Bootstrap Methods For Long-Memory Processes: A Review
COMMUNICATIONS FOR STATISTICAL APPLICATIONS AND METHODS(2017)
摘要
This manuscript summarized advances in bootstrap methods for long-range dependent time series data. The stationary linear long-memory process is briefly described, which is a target process for bootstrap methodologies on time-domain and frequency-domain in this review. We illustrate time-domain bootstrap under long-range dependence, moving or non-overlapping block bootstraps, and the autoregressive-sieve bootstrap. In particular, block bootstrap methodologies need an adjustment factor for the distribution estimation of the sample mean in contrast to applications to weak dependent time processes. However, the autoregressive-sieve bootstrap does not need any other modification for application to long-memory. The frequency domain bootstrap for Whittle estimation is provided using parametric spectral density estimates because there is no current nonparametric spectral density estimation method using a kernel function for the linear long-range dependent time process.
更多查看译文
关键词
autoregressive-sieve bootstrap, block bootstrap, frequency domain bootstrap, long-memory
AI 理解论文
溯源树
样例
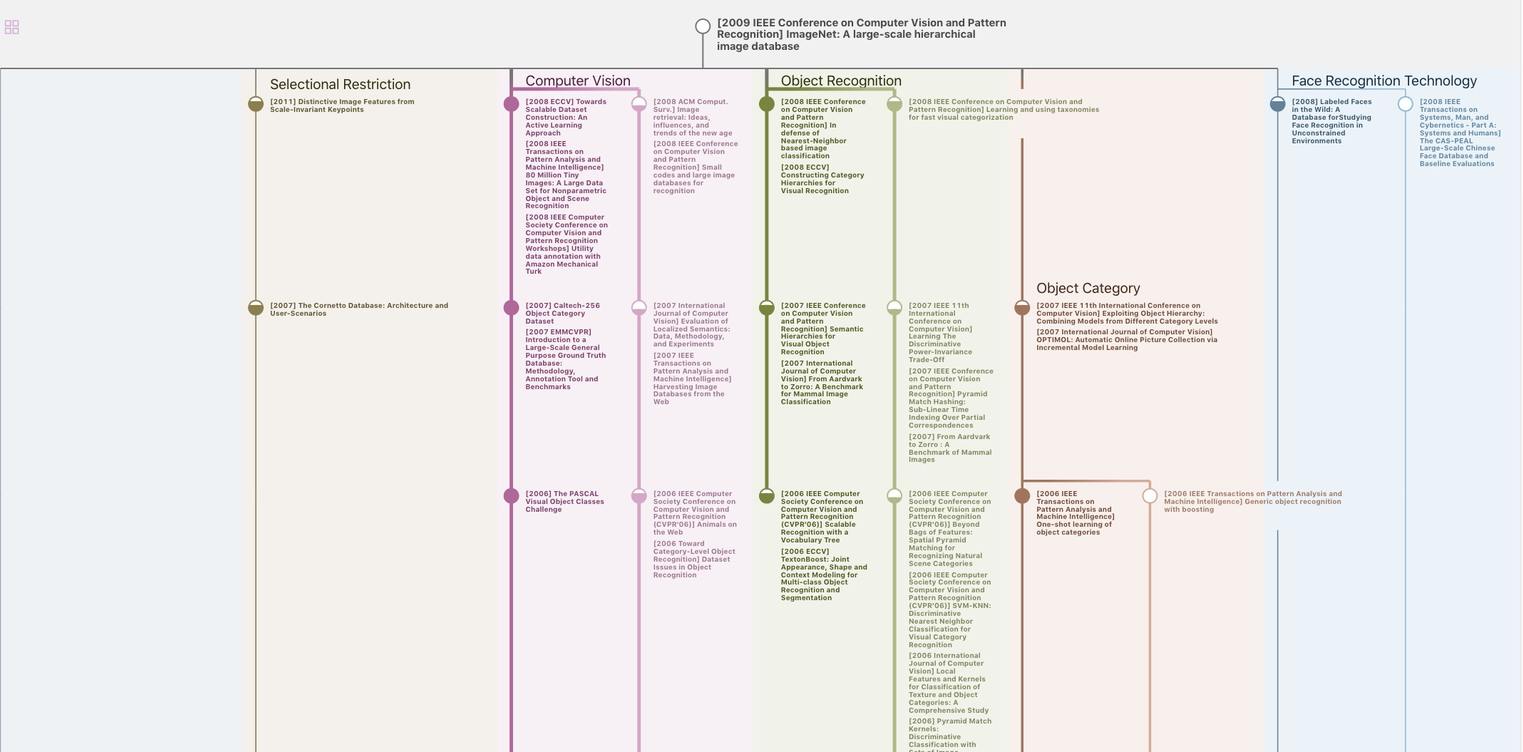
生成溯源树,研究论文发展脉络
Chat Paper
正在生成论文摘要