Modern machine learning far outperforms GLMs at predicting spikes
bioRxiv(2017)
摘要
Neuroscience has long focused on finding encoding models that effectively ask 9what predicts neural spiking?9 and generalized linear models (GLMs) are a typical approach. Modern machine learning techniques have the potential to perform better. Here we directly compared GLMs to three leading methods: feedforward neural networks, gradient boosted trees, and stacked ensembles that combine the predictions of several methods. We predicted spike counts in macaque motor (M1) and somatosensory (S1) cortices from reaching kinematics, and in rat hippocampal cells from open field location and orientation. In general, the modern methods produced far better spike predictions and were less sensitive to the preprocessing of features. XGBoost and the ensemble were the best-performing methods and worked well even on neural data with very low spike rates. This overall performance suggests that tuning curves built with GLMs are at times inaccurate and can be easily improved upon. Our publicly shared code uses standard packages and can be quickly applied to other datasets. Encoding models built with machine learning techniques more accurately predict spikes and can offer meaningful benchmarks for simpler models.
更多查看译文
关键词
encoding models,neural coding,tuning curves,machine learning,generalized linear model,GLM,spike prediction
AI 理解论文
溯源树
样例
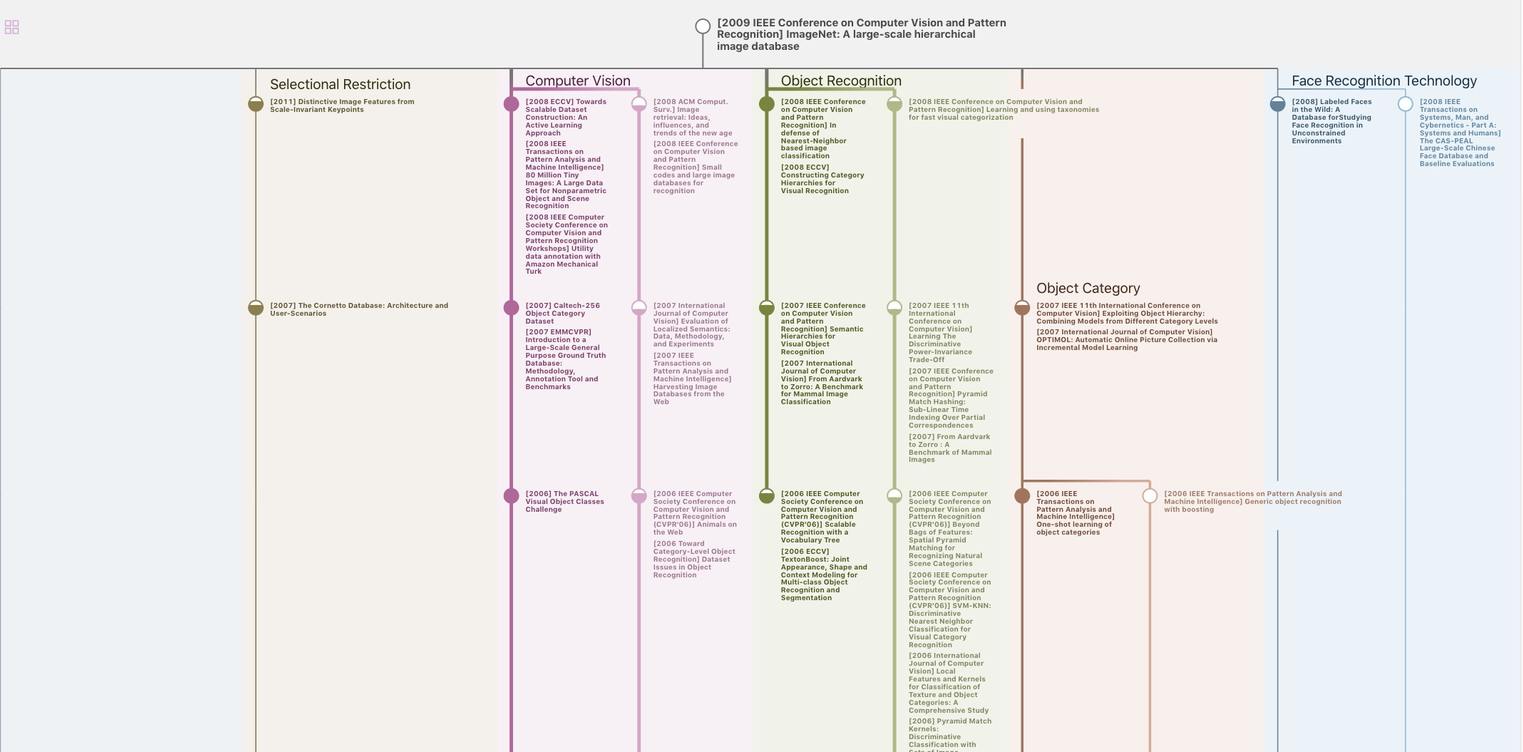
生成溯源树,研究论文发展脉络
Chat Paper
正在生成论文摘要