Monte Carlo Structured SVI for Non-Conjugate Models
arXiv: Machine Learning(2016)
摘要
The stochastic variational inference (SVI) paradigm, which combines variational inference, natural gradients, and stochastic updates, was recently proposed for large-scale data analysis in conjugate Bayesian models and demonstrated to be effective in several problems. This paper studies a family of Bayesian latent variable models with two levels of hidden variables but without any conjugacy requirements, making several contributions in this context. The first is observing that SVI, with an improved structured variational approximation, is applicable under more general conditions than previously thought with the only requirement being that the approximating variational distribution be in the same family as the prior. The resulting approach, Monte Carlo Structured SVI (MC-SSVI), significantly extends the scope of SVI, enabling large-scale learning in non-conjugate models. The second contribution is developing the algorithmic details of MC-SSVI for two challenging models. The application of MC-SSVI to probabilistic matrix factorization (PMF) yields an algorithm which is efficient and generic in that it is applicable to any type of observation likelihood, with improvements in convergence speed and in general applicability over previous work. The application of MC-SSVI to the correlated topic model (CTM) improves over previous work which used the much stronger mean field variational approximation. An experimental evaluation demonstrates the advantages of MC-SSVI.
更多查看译文
AI 理解论文
溯源树
样例
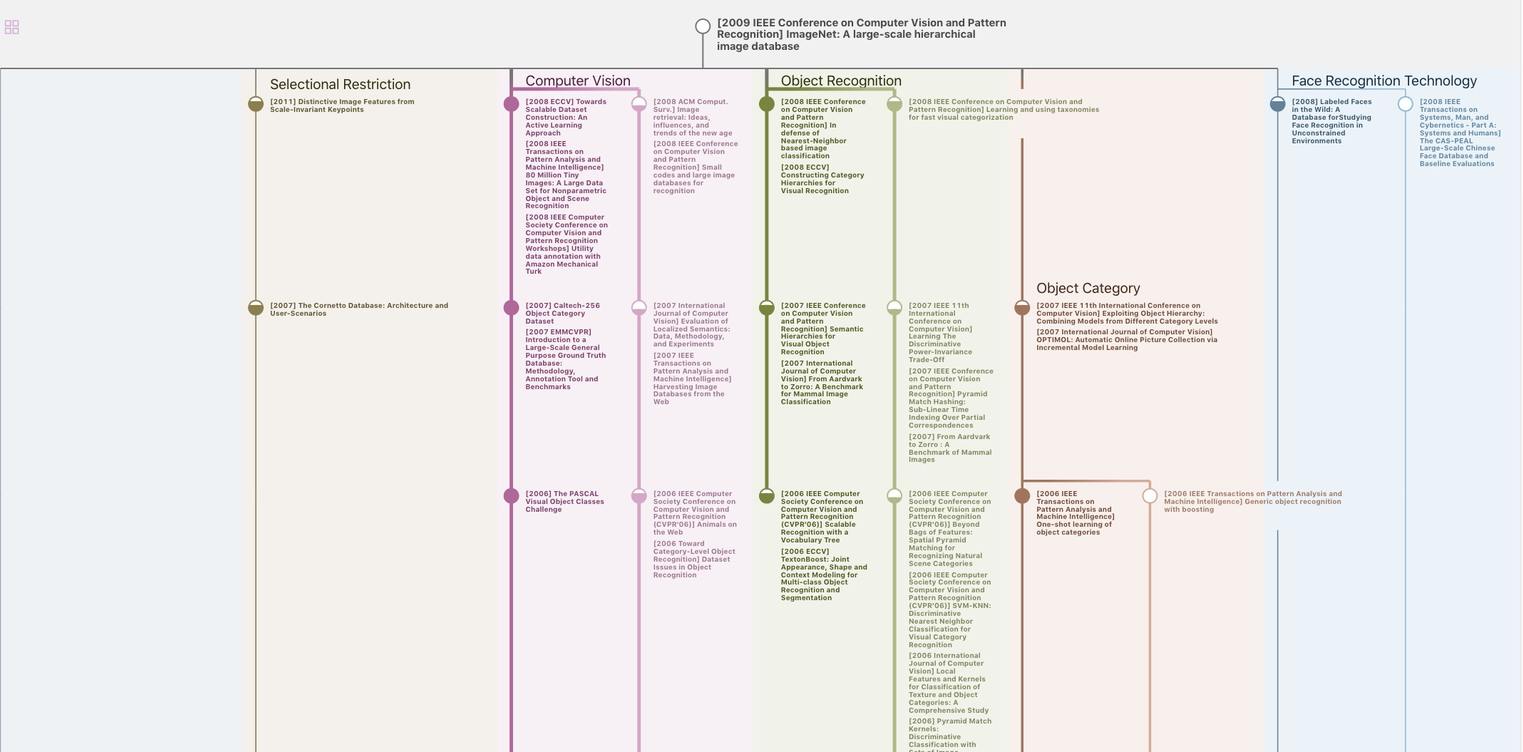
生成溯源树,研究论文发展脉络
Chat Paper
正在生成论文摘要