EM and component-wise boosting for Hidden Markov Models: a machine-learning approach to capture-recapture
bioRxiv(2017)
摘要
This study presents a new boosting method for capture-recapture models, rooted in predictive-performance and machine-learning. The regularization algorithm combines Expectation-Maximization and boosting to yield a type of multimodel inference, including automatic variable selection and control of model complexity. By analyzing simulations and a real dataset, this study shows the qualitatively similar estimates between AICc model-averaging and boosted capture-recapture for the CJS model. I discuss a number of benefits of boosting for capture-recapture, including: i) ability to fit non-linear patterns (regression-trees, splines); ii) sparser, simpler models that are less prone to over-fitting, singularities or boundary-value estimates than conventional methods; iii) an inference paradigm that is rooted in predictive-performance and free of p-values or 95% confidence intervals; and v) estimates that are slightly biased, but are more stable over multiple realizations of the data. Finally, I discuss some philosophical considerations to help practitioners motivate the use of either prediction-optimal methods (AIC, boosting) or model-consistent methods. The boosted capture-recapture framework is highly extensible and could provide a rich, unified framework for addressing many topics in capture-recapture, such as spatial capture-recapture, individual heterogeneity, and non-linear effects.
更多查看译文
关键词
capture-recapture,boosting,machine-learning,model-selection,marked animals,high-dimensional data
AI 理解论文
溯源树
样例
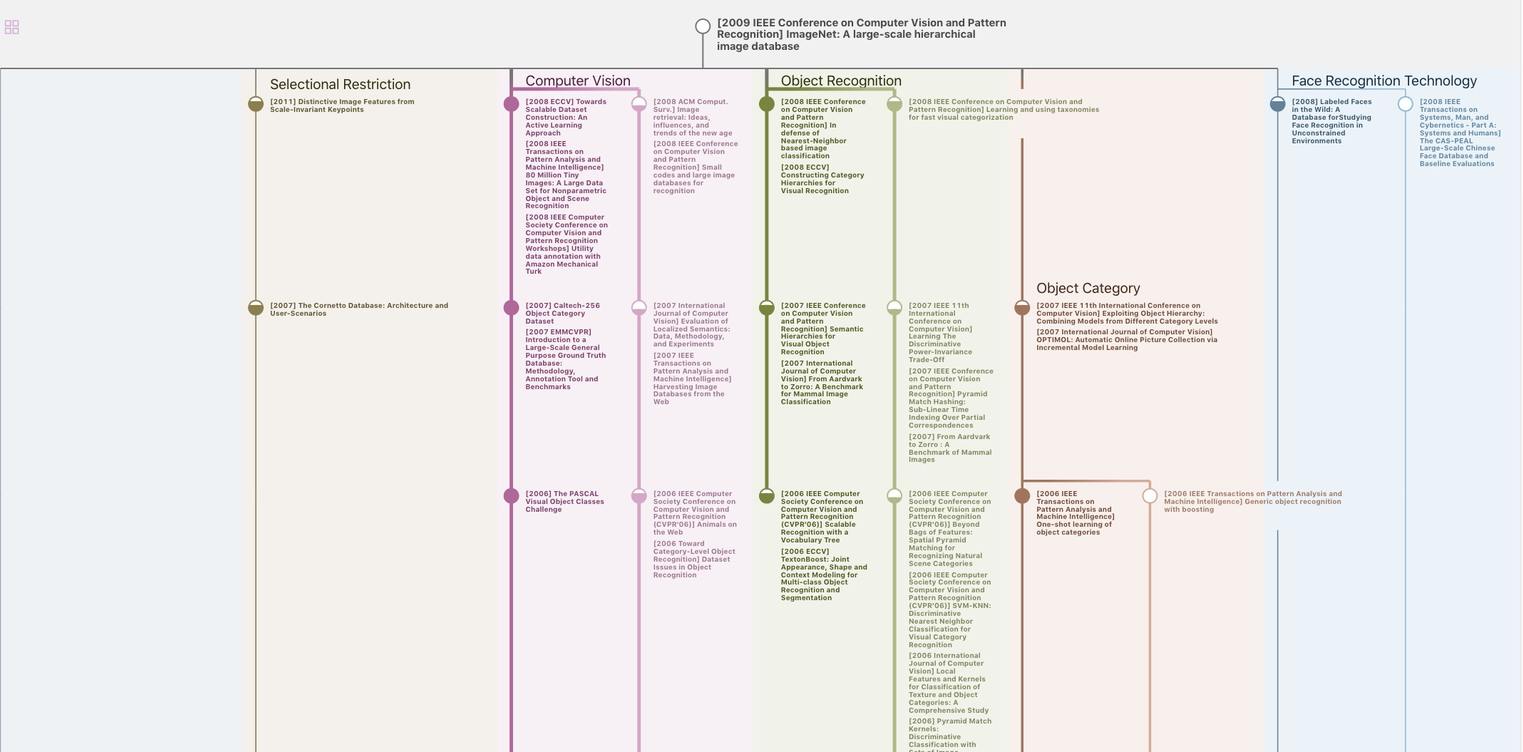
生成溯源树,研究论文发展脉络
Chat Paper
正在生成论文摘要