Short-Term Load Forecasting Using Adaptive Annealing Learning Algorithm Based Reinforcement Neural Network
ENERGIES(2016)
摘要
A reinforcement learning algorithm is proposed to improve the accuracy of short-term load forecasting (STLF) in this article. The proposed model integrates radial basis function neural network (RBFNN), support vector regression (SVR), and adaptive annealing learning algorithm (AALA). In the proposed methodology, firstly, the initial structure of RBFNN is determined by using an SVR. Then, an AALA with time-varying learning rates is used to optimize the initial parameters of SVR-RBFNN (AALA-SVR-RBFNN). In order to overcome the stagnation for searching optimal RBFNN, a particle swarm optimization (PSO) is applied to simultaneously find promising learning rates in AALA. Finally, the short-term load demands are predicted by using the optimal RBFNN. The performance of the proposed methodology is verified on the actual load dataset from the Taiwan Power Company (TPC). Simulation results reveal that the proposed AALA-SVR-RBFNN can achieve a better load forecasting precision compared to various RBFNNs.
更多查看译文
关键词
short-term load forecasting,radial basis function neural network,support vector regression,particle swarm optimization,adaptive annealing learning algorithm
AI 理解论文
溯源树
样例
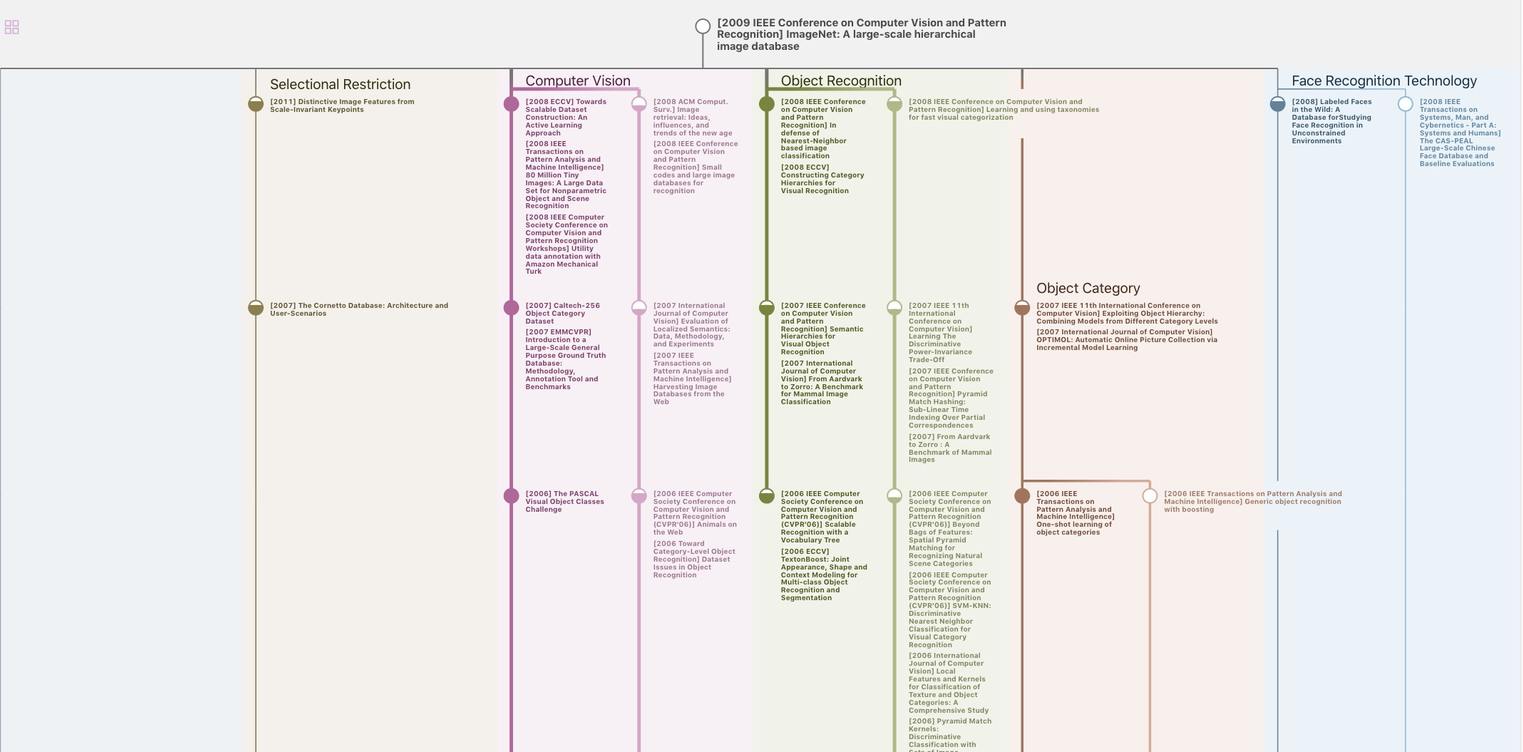
生成溯源树,研究论文发展脉络
Chat Paper
正在生成论文摘要