Evaluation of spectral pretreatments, spectral range, and regression methods for quantitative spectroscopic analysis of soil organic carbon composition
SPECTROSCOPY LETTERS(2017)
摘要
Although there is an increasing interest in using infrared spectroscopy for the simple, rapid, and inexpensive prediction of soil organic carbon content, few studies have used this technique to measure organic carbon chemistry. In this paper, based on both near-infrared and mid-infrared diffuse reflectance spectroscopy, we compared the use of instrumentation, spectral pretreatment, and regression method for the prediction of three parameters related to organic carbon content, one related to isotopic composition, and five related to organic carbon chemistry. A total of 140 soil samples collected from seven oriental oak forest sites across East China were used as the data set for the calibration-validation procedure. Calibrations using sample set partitioning based on joint x-y distances method significantly outperformed those using Kennard-Stone method. Compared to models using linear method (i.e., partial least squares), those using non-linear regression method (i.e., support vector machines) greatly improved the prediction precision of the alkyl-to-O-alkyl ratio and performed slightly better for the other organic carbon chemical compositions. Instrumentation had a large effect as mid-infrared models had higher average prediction accuracies than near-infrared models. We finally proposed a model using second derivative preprocessing, joint x-y distances based sample set partitioning, mid-infrared spectra, and support vector machines regression to quantify organic carbon chemistry in this study. The results are helpful for the further study of soil composition measurement.
更多查看译文
关键词
Infrared spectroscopy,partial least squares,soil organic carbon,support vector machines
AI 理解论文
溯源树
样例
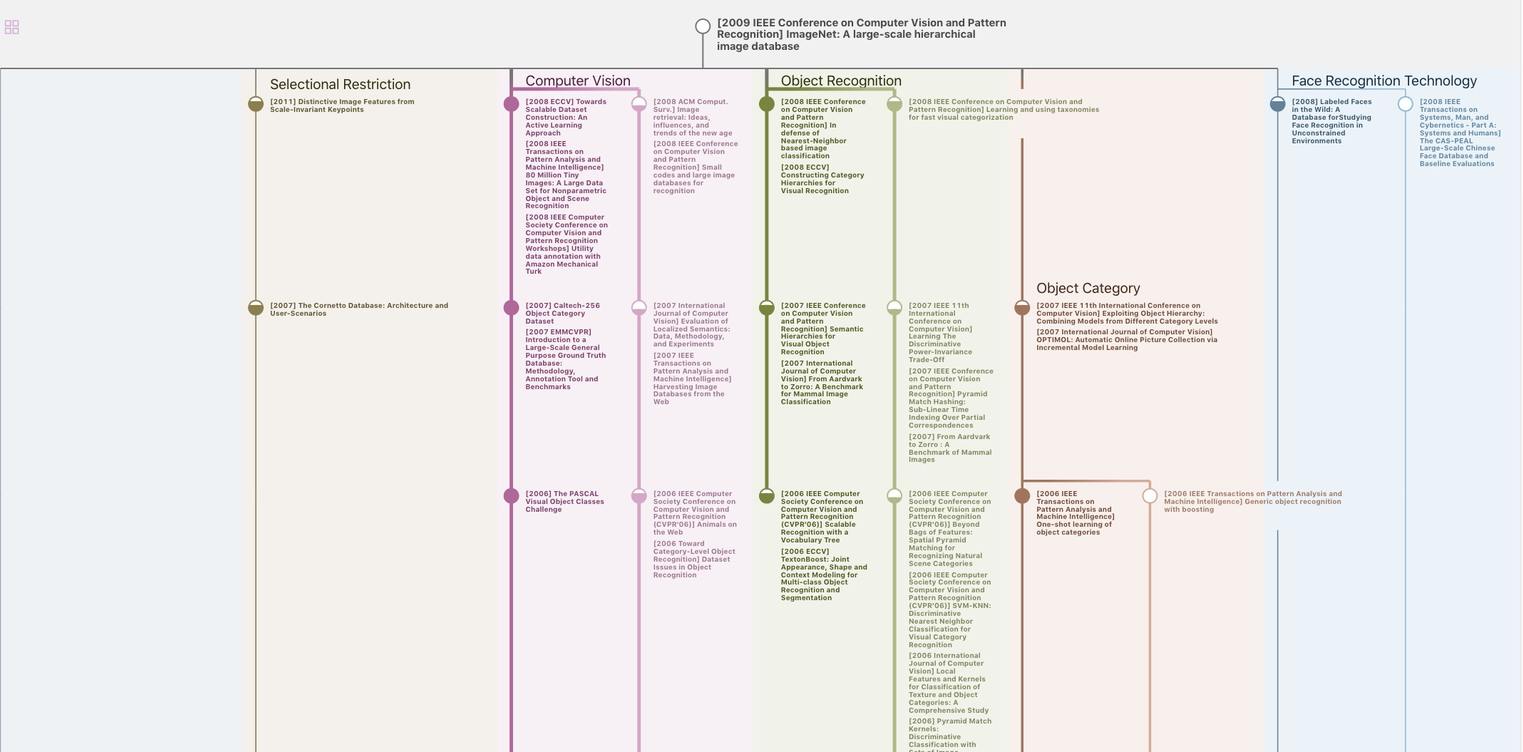
生成溯源树,研究论文发展脉络
Chat Paper
正在生成论文摘要