Weighted scoring rules and hypothesis testing
arXiv: Methodology(2016)
摘要
We discuss weighted scoring rules for forecast evaluation and their connection to hypothesis testing. First, a general construction principle for strictly locally proper weighted scoring rules based on conditional densities and scoring rules for probability forecasts is proposed. We show how likelihood-based weighted scoring rules from the literature fit into this framework, and also introduce a weighted version of the Hyvärinen score, which is a local scoring rule in the sense that it only depends on the forecast density and its derivatives at the observation, and does not require evaluation of integrals. Further, we discuss the relation to hypothesis testing. Using a weighted scoring rule introduces a censoring mechanism, in which the form of the density is irrelevant outside the region of interest. For the resulting testing problem with composite null - and alternative hypotheses, we construct optimal tests, and identify the associated weighted scoring rule. As a practical consequence, using a weighted scoring rule allows to decide in favor of a forecast which is superior to a competing forecast on a region of interest, even though it may be inferior outside this region. A simulation study and an application to financial time-series data illustrate these findings.
更多查看译文
AI 理解论文
溯源树
样例
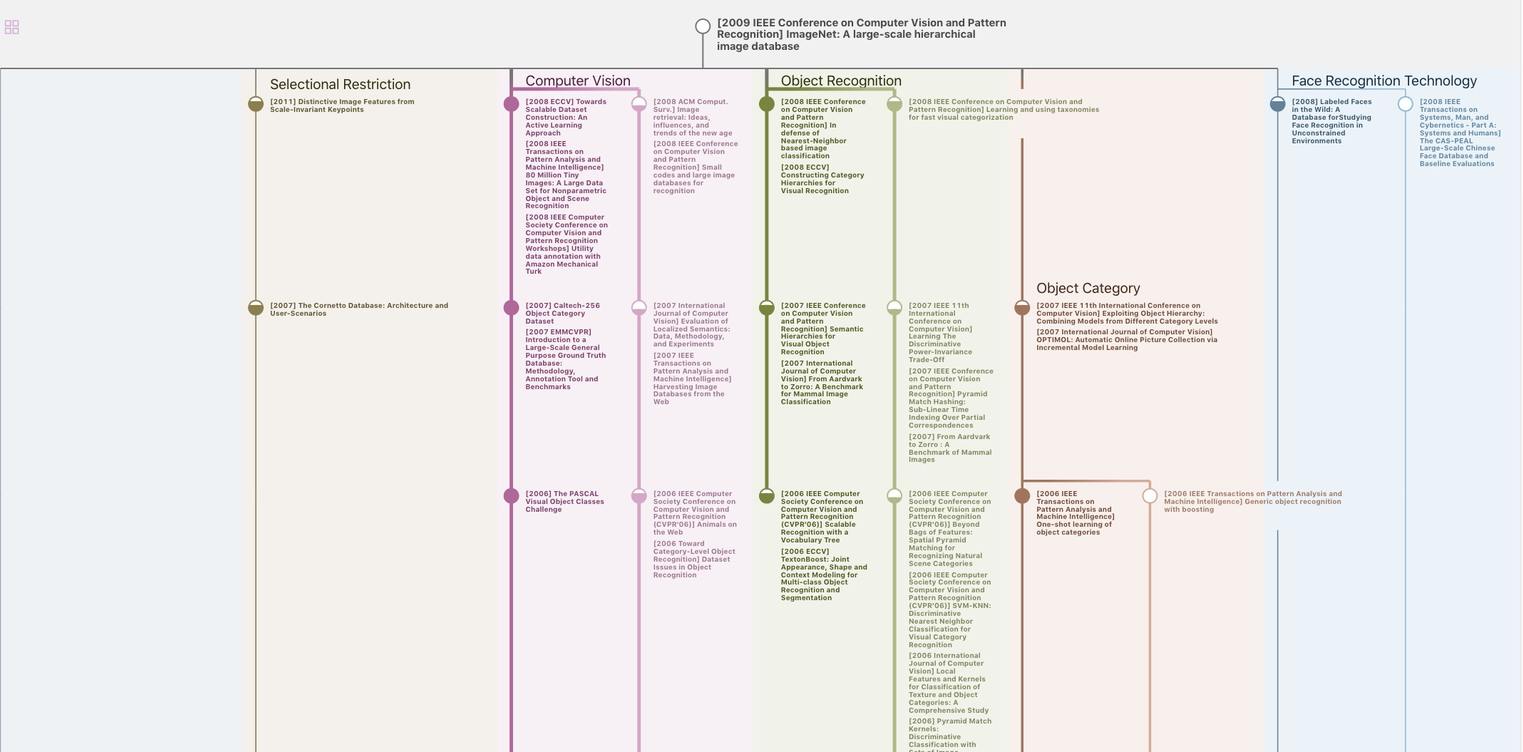
生成溯源树,研究论文发展脉络
Chat Paper
正在生成论文摘要