Examining nonstationarity in the recruitment dynamics of fishes using Bayesian change point analysis
CANADIAN JOURNAL OF FISHERIES AND AQUATIC SCIENCES(2017)
摘要
Marine ecosystems can undergo regime shifts, which result in nonstationarity in the dynamics of the fish populations inhabiting them. The assumption of time-invariant parameters in stock-recruitment models can lead to severe errors when forecasting renewal ability of stocks that experience shifts in their recruitment dynamics. We present a novel method for fitting stock-recruitment models using the Bayesian online change point detection algorithm, which is able to cope with sudden changes in the model parameters. We validate our method using simulations and apply it to empirical data of four demersal fishes in the southern Gulf of St. Lawrence. We show that all of the stocks have experienced shifts in their recruitment dynamics that cannot be captured by a model that assumes time-invariant parameters. The detected shifts in the recruitment dynamics result in clearly different parameter distributions and recruitment predictions between the regimes. This study illustrates how stock-recruitment relationships can experience shifts, which, if not accounted for, can lead to false predictions about a stock's recovery ability and resilience to fishing.
更多查看译文
AI 理解论文
溯源树
样例
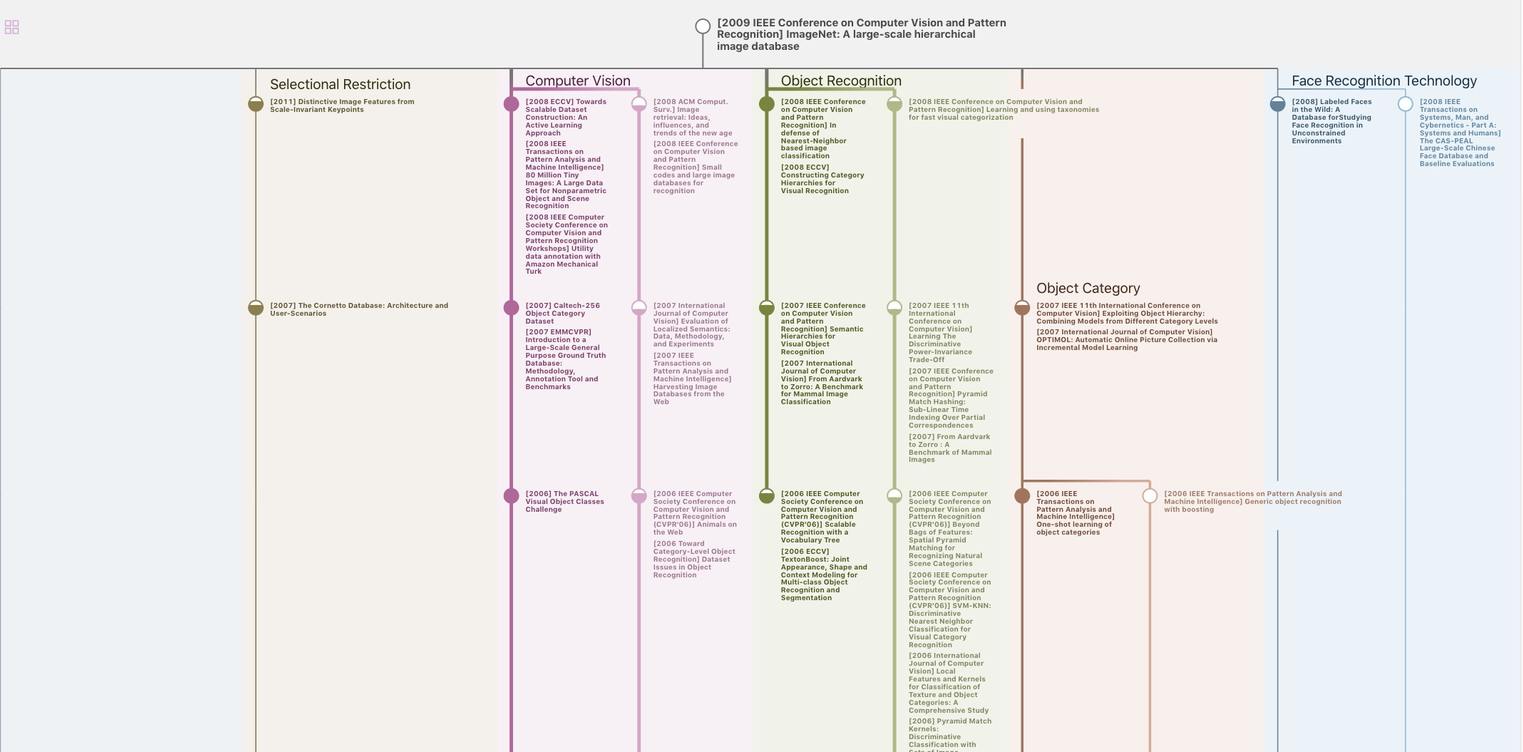
生成溯源树,研究论文发展脉络
Chat Paper
正在生成论文摘要