Multi-category Angle-based Classifier Refit
arXiv: Statistics Theory(2016)
摘要
Classification is an important statistical learning tool. In real application, besides high prediction accuracy, it is often desirable to estimate class conditional probabilities for new observations. For traditional problems where the number of observations is large, there exist many well developed approaches. Recently, high dimensional low sample size problems are becoming increasingly popular. Margin-based classifiers, such as logistic regression, are well established methods in the literature. On the other hand, in terms of probability estimation, it is known that for binary classifiers, the commonly used methods tend to under-estimate the norm of the classification function. This can lead to biased probability estimation. Remedy approaches have been proposed in the literature. However, for the simultaneous multicategory classification framework, much less work has been done. We fill the gap in this paper. In particular, we give theoretical insights on why heavy regularization terms are often needed in high dimensional applications, and how this can lead to bias in probability estimation. To overcome this difficulty, we propose a new refit strategy for multicategory angle-based classifiers. Our new method only adds a small computation cost to the problem, and is able to attain prediction accuracy that is as good as the regular margin-based classifiers. On the other hand, the improvement of probability estimation can be very significant. Numerical results suggest that the new refit approach is highly competitive.
更多查看译文
AI 理解论文
溯源树
样例
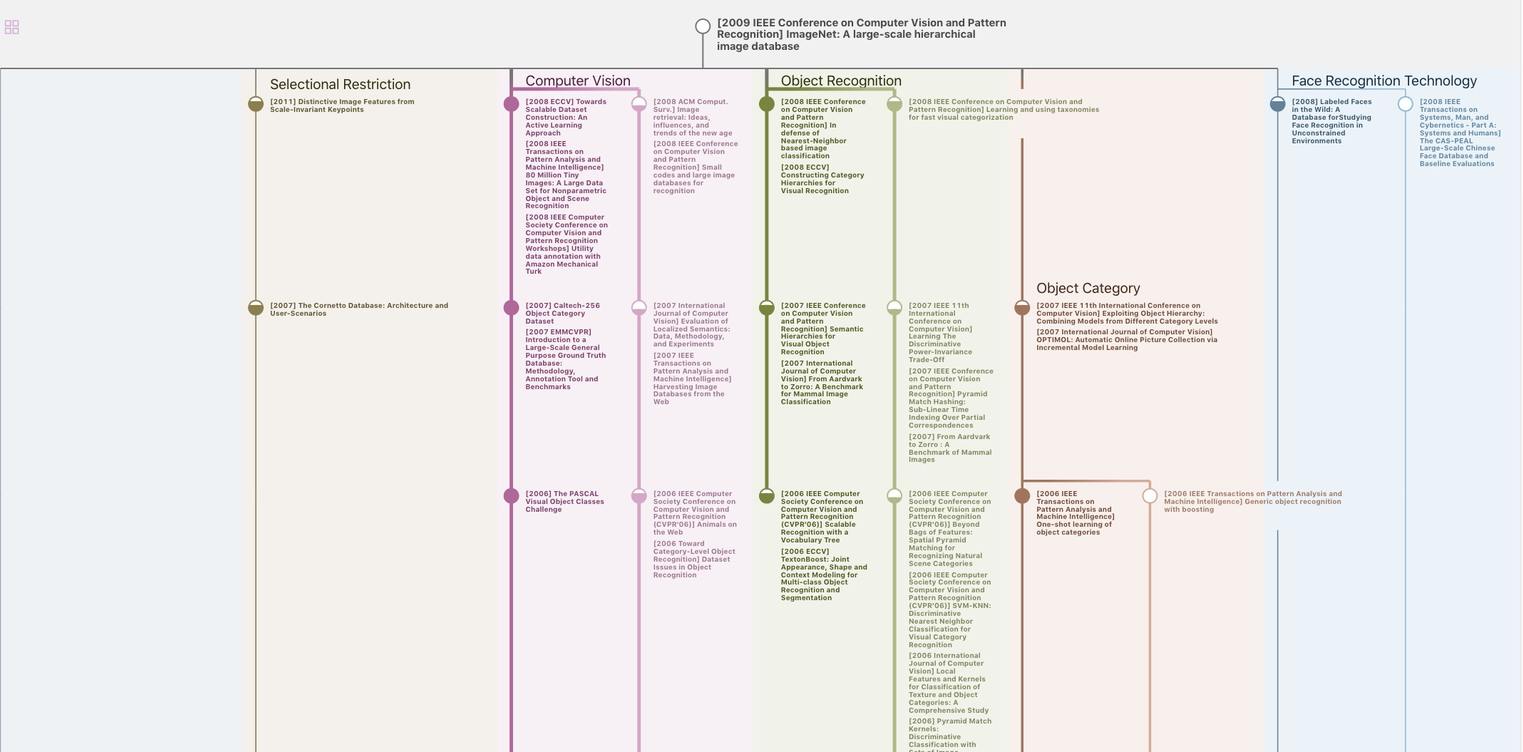
生成溯源树,研究论文发展脉络
Chat Paper
正在生成论文摘要