A new approach to theoretical investigations of high harmonics generation by means of fs laser interaction with overdense plasma layers. Combining particle-in-cell simulations with machine learning.
JOURNAL OF INSTRUMENTATION(2016)
摘要
Within the past decade, various experimental and theoretical investigations have been performed in the field of high-order harmonics generation (HHG) by means of femtosecond (fs) laser pulses interacting with laser produced plasmas. Numerous potential future applications thus arise. Beyond achieving higher conversion efficiency for higher harmonic orders and hence harmonic power and brilliance, there are more ambitious scientific goals such as attaining shorter harmonic wavelengths or reducing harmonic pulse durations towards the attosecond and even the zeptosecond range. High order harmonics are also an attractive diagnostic tool for the laser-plasma interaction process itself. Particle-in-Cell (PIC) simulations are known to be one of the most important numerical instruments employed in plasma physics and in laser-plasma interaction investigations. The novelty brought by this paper consists in combining the PIC method with several machine learning approaches. For predictive modelling purposes, a universal functional approximator is used, namely a multi-layer perceptron (MLP), in conjunction with a self-organizing map (SOM). The training sets have been retrieved from the PIC simulations and also from the available literature in the field. The results demonstrate the potential utility of machine learning in predicting optimal interaction scenarios for gaining higher order harmonics or harmonics with particular features such as a particular wavelength range, a particular harmonic pulse duration or a certain intensity. Furthermore, the author will show how machine learning can be used for estimations of electronic temperatures, proving that it can be a reliable tool for obtaining better insights into the fs laser interaction physics.
更多查看译文
关键词
Data processing methods,Pattern recognition,cluster finding,calibration and fitting methods,Analysis and statistical methods,Simulation methods and programs
AI 理解论文
溯源树
样例
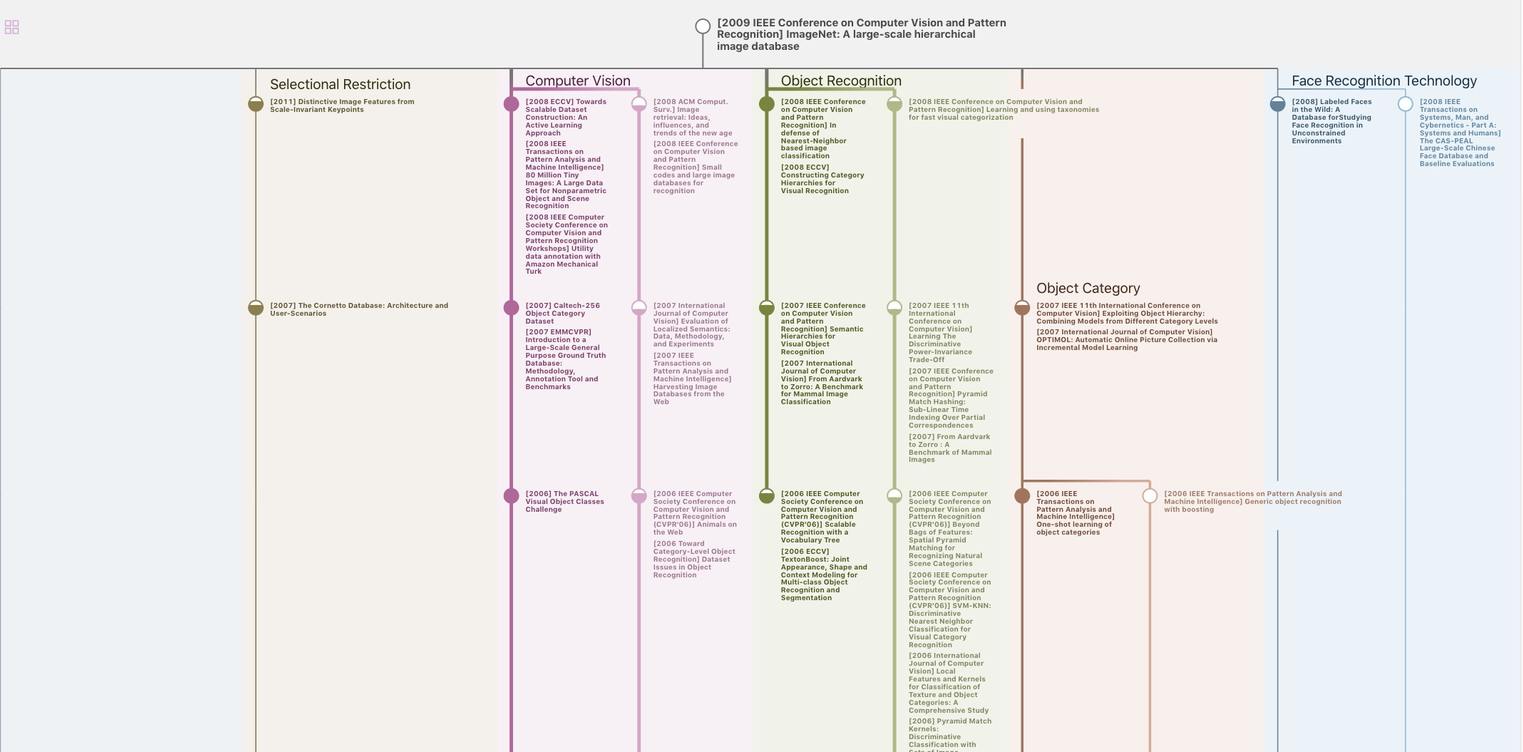
生成溯源树,研究论文发展脉络
Chat Paper
正在生成论文摘要