Model Predictive Control oriented experiment design for system identification: A graph theoretical approach
Journal of Process Control(2017)
摘要
We present a new approach to Model Predictive Control (MPC) oriented experiment design for the identification of systems operating in closed-loop. The method considers the design of an experiment by minimizing the experimental cost, subject to probabilistic bounds on the input and output signals due to physical limitations of actuators, and quality constraints on the identified model. The excitation is done by intentionally adding a disturbance to the loop. We then design the external excitation to achieve the minimum experimental effort while we are also taking care of the tracking performance of MPC. The stability of the closed-loop system is guaranteed by employing robust MPC during the experiment. The problem is then defined as an optimization problem. However, the aforementioned constraints result in a non-convex optimization which is relaxed by using results from graph theory. The proposed technique is evaluated through a numerical example showing that it is an attractive alternative for closed-loop experiment design.
更多查看译文
关键词
Closed-loop identification,Optimal input design,System identification,Model Predictive Control,Constrained systems
AI 理解论文
溯源树
样例
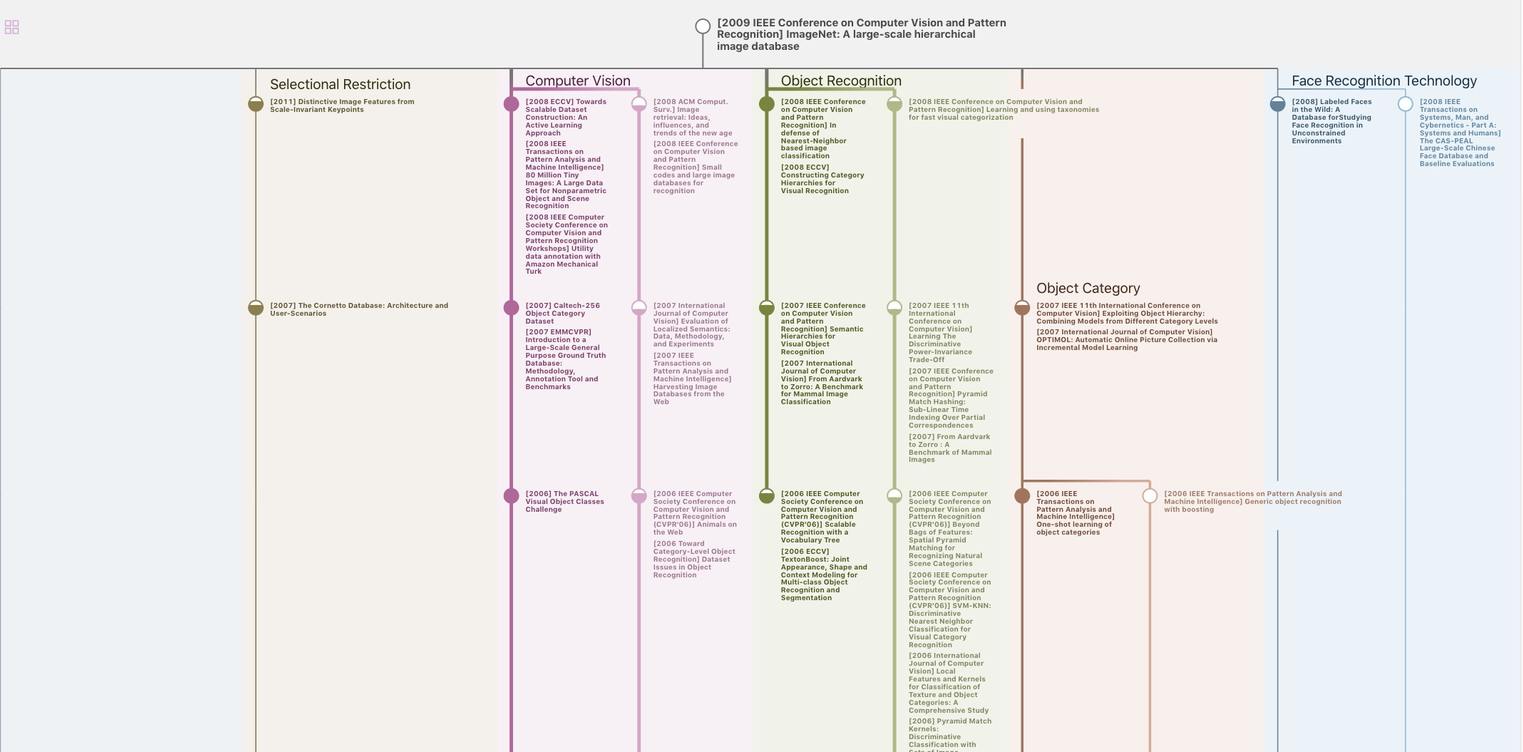
生成溯源树,研究论文发展脉络
Chat Paper
正在生成论文摘要