String-Averaging Incremental Subgradients For Constrained Convex Optimization With Applications To Reconstruction Of Tomographic Images
INVERSE PROBLEMS(2016)
摘要
We present a method for non-smooth convex minimization which is based on subgradient directions and string-averaging techniques. In this approach, the set of available data is split into sequences (strings) and a given iterate is processed independently along each string, possibly in parallel, by an incremental subgradient method. (ISM). The end-points of all strings are averaged to form the next iterate. The method is useful to solve sparse and large-scale non-smooth convex optimization problems, such as those arising in tomographic imaging. A convergence analysis is provided under realistic, standard conditions. Numerical tests are performed in a tomographic image reconstruction application, showing good performance for the convergence speed when measured as the decrease ratio of the objective function, in comparison to classical ISM.
更多查看译文
关键词
convex optimization, incremental algorithms, subgradient methods, projection methods, string-averaging algorithms
AI 理解论文
溯源树
样例
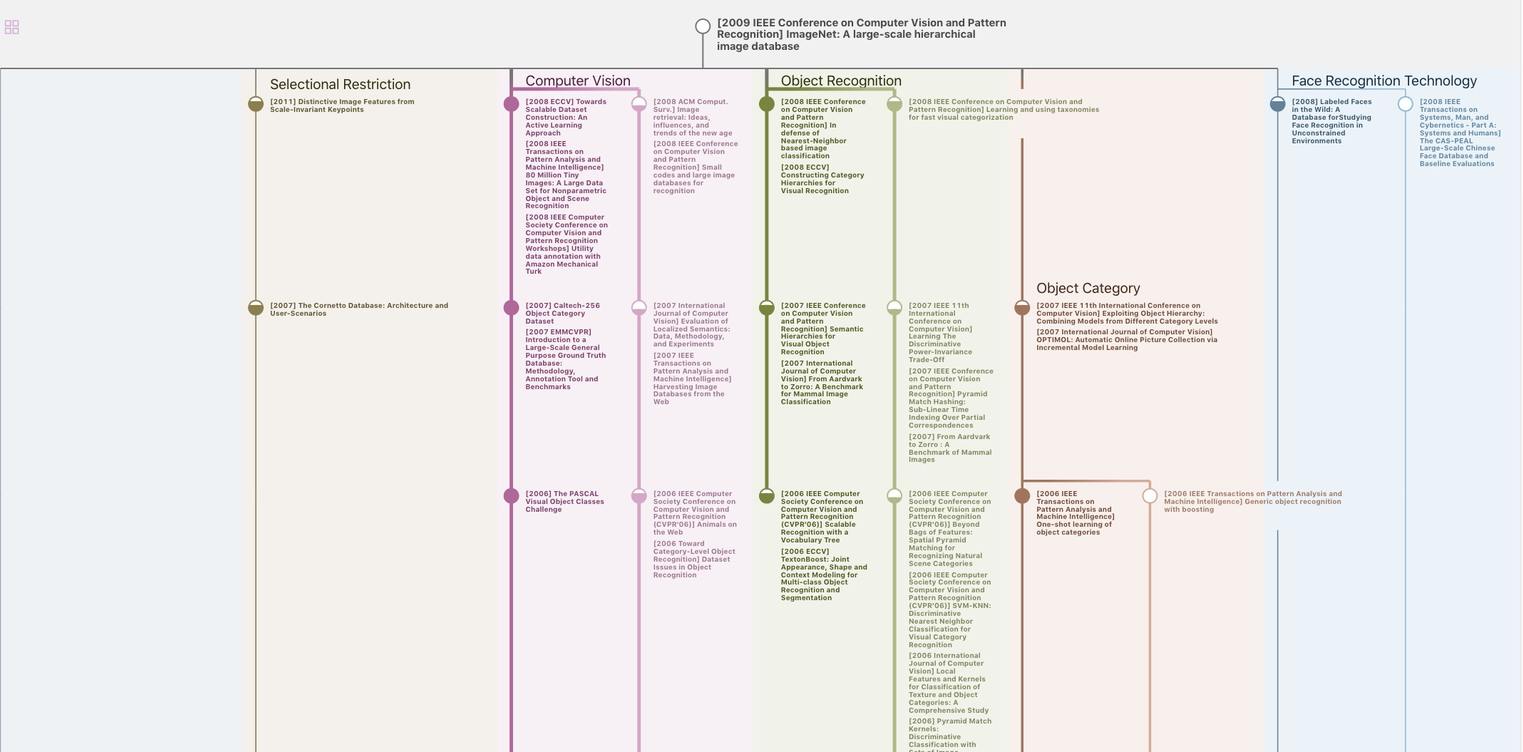
生成溯源树,研究论文发展脉络
Chat Paper
正在生成论文摘要