Robust text-to-speech duration modelling with a deep neural network
Journal of the Acoustical Society of America(2016)
摘要
Accurate modeling and prediction of speech-sound durations is important for generating more natural synthetic speech. Deep neural networks (DNNs) offer powerful models, and large, found corpora of natural speech are easily acquired for training them. Unfortunately, poor quality control (e.g., transcription errors) and phenomena such as reductions and filled pauses complicate duration modelling from found speech data. To mitigate issues caused by these idiosyncrasies, we propose to incorporate methods from robust statistics into speech synthesis. Robust methods can disregard ill-fitting training-data points—errors or other outliers—to describe the typical case better. For instance, parameter estimation can be made robust by replacing maximum likelihood with a robust estimation criterion based on the density power divergence (a.k.a. the β-divergence). Alternatively, a standard approximation for output generation with mixture density networks (MDNs) can be interpreted as a robust output generation heuristic....
更多查看译文
AI 理解论文
溯源树
样例
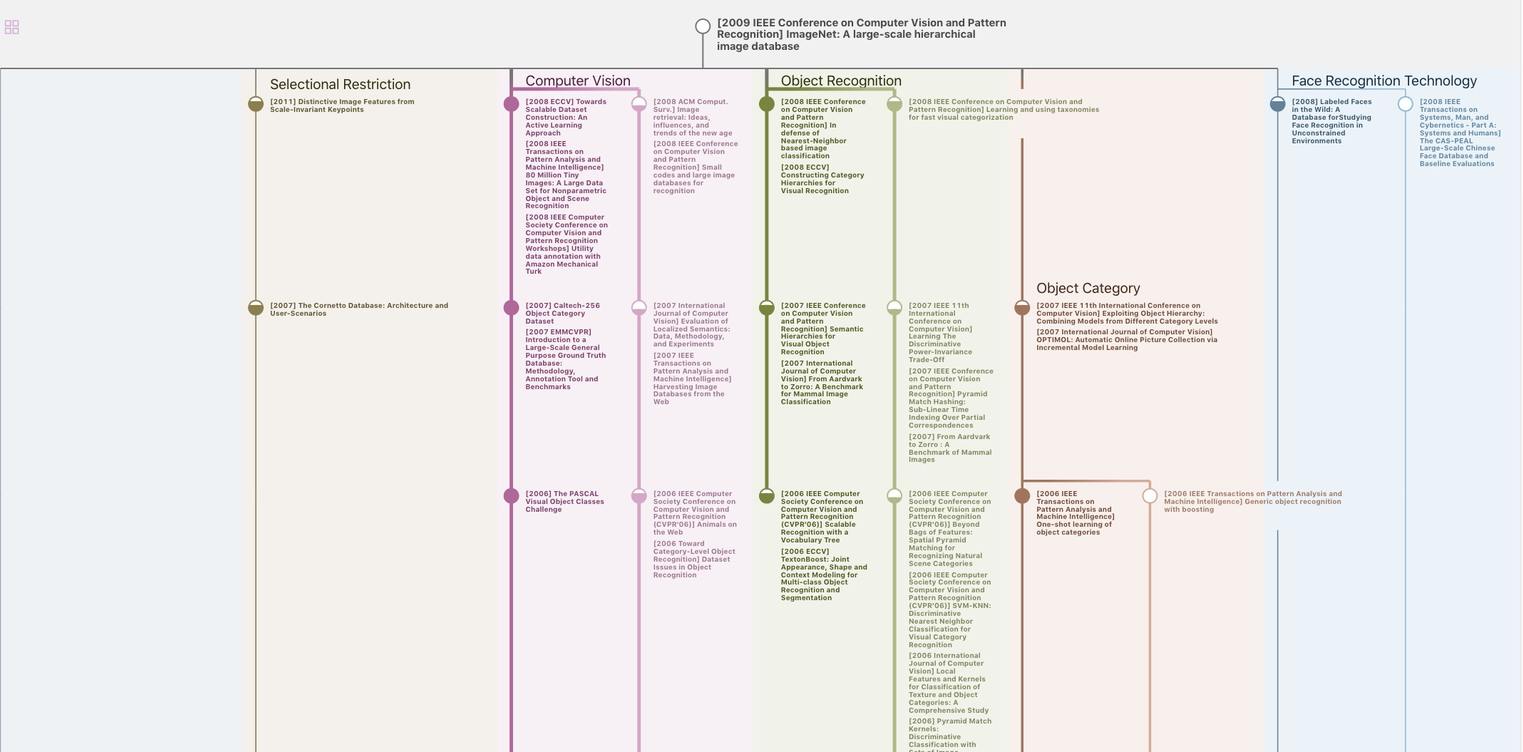
生成溯源树,研究论文发展脉络
Chat Paper
正在生成论文摘要