Wear Rate Prediction Of Grinding Media Using Bpnn And Mlr Models In Grinding Of Sulphide Ores
IRANIAN JOURNAL OF MATERIALS SCIENCE AND ENGINEERING(2016)
摘要
Nowadays steel halls wear is a major problem in mineral processing industries and forms a significant part of the grinding cost. Different factors are effective on halls wear. It is needed to find models which are capable to estimate wear rate from these factors. In this paper a hack propagation neural network (BPNN) and multiple linear regression (MLR) method have been used to predict wear rate of steel halls using some significant parameters including, pH, solid content, throughout of grinding circuit, speed of mill, charge weight of halls and grinding time. The comparison between the predicted wear rates and the measured data resulted in the correlation coefficients (R), 0.977 and 0.955 for training and test data using BPNN model. However, the R values were 0.936 and 0.969 for training and test data by MLR method. In addition, the average absolute percent relative error (RAPE) obtained 2.79 and 4.18 for train and test data in BPNN model, respectively. Finally, Analysis of the predictions shows that the BPNN and MLR methods could he used with good engineering accuracy to directly predict the wear rate of steel halls.
更多查看译文
关键词
Wear rate, Steel halls, Grinding, Neural Network, Multiple Linear Regression
AI 理解论文
溯源树
样例
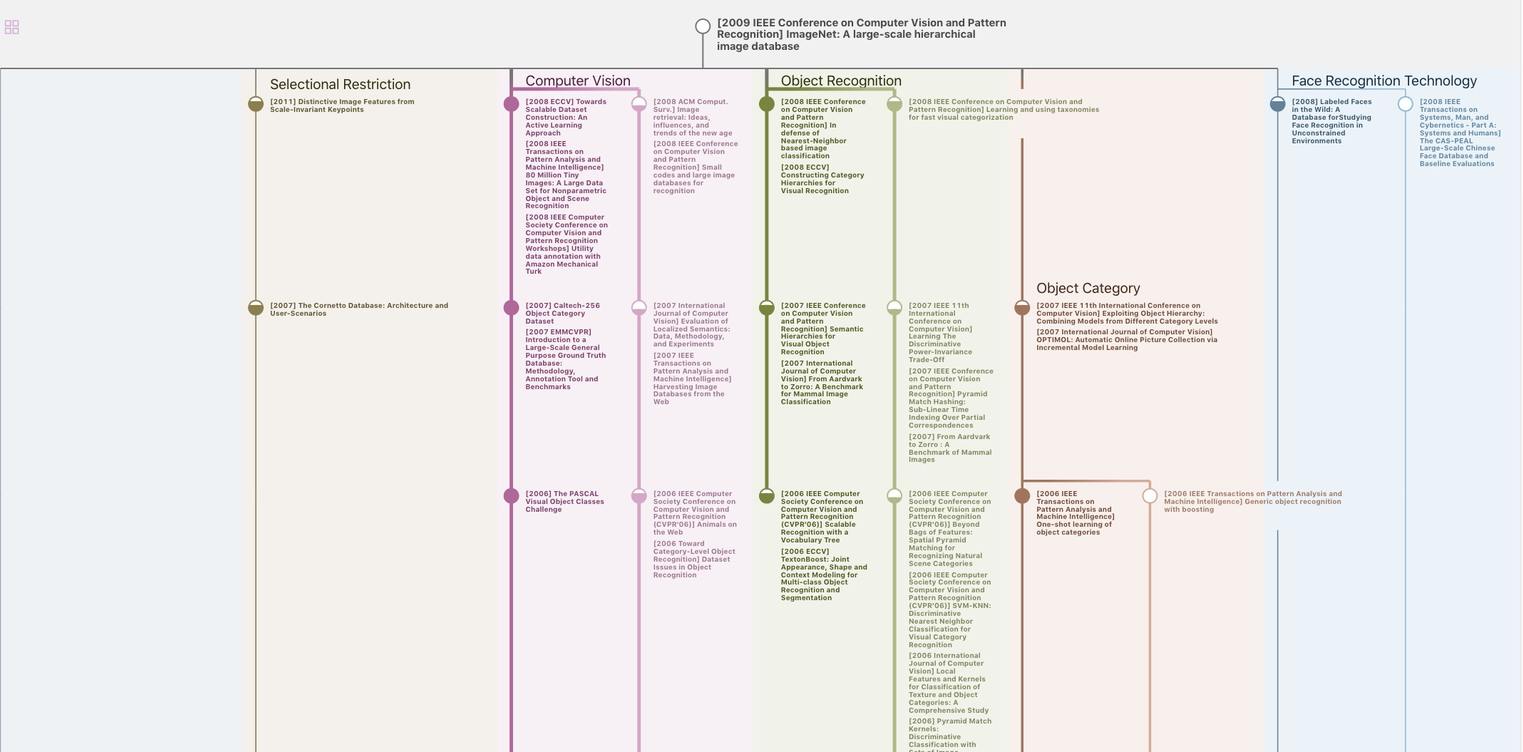
生成溯源树,研究论文发展脉络
Chat Paper
正在生成论文摘要