Kernel-Based Online Object Tracking Via Gaussian Mixture Model Learning
PROCEEDINGS OF 2016 SIXTH INTERNATIONAL CONFERENCE ON INSTRUMENTATION & MEASUREMENT, COMPUTER, COMMUNICATION AND CONTROL (IMCCC 2016)(2016)
摘要
Object tracking has attracted increasing attention and requires difficult object appearance recognition and learning. This paper proposes a novel object tracking by matching corresponding SURF-based keypoints. A dynamic 2D scale-rotation space is constructed for each object keypoint to strengthen its variation and distinctiveness. During matching, we assign each keypoint a kernel weight employing Gaussian mixture model, to make sure those keypoints with higher repeatability and reliability are used. Meanwhile, improved weighted RANSAC is applied to estimate motion parameters. Finally, on-line learning is performed on SURF feature, 2D scale-rotation space and Gaussian mixture model once tracking is successful. Experimental results using both public and private image sequences validate the robustness and accuracy of the proposed method under complex scene changes.
更多查看译文
关键词
objec tracking,kernel function,Gaussian mixuture model,feature matching,online learning
AI 理解论文
溯源树
样例
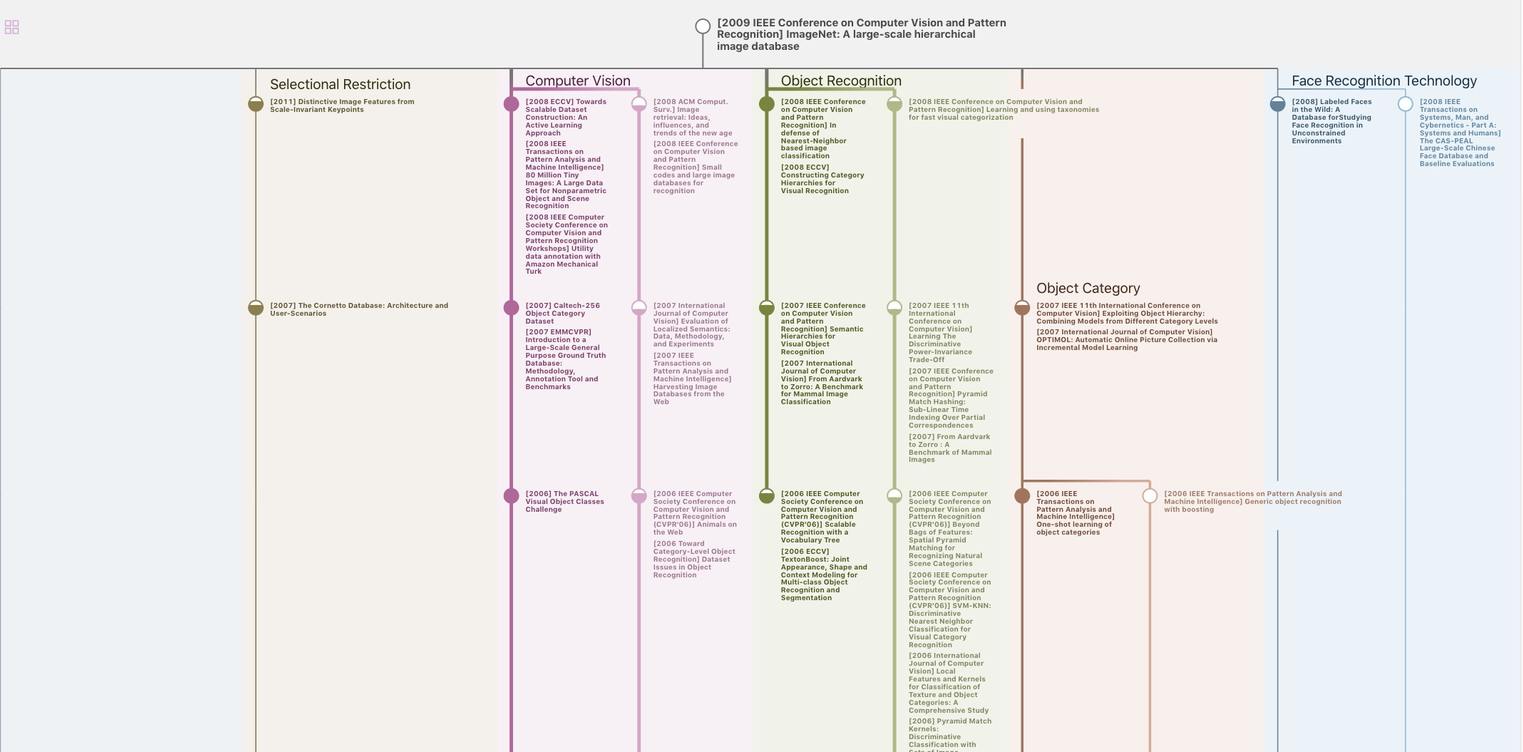
生成溯源树,研究论文发展脉络
Chat Paper
正在生成论文摘要