Identification of Causal Effects using Conditional Auxiliary Instruments
arXiv: Methodology(2016)
摘要
In this paper, we provide a novel algorithm for the identification of parameters in linear structural equation models (SEMs) that subsumes the previous state-of-the-art method. This algorithm combines instrumental with variables, which allow the incorporation of external or newly acquired knowledge into the SEM. In order to apply variables to instrumental sets, we first graphically characterize conditional independence between and model variables. This characterization allows variables to enhance identification and model testing techniques that rely on recognizing conditional independences implied by the model structure (e.g. the single-door criterion, instrumental variables, instrumental sets, d-separation, and more). We then give an algorithm that applies these auxiliary instrumental sets to identify as many coefficients as possible in a linear SEM and demonstrate that it is able to identify strictly more coefficients and models than the existing state of the art methods. Lastly, we discuss applications of our results to the problems of model testing and identification of causal effects with limited experimental data.
更多查看译文
AI 理解论文
溯源树
样例
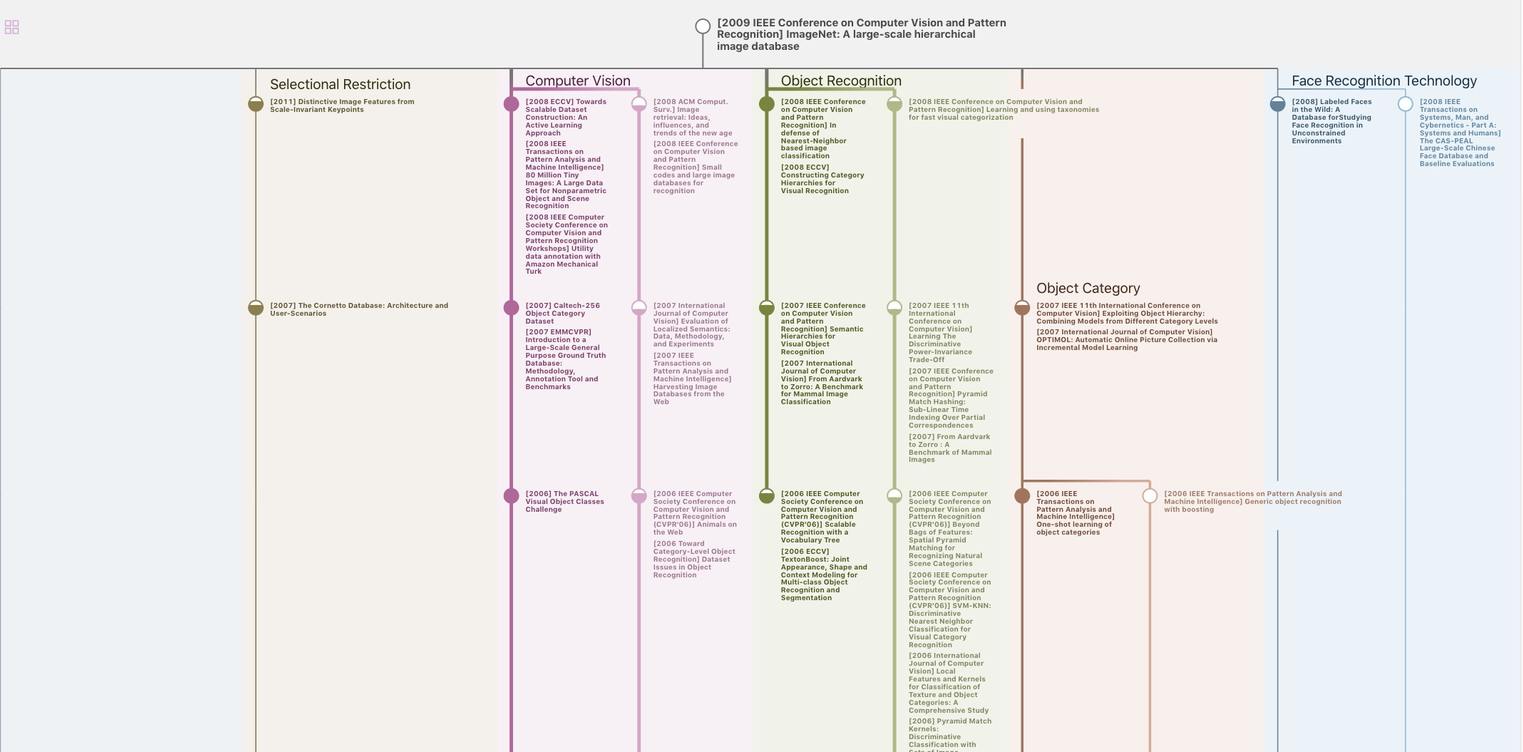
生成溯源树,研究论文发展脉络
Chat Paper
正在生成论文摘要