Principal Component Analysis Vs. Self-Organizing Maps Combined with Hierarchical Clustering for Pattern Recognition in Volcano Seismic Spectra
Journal of volcanology and geothermal research(2016)
摘要
Variations in the spectral content of volcano seismicity related to changes in volcanic activity are commonly identified manually in spectrograms. However, long time series of monitoring data at volcano observatories require tools to facilitate automated and rapid processing. Techniques such as self-organizing maps (SOM) and principal component analysis (PCA) can help to quickly and automatically identify important patterns related to impending eruptions. For the first time, we evaluate the performance of SOM and PCA on synthetic volcano seismic spectra constructed from observations during two well-studied eruptions at Klauea Volcano, Hawai'i, that include features observed in many volcanic settings. In particular, our objective is to test which of the techniques can best retrieve a set of three spectral patterns that we used to compose a synthetic spectrogram. We find that, without a priori knowledge of the given set of patterns, neither SOM nor PCA can directly recover the spectra. We thus test hierarchical clustering, a commonly used method, to investigate whether clustering in the space of the principal components and on the SOM, respectively, can retrieve the known patterns. Our clustering method applied to the SOM fails to detect the correct number and shape of the known input spectra. In contrast, clustering of the data reconstructed by the first three PCA modes reproduces these patterns and their occurrence in time more consistently. This result suggests that PCA in combination with hierarchical clustering is a powerful practical tool for automated identification of characteristic patterns in volcano seismic spectra. Our results indicate that, in contrast to PCA, common clustering algorithms may not be ideal to group patterns on the SOM and that it is crucial to evaluate the performance of these tools on a control dataset prior to their application to real data.
更多查看译文
关键词
Volcano seismicity,Pattern recognition,Hierarchical clustering,Principal component analysis,Self-organizing maps
AI 理解论文
溯源树
样例
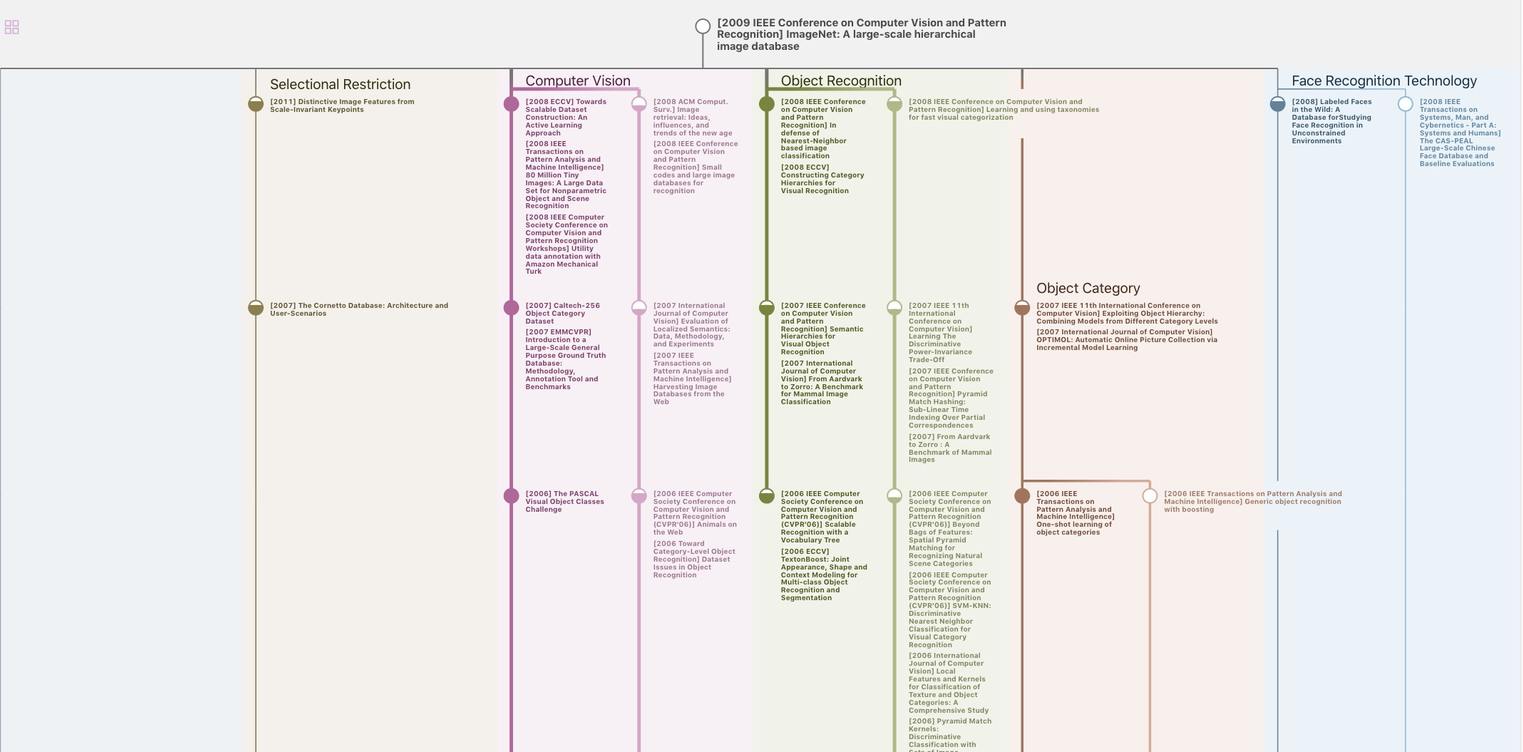
生成溯源树,研究论文发展脉络
Chat Paper
正在生成论文摘要