Robust nonlinear system identification: Bayesian mixture of experts using the t-distribution
Mechanical Systems and Signal Processing(2017)
摘要
A novel variational Bayesian mixture of experts model for robust regression of bifurcating and piece-wise continuous processes is introduced. The mixture of experts model is a powerful model which probabilistically splits the input space allowing different models to operate in the separate regions. However, current methods have no fail-safe against outliers. In this paper, a robust mixture of experts model is proposed which consists of Student-t mixture models at the gates and Student-t distributed experts, trained via Bayesian inference. The Student-t distribution has heavier tails than the Gaussian distribution, and so it is more robust to outliers, noise and non-normality in the data. Using both simulated data and real data obtained from the Z24 bridge this robust mixture of experts performs better than its Gaussian counterpart when outliers are present. In particular, it provides robustness to outliers in two forms: unbiased parameter regression models, and robustness to overfitting/complex models.
更多查看译文
关键词
Outliers,Robust estimation,Student-t distribution,Variational Bayes,Mixture of experts,Bifurcating mechanical structures
AI 理解论文
溯源树
样例
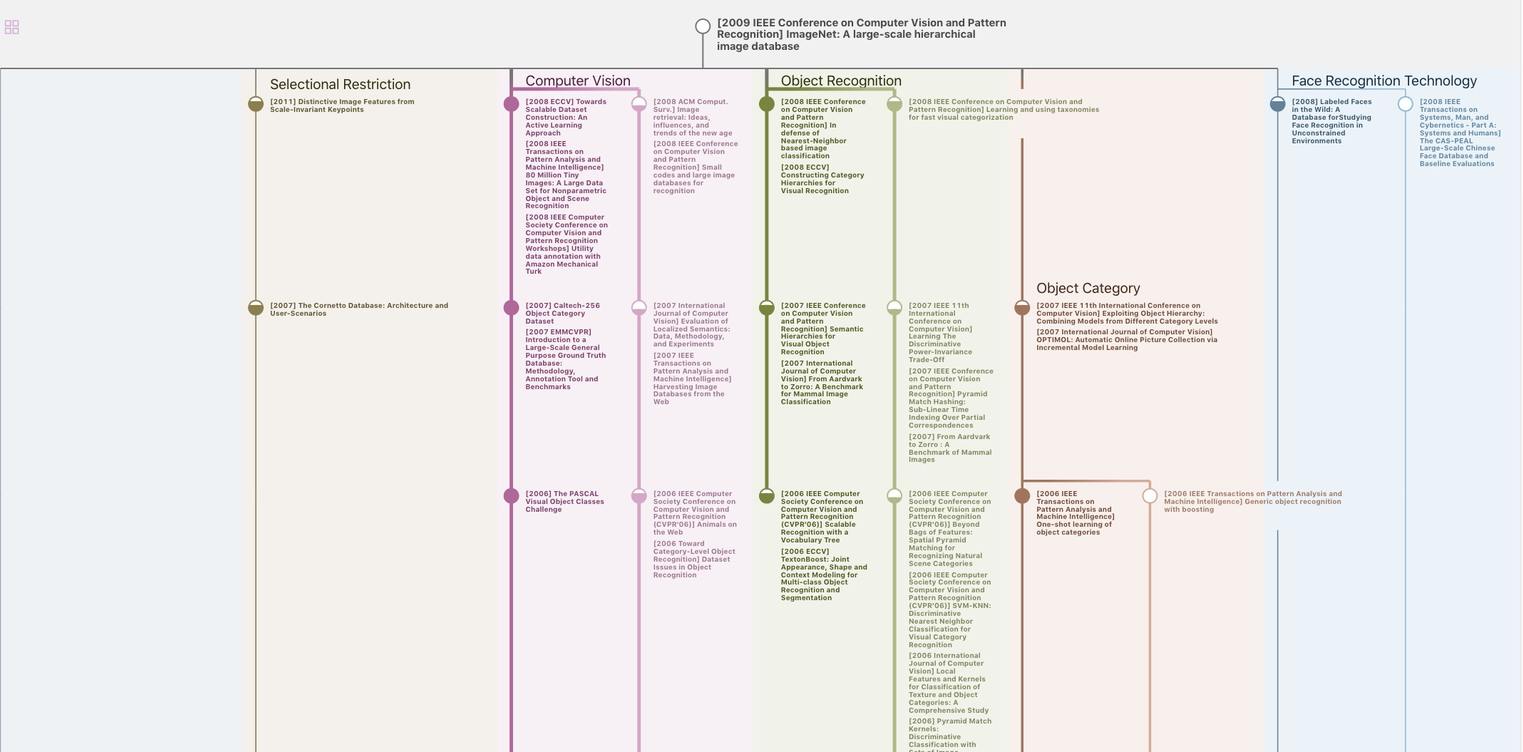
生成溯源树,研究论文发展脉络
Chat Paper
正在生成论文摘要