Ontology computation for graph spaces focus on partial vertex pairs
JOURNAL OF DIFFERENCE EQUATIONS AND APPLICATIONS(2017)
摘要
The essence of all kinds of ontology applications is similarity measuring. In recent years, a variety of learning approaches are introduced to ontology similarity computation and ontology mapping. The purpose of these ontology learning technologies is to get an ontology score function which maps each vertex to a real number, and then the similarity between ontology vertices are judged according to the difference of their scores. However, such learning data should containing all pairs of sample vertices. In this paper, we raise a new ontology learning algorithm in which its training sample set only contains important edges (vertex pair) of which the similarities are to be determined by us here. Our method is based on the Kronecker kernel technologies and the solution is attributed to solving the linear system. Two simulation experiments reveal that our new ontology learning model has higher precision ratio on plant ontology and humanoid robotics ontology for similarity measuring and ontology mapping applications.
更多查看译文
关键词
Ontology,similarity measure,ontology mapping,adjoint graph,Kronecker kernel
AI 理解论文
溯源树
样例
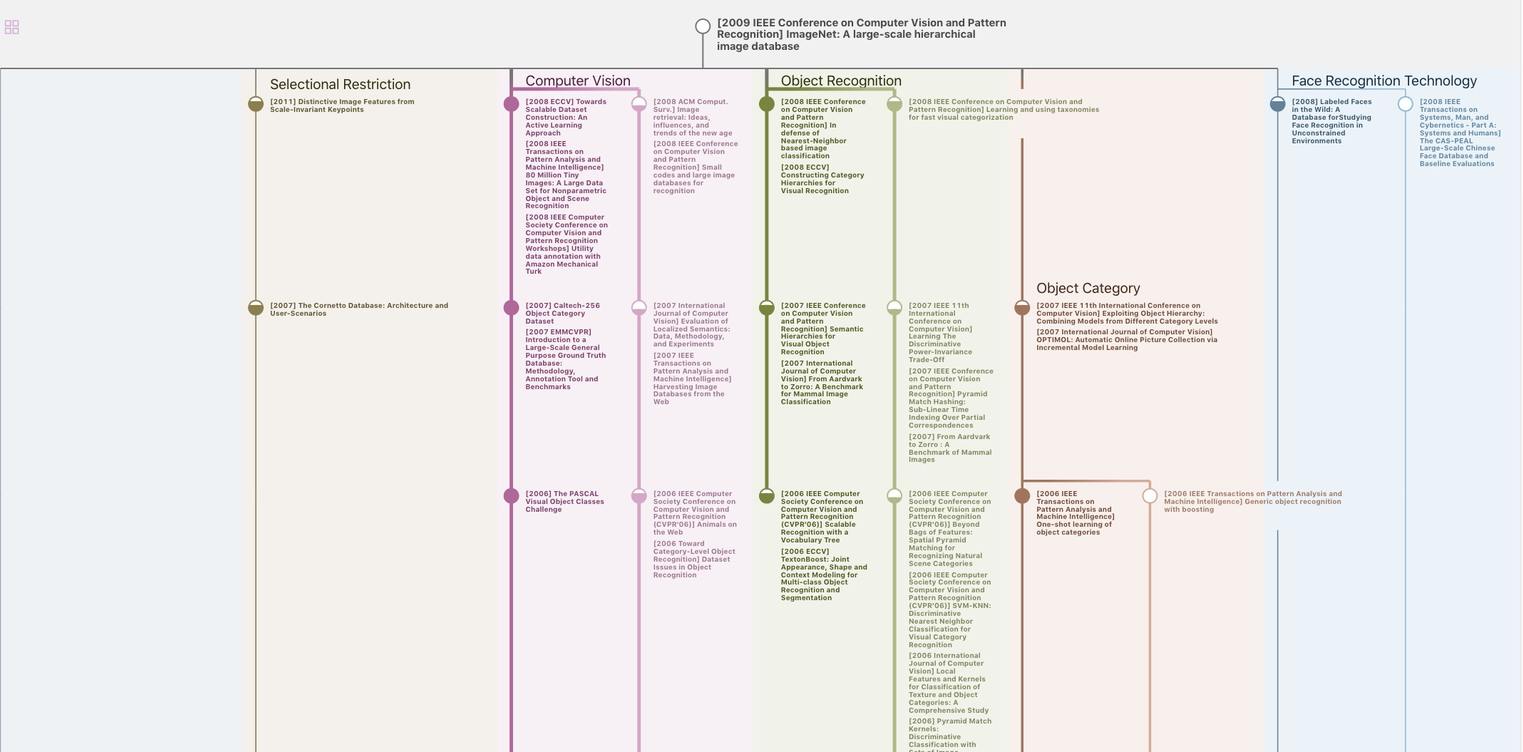
生成溯源树,研究论文发展脉络
Chat Paper
正在生成论文摘要