . 73), previously shown to be predictive of better overall survival (Neal 2013). The variables that clustered patients by treatment response were age, gender, Karnofsky Performance Score, tumors size on MRI (T1Gd and T2) at diagnosis, extent of resection, pre-treatment velocity, and the ratio of the net rates of diffusion to proliferation. A test performed on the test cohort showed 95% accuracy in predicting their response to treatment. This tool, utilizing nothing more than information available at the time of surgery, patients can be stratified according to expected response to adjuvant therapy. By targeting those patients that are predicted not to respond, clinicians can begin to consider more aggressive radiation schemes, faster implementation of salvage therapy or clinical trials. Neuro-Oncology 17:v153–v171, 2015. doi:10.1093/neuonc/nov225.59 Published by Oxford University Press on behalf of the Society for Neuro-Oncology 2015.
更多查看译文
AI 理解论文
溯源树
样例
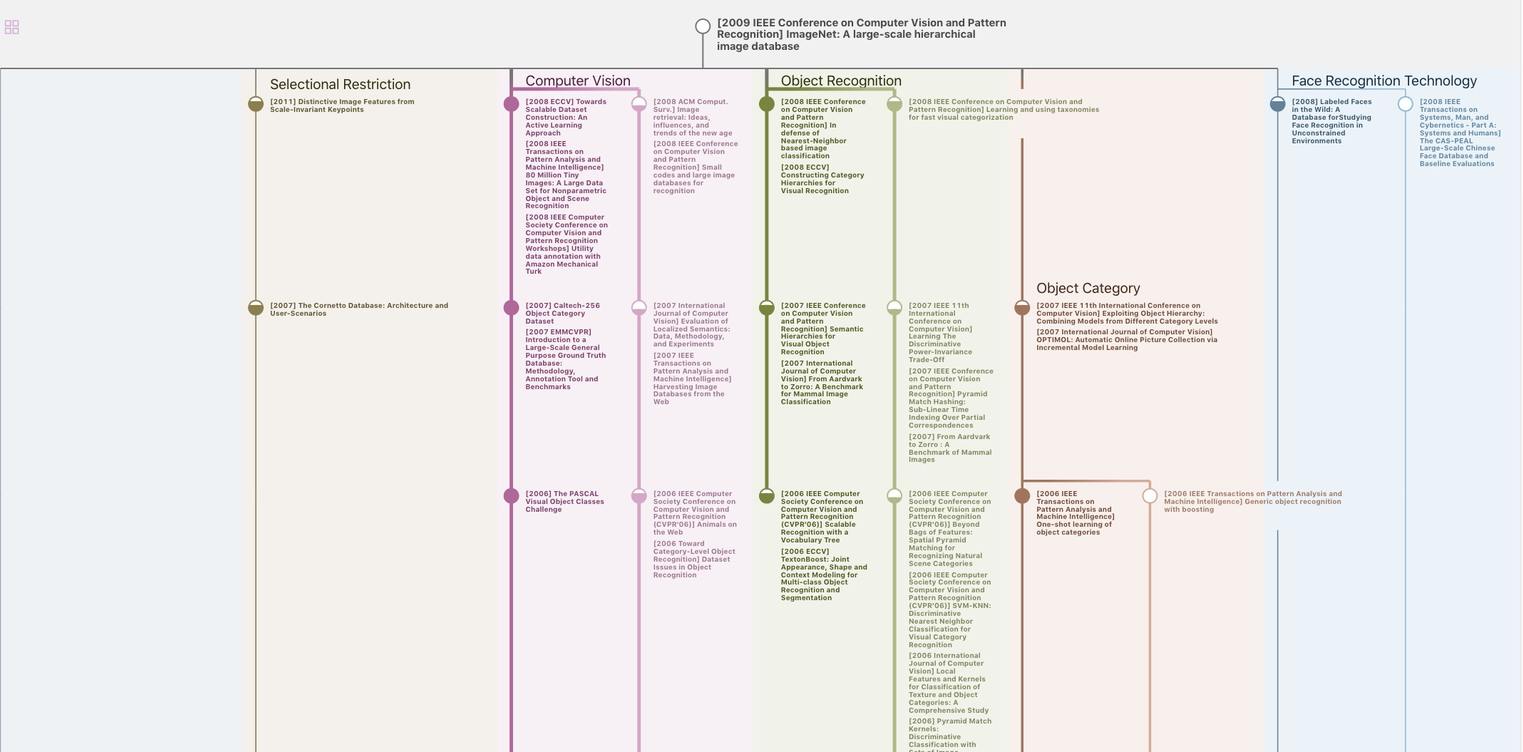
生成溯源树,研究论文发展脉络
Chat Paper
正在生成论文摘要