Imputing a variational inequality function or a convex objective function: A robust approach
Journal of Mathematical Analysis and Applications(2018)
摘要
To impute the function of a variational inequality and the objective of a convex optimization problem from observations of (nearly) optimal decisions, previous approaches constructed inverse programming methods based on solving a convex optimization problem [17], [7]. However, we show that, in addition to requiring complete observations, these approaches are not robust to measurement errors, while in many applications, the outputs of decision processes are noisy and only partially observable from, e.g., limitations in the sensing infrastructure. To deal with noisy and missing data, we formulate our inverse problem as the minimization of a weighted sum of two objectives: 1) a duality gap or Karush–Kuhn–Tucker (KKT) residual, and 2) a distance from the observations robust to measurement errors. In addition, we show that our method encompasses previous ones by generating a sequence of Pareto optimal points (with respect to the two objectives) converging to an optimal solution of previous formulations. To compare duality gaps and KKT residuals, we also derive new sub-optimality results defined by KKT residuals. Finally, an implementation framework is proposed with applications to delay function inference on the road network of Los Angeles, and consumer utility estimation in oligopolies.
更多查看译文
关键词
Convex optimization,Variational inequality,Inverse programming
AI 理解论文
溯源树
样例
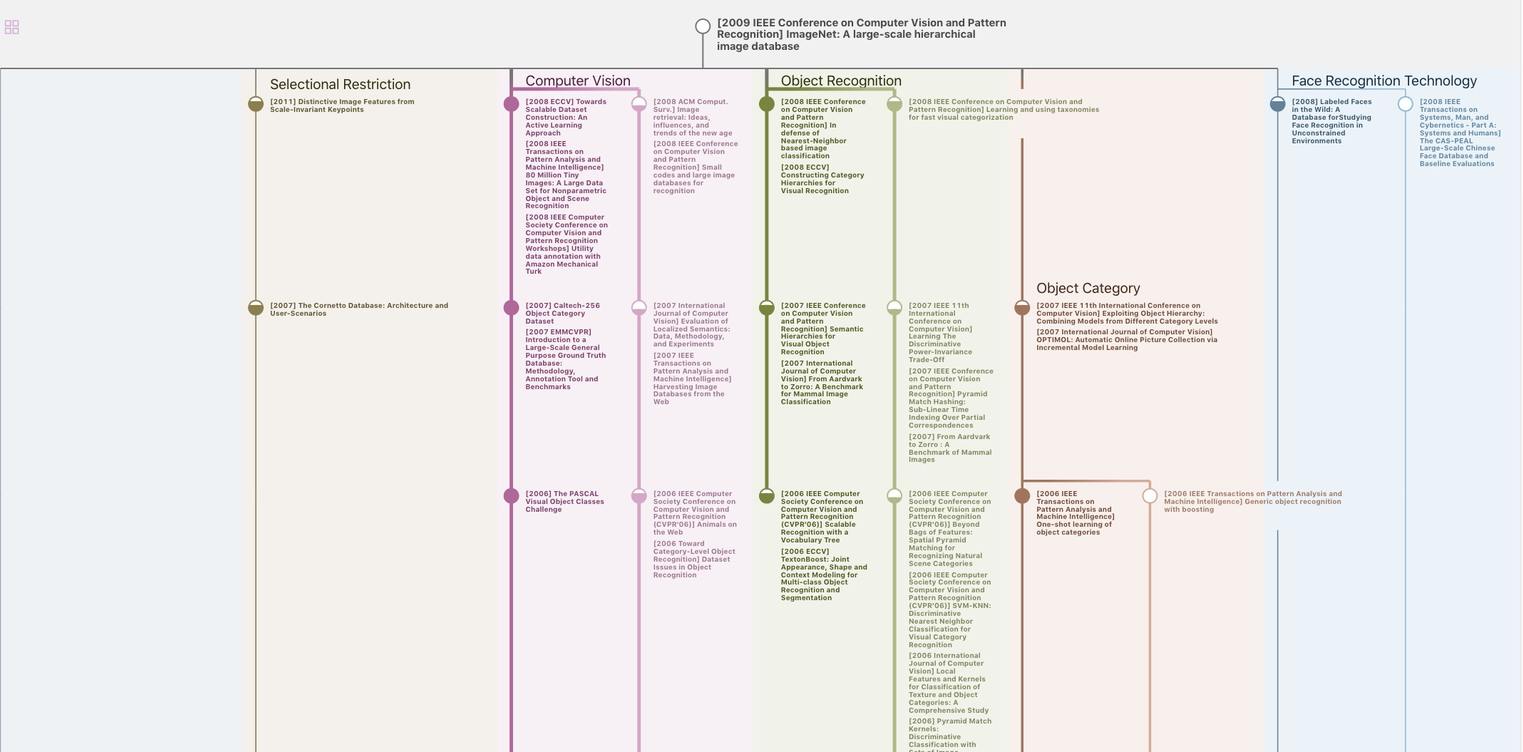
生成溯源树,研究论文发展脉络
Chat Paper
正在生成论文摘要