Provable Symmetric Nonnegative Matrix Factorization for Overlapping Clustering
arXiv: Machine Learning(2016)
摘要
The problem of finding overlapping communities in networks has gained much attention recently. Algorithmic approaches often employ non-negative matrix factorization (NMF) or variants, while model-based approaches (such as the widely used mixed-membership stochastic blockmodel, or MMSB) assume a distribution over communities for each node and run standard inference techniques to recover these parameters. However, few of these approaches have provable consistency guarantees. We investigate the use of the symmetric NMF (or SNMF) for the MMSB model, and provide conditions under which an optimal SNMF algorithm can recover the MMSB parameters consistently. Since we are unaware of general-purpose optimal SNMF algorithms, we develop an SNMF variant, called GeoNMF, designed specifically for the MMSB model. GeoNMF is provably consistent, and experiments on both simulated and real-world datasets show its accuracy.
更多查看译文
AI 理解论文
溯源树
样例
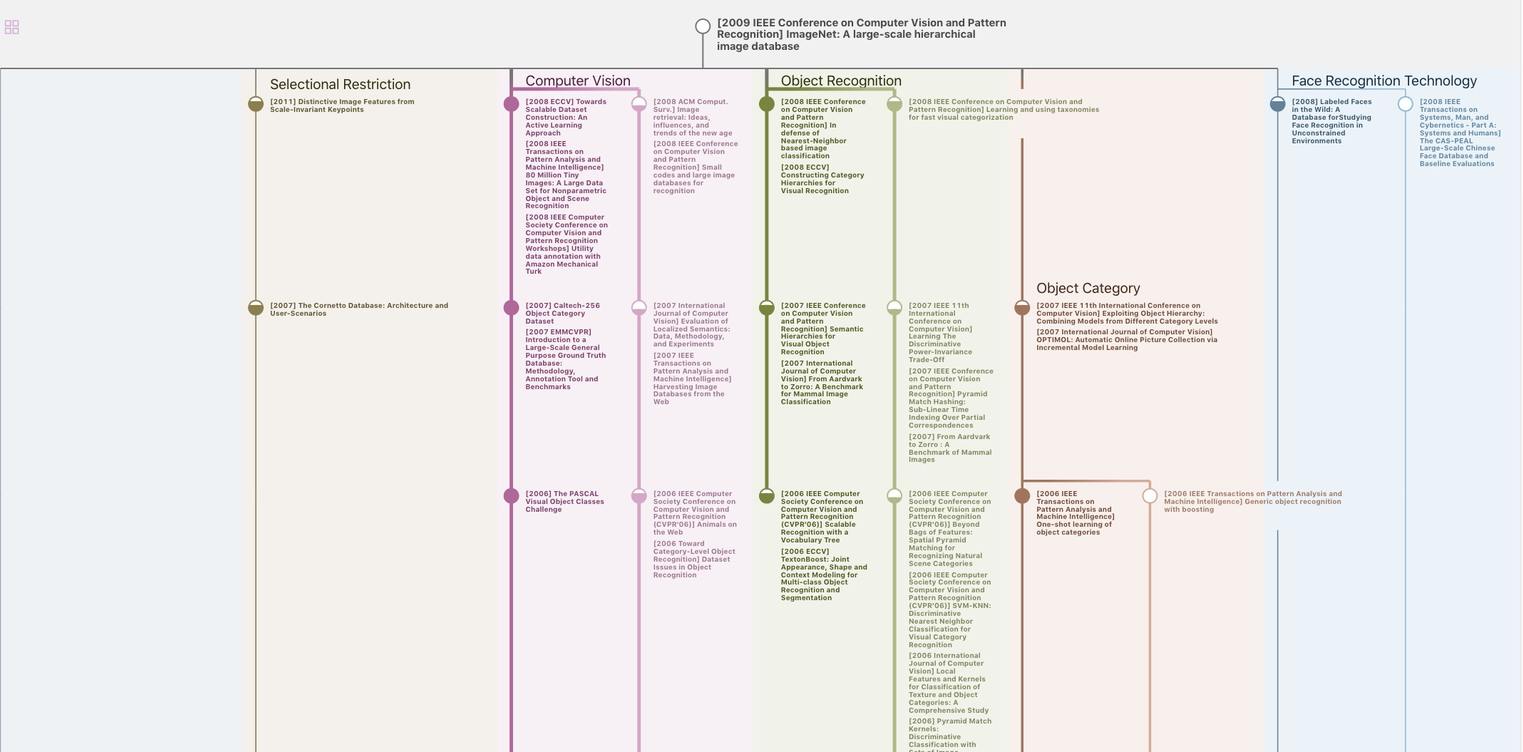
生成溯源树,研究论文发展脉络
Chat Paper
正在生成论文摘要