Parallel Computing for Copula Parameter Estimation with Big Data: A Simulation Study
arXiv: Methodology(2016)
摘要
Copula-based modeling has seen rapid advances in recent years. However, in big data applications, the lengthy computation time for estimating copula parameters is a major difficulty. Here, we develop a novel method to speed computation time in estimating copula parameters, using communication-free parallel computing. Our procedure partitions full data sets into disjoint independent subsets, performs copula parameter estimation on the subsets, and combines the results to produce an approximation to the full data copula parameter. We show in simulation studies that the computation time is greatly reduced through our method, using three well-known one-parameter bivariate copulas within the elliptical and Archimedean families: Gaussian, Frank and Gumbel. In addition, our simulation studies find small values for estimated bias, estimated mean squared error, and estimated relative L1 and L2 errors for our method, when compared to the full data parameter estimates.
更多查看译文
AI 理解论文
溯源树
样例
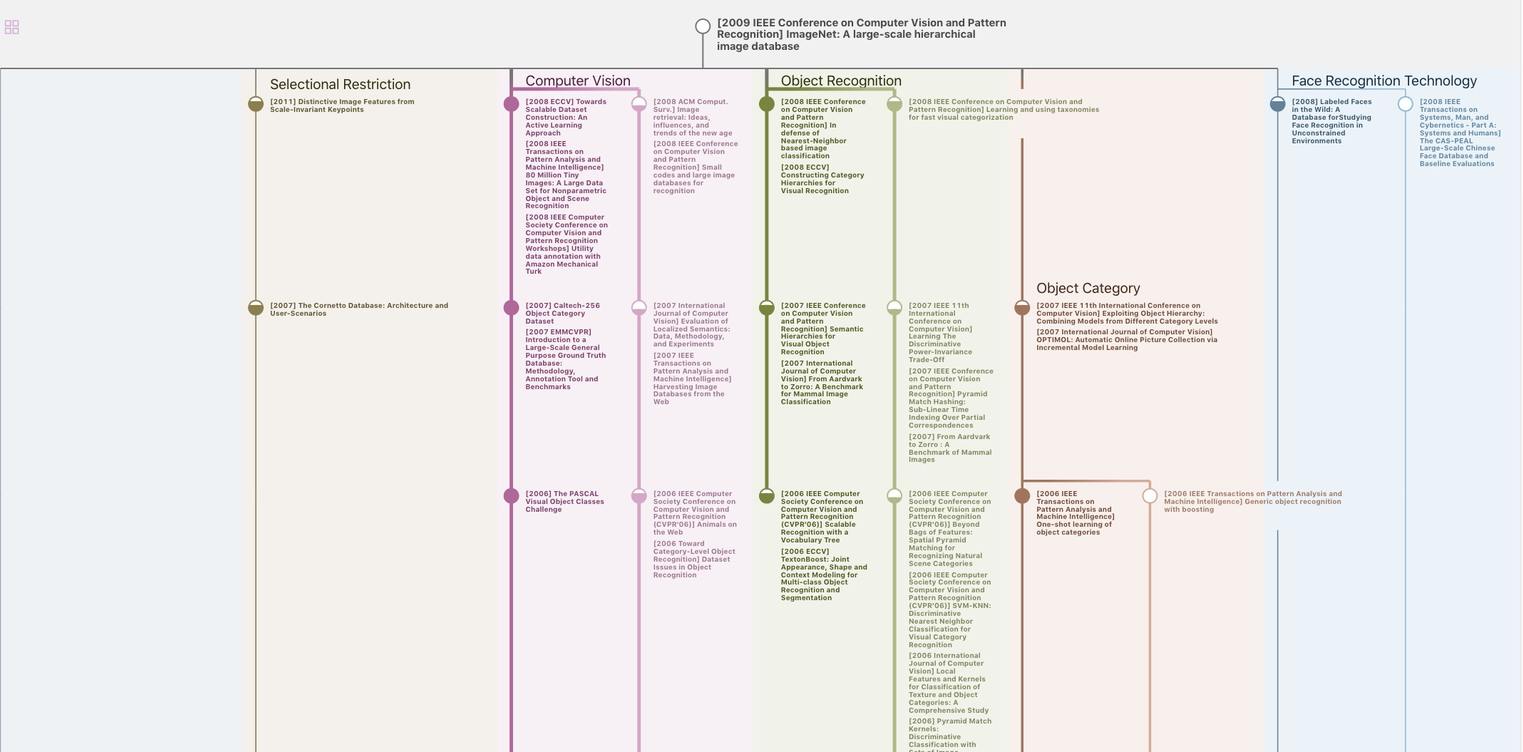
生成溯源树,研究论文发展脉络
Chat Paper
正在生成论文摘要