Universal structural estimator and dynamics approximator for complex networks
arXiv: Physics and Society(2016)
摘要
Revealing the structure and dynamics of complex networked systems from observed data is of fundamental importance to science, engineering, and society. Is it possible to develop a universal, completely data driven framework to decipher the network structure and different types of dynamical processes on complex networks, regardless of their details? We develop a Markov network based model, sparse dynamical Boltzmann machine (SDBM), as a universal network structural estimator and dynamics approximator. The SDBM attains its topology according to that of the original system and is capable of simulating the original dynamical process. We develop a fully automated method based on compressive sensing and machine learning to find the SDBM. We demonstrate, for a large variety of representative dynamical processes on model and real world complex networks, that the equivalent SDBM can recover the network structure of the original system and predicts its dynamical behavior with high precision.
更多查看译文
AI 理解论文
溯源树
样例
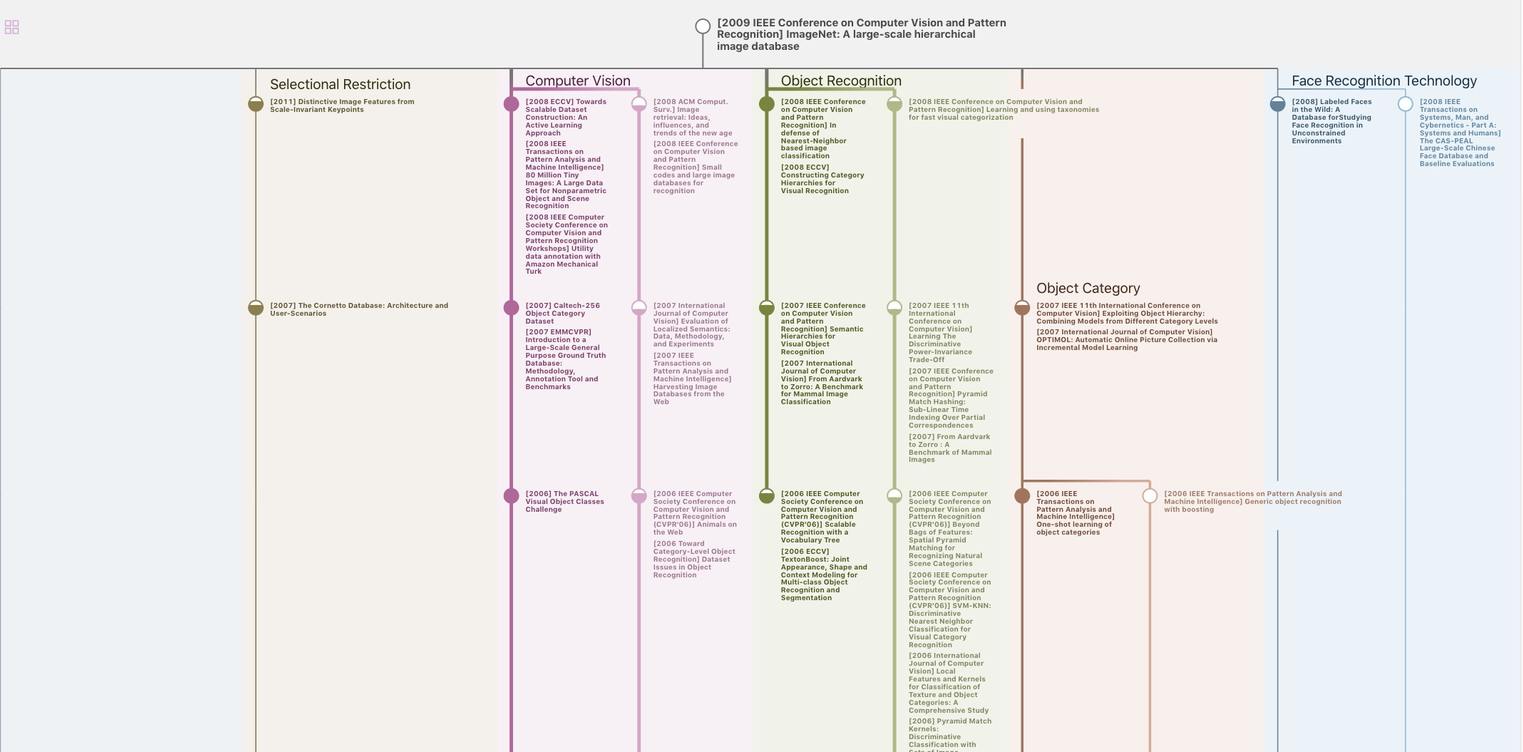
生成溯源树,研究论文发展脉络
Chat Paper
正在生成论文摘要