Sparse Representation Of Salient Regions For No-Reference Image Quality Assessment
INTERNATIONAL JOURNAL OF ADVANCED ROBOTIC SYSTEMS(2016)
摘要
This paper introduces an efficient feature learning framework via sparse coding for no-reference image quality assessment. The important part of the proposed framework is based on sparse feature extraction from a sparse representation matrix, which is computed using a sparse coding algorithm. Image patches extracted from salient regions of unlabeled images are used to learn a dictionary of sparse coding. The l1-norm of the sparse representation is taken as a sparse penalty term in the process of learning the dictionary and computing the sparse representation. A feature detector adopts the l1-norm together with the max-pooling results of the sparse representation matrix as the output sparse features to obtain the objective quality scores. Sparse features of salient regions are evaluated using the LIVE, CSIQ and TID2013 databases, and result in good generalization ability, performing better than or on par with other image quality assessment algorithms.
更多查看译文
关键词
l1-norm, no-reference image quality assessment, sparse coding, sparse representation
AI 理解论文
溯源树
样例
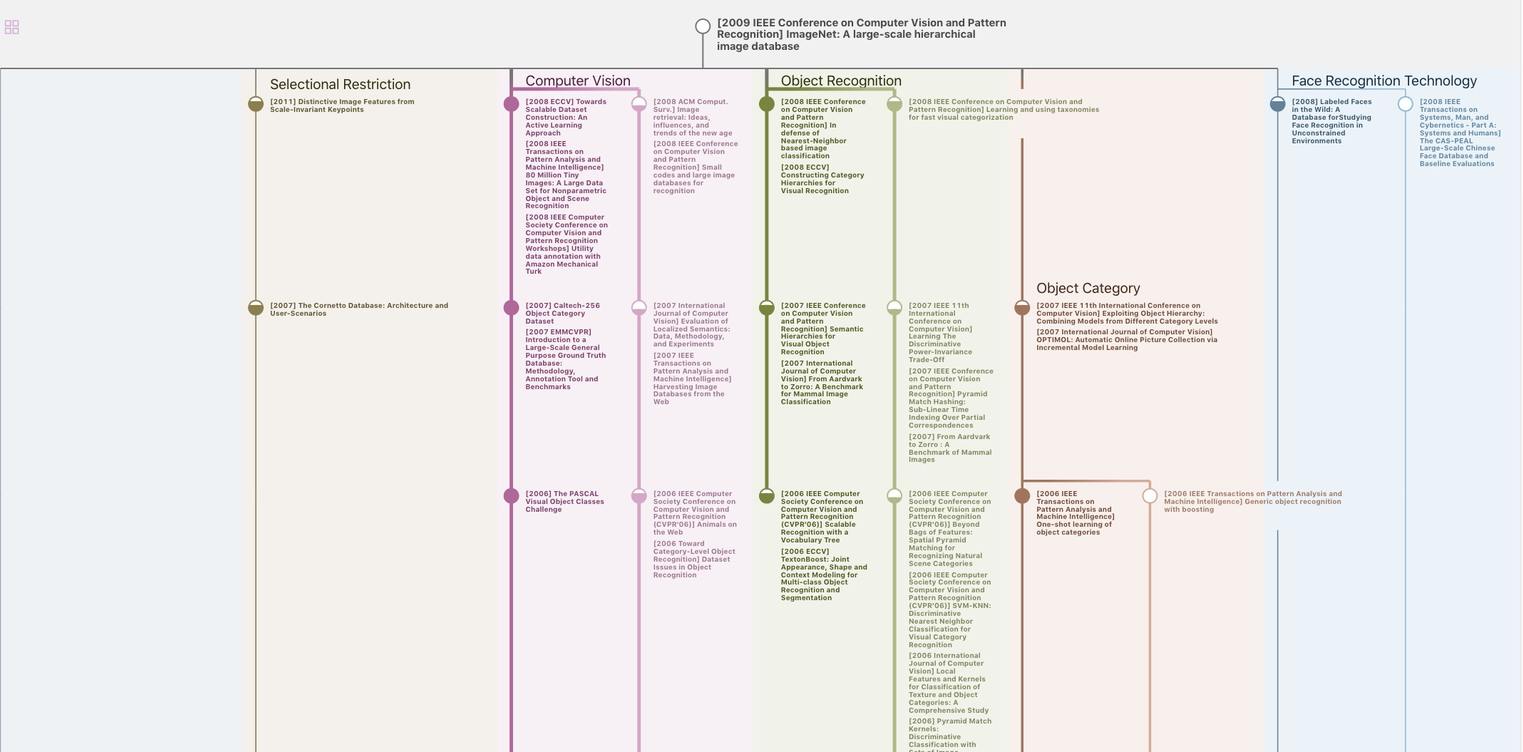
生成溯源树,研究论文发展脉络
Chat Paper
正在生成论文摘要