Improved Geometric Deconvolution Of Bulk Tumor Genomic Data
CANCER RESEARCH(2016)
摘要
We develop new methods for improved computational deconvolution of clonal populations from heterogeneous bulk genomic data. Deconvolution methods have become an important tool in resolving intratumor heterogeneity from bulk tumor genomic data, but prevailing methods are still able only to resolve only coarse-grained approximations of true clonal substructure. We seek to improve a genomic deconvolution strategy called “simplicial complex inference” that is designed to take advantage of similarity between the clonal subpopulations of single tumors or tumor regions to better infer portraits of those clonal subpopulations and their distribution across tumor samples. We evaluate the method through application to DNA copy-number (CN) data from a 472-tumor ovarian cancer (OV) panel and a 408-tumor lung squamous small cell carcinoma (LUSC) panel from The Cancer Genome Atlas (TCGA). We deconvolved the OV data into a mixture of six clonal models, two per data sample, each interpreted as a consensus copy number profile of one recurring progression stage found in a subset of OV samples. We analyzed the resulting models for enrichment of labels derived from RNASeq-based subtyping (Verhaak et al., 2013), revealing significant correspondence by chi-squared test between tumor subclusters identified by the deconvolution and OV subtypes (p = 0.0168). We further analyzed the clonal models to identify regions of significant amplification or loss, which we tested for enrichment of gene ontology (GO) terms and pathway terms through the DAVID toolkit. Ontological analysis showed significant enrichment for GO terms connected to genetic regulation and proliferation including “DNA binding,” “DNA packing,” and “protein-DNA complex” (p Citation Format: Theodore Roman, Lu Xie, Russell Schwartz. Improved geometric deconvolution of bulk tumor genomic data. [abstract]. In: Proceedings of the 107th Annual Meeting of the American Association for Cancer Research; 2016 Apr 16-20; New Orleans, LA. Philadelphia (PA): AACR; Cancer Res 2016;76(14 Suppl):Abstract nr 849.
更多查看译文
AI 理解论文
溯源树
样例
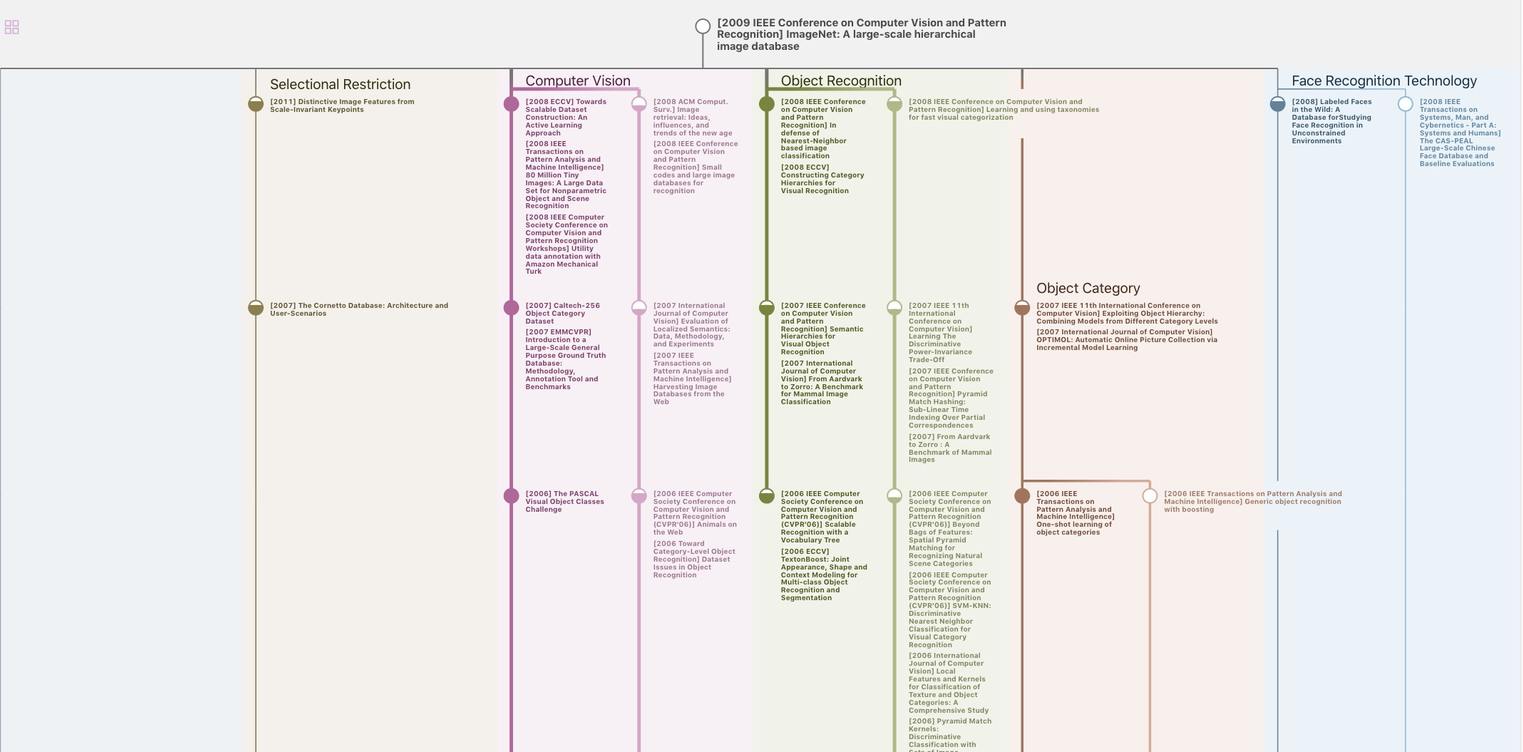
生成溯源树,研究论文发展脉络
Chat Paper
正在生成论文摘要