Prediction of Resistivity Index by Use of Neural Networks With Different Combinations of Wireline Logs and Minimal Core Data (see associated supplementary discussion)
SPE RESERVOIR EVALUATION & ENGINEERING(2017)
摘要
The measurement of special-core-analysis (SCAL) parameters in the laboratory can be a very time-consuming and costly process. The purpose of the present study was to see whether neural networks could be applied to predict a SCAL parameter, such as resistivity index (RI), from minimal training data, because this approach could potentially be used in the future to save time and costs. Our study was undertaken in the Nubian sandstone formation of two Libyan oil fields (A-Libya and B-Libya). A series of different neural-network predictors, to predict the parameter RI, was trained in Well A-02 by use of laboratory measurements undertaken on SCAL plugs together with different combinations of associated wireline logs. The performance of the predictors was then tested in two test wells (Well A-01 in the same oil field as Well A-02, and Well B-01 in a different neighboring oil field). One set of neural-network predictors was trained on a data set containing all 55 SCAL plugs, along with the corresponding wire line -log data, from the Training Well A-02. This training data set is in itself relatively small compared with many previous neural net studies in the petroleum industry. The predictions were then compared with another set of genetically-focused-neural-net (GFNN) predictors trained on a much smaller subset of just 14 SCAL plugs, again with the corresponding wireline-log data, from a representative genetic unit (RGU) in Well A-02. The RGU contained representative core plugs from each global hydraulic element (GHE) in that well. Remarkably, the predictors trained on the smaller subset of 14 representative SCAL plugs gave slightly better predictions, compared with the measured values throughout the test wells, than the predictors trained on the larger data set comprising 55 SCAL plugs. For Test Well A-01, we obtained even better results when the GFNN predictors were tested only in the interval equivalent to the RGU of Training Well A-02. We subsequently also obtained very good results from GFNN predictors of the Amott-Harvey wettability index in Test Wells A-01 and B-01 when tested in the intervals equivalent to the RGU of Training Well A-02. This study demonstrates that the SCAL parameters investigated here can be reasonably well-predicted from neural nets, and that the training data set can be quite small if one carefully chooses SCAL plugs that are representative of the petrophysical properties in the reservoir. This approach has the potential of saving both time and costs, because it requires only minimal SCAL data, and would be particularly useful for wells where no core material is available.
更多查看译文
AI 理解论文
溯源树
样例
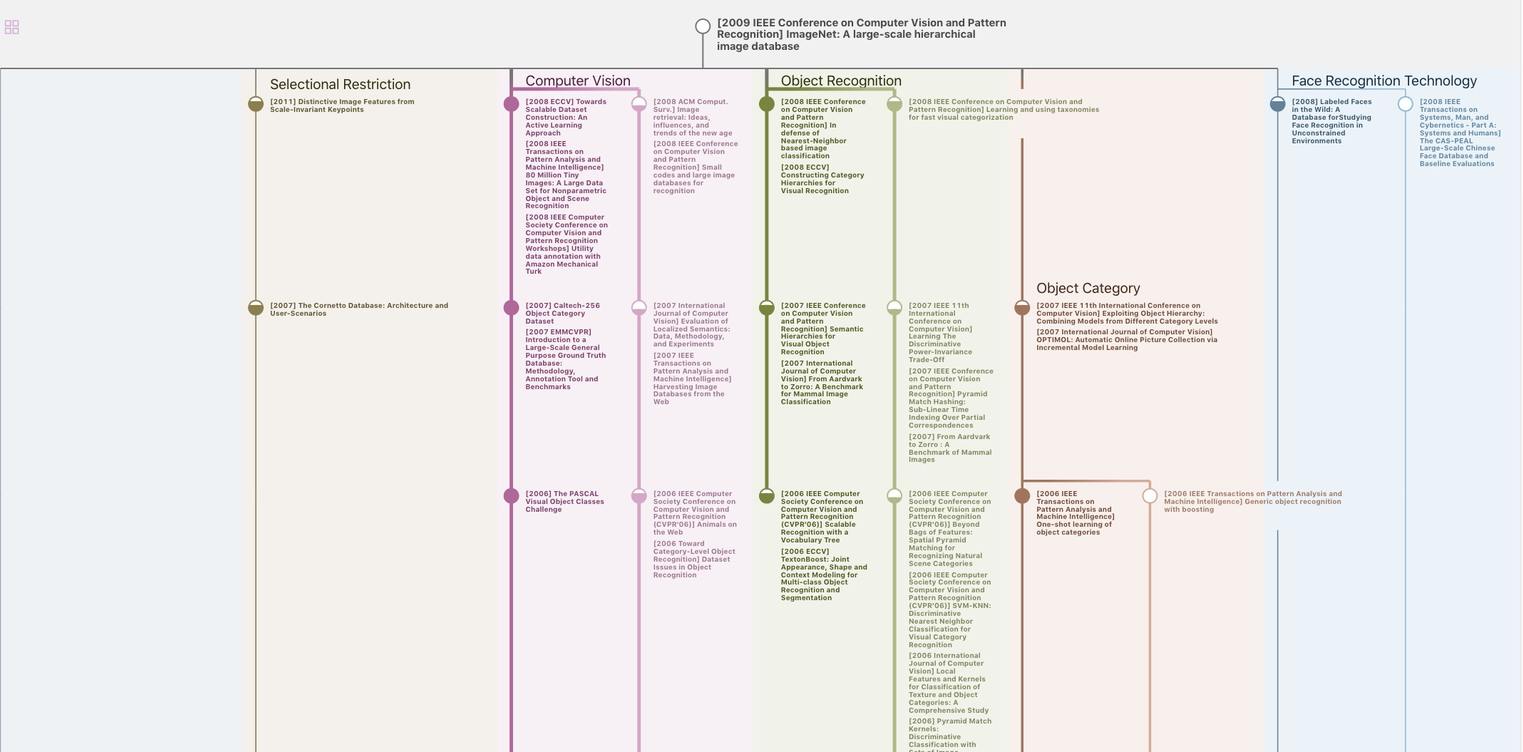
生成溯源树,研究论文发展脉络
Chat Paper
正在生成论文摘要