Comparison of time series forecasting techniques with respect to tolerance to noise
2016 IEEE International Autumn Meeting on Power, Electronics and Computing (ROPEC)(2016)
摘要
Noise is ubiquitous in the production of time series; we cannot assume that our source data is clean, data is always (most of the time) contaminated with noise. Noise may come from different sources: measuring devices, transmission means, etc. This article presents an analysis and comparison of how the presence of noise affects different forecasting techniques. Since chaotic time series are the most difficult to predict, we base our study on this kind of time series. Furthermore, there exist several small mathematical models that exhibit chaotic behavior. We can produce clean data by integrating those models over time. We then add noise at different levels of Noise to Signal Ratios, and measure the performance of the models produced by different forecasting techniques. The forecasting techniques included in this comparison are Nearest Neighbors, Artificial Neural Networks, ARIMA, Fuzzy Neural Networks, and Nearest Neighbors combined with Differential Evolution. Among all of them, the technique that performs better and is less affected by noise is Nearest Neighbors combined with Differential Evolution.
更多查看译文
关键词
time series forecasting,clean data,mathematical models,noise to signal ratio,nearest neighbors,artificial neural networks,ARIMA,fuzzy neural networks,differential evolution,autoregressive integrated moving average
AI 理解论文
溯源树
样例
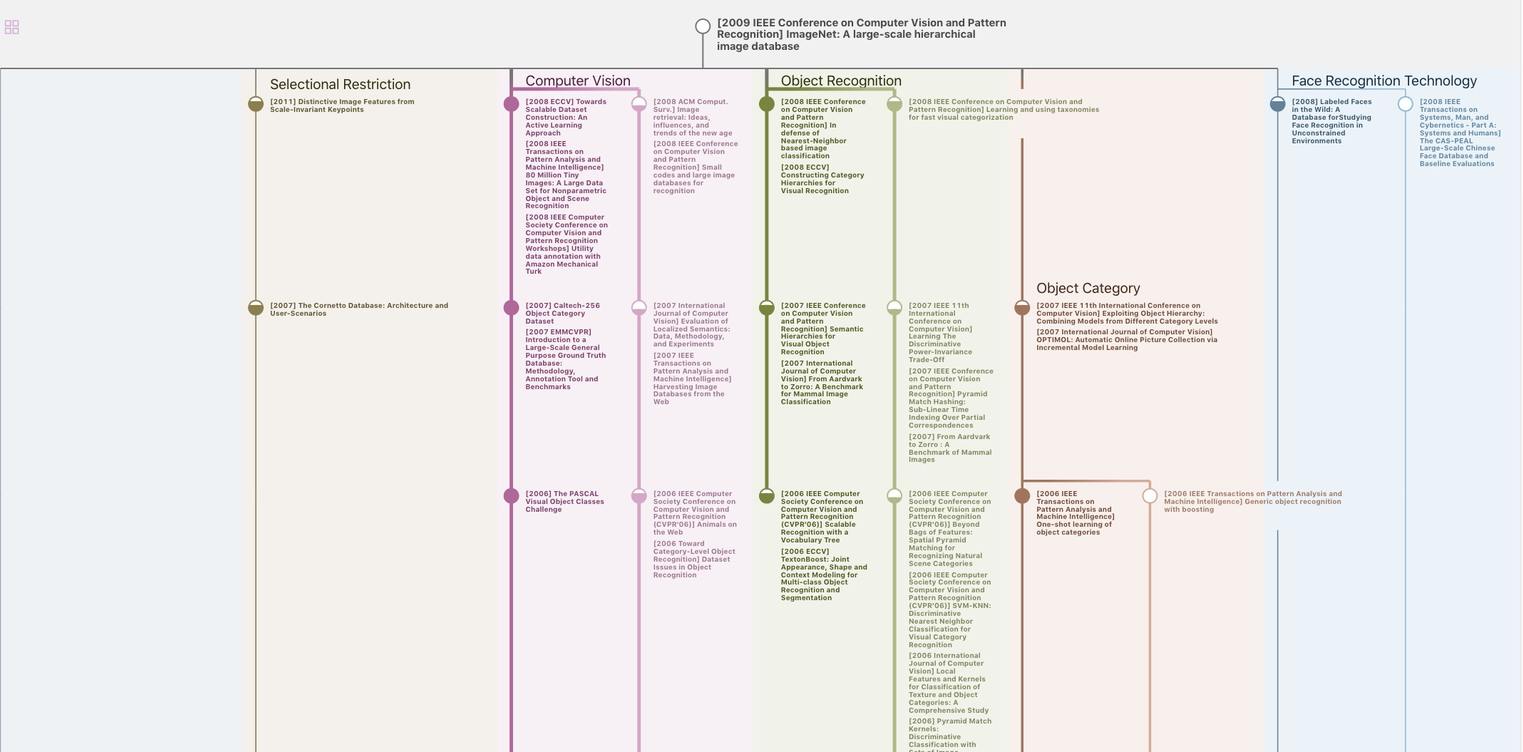
生成溯源树,研究论文发展脉络
Chat Paper
正在生成论文摘要