Predicting The Response Of Triple Negative Breast Cancer To Doxorubicin
CANCER RESEARCH(2017)
摘要
Abstracts: AACR Special Conference: Engineering and Physical Sciences in Oncology; June 25-28, 2016; Boston, MAIntroduction : The goal of this study is to establish a data-driven model of cytotoxic therapy that predicts in vitro cell population dynamics in response to a single doxorubicin dose. Whereas traditional therapy response assays are evaluated at a single timepoint following constant exposure to drug, this abstract focuses on a series of fluorescence microscopy experiments that characterize the temporal dynamics of SUM-149PT cell populations, a model of triple negative breast cancer, in response to time-resolved doxorubicin treatments. These data are then used to train a model to predict population dynamics following exposure to doxorubicin.Experimental Design : SUM-149PT cells, labeled with a nuclear fluorescent marker, were seeded in microtiter plates and allowed to reach a steady proliferation rate. Doxorubicin was then introduced at concentrations ranging from 10 nM to 2.5 μM and subsequently removed via media replacement after 6, 12, or 24 hours. These cells were imaged daily via fluorescent microscopy for 25 days following application of doxorubicin. Nuclei were segmented and counted to quantify cell population. Six replicates of each of the 28 treatment conditions were collected. A logistic growth model modified by a set of time-dependent growth functions was employed for this study. Briefly, two piecewise growth functions were implemented: a constant function and a monoexponential function. The constant function assumed an immediate transition from the pre-treatment growth rate to a stable, post-treatment rate following doxorubicin exposure. The monoexponential function allowed for a smooth induction of drug effect, while ultimately allowing for recovery of the cell population. Both models were fit to each treatment condition independently using MATLAB (Natick, MA). The area under the concentration of drug versus time curve (AUC) was used to summarize each treatment condition, allowing for comparison of data from all exposure times. The ability of the model to predict population changes following treatment at new concentrations and exposure times was then evaluated. A leave-one-out approach was employed for these predictions. For each treatment condition in the test set, the Akaike information criterion (AIC) was used to select the appropriate growth model, and the appropriate parameters were learned from the training data. The average percent error of both the best fit and predicted models are reported.Results: SUM-149PT cellular response to doxorubicin was tightly linked to both drug concentration and exposure time. Higher doses (u003e1 μM) induced rapid cell death. Smaller doses ( 15000 nM–hr). The monoexponential model was selected at intermediate AUCu0027s. The mean percent error of the AIC-selected models across all treatment conditions was 12.4 (±11.0) while the mean percent error of the predicted models was 14.9 (±13.0).Conclusion : These time-resolved treatment protocols replicate the clinically observed pharmacokinetics of cytotoxic therapies more closely than the constant concentrations in previous dose-response assays. With this data-rich approach, the in vitro response of a triple negative breast cancer cell line to doxorubicin can be predicted. Of note, different models are selected over the range of AUCu0027s to reflect the various drug mechanisms of action. This approach should allow for predictions of tumor response in animal models and, eventually, personalized, computationally-optimized treatment regimens that maximize tumor control with doxorubicin.Citation Format: Matthew T. McKenna, Stephanie L. Barnes, Jared A. Weis, Abigail M. Searfoss, Darren R. Tyson, Erin C. Rericha, Michael I. Miga, Vito Quaranta, Thomas E. Yankeelov. Predicting the response of triple negative breast cancer to doxorubicin. [abstract]. In: Proceedings of the AACR Special Conference on Engineering and Physical Sciences in Oncology; 2016 Jun 25-28; Boston, MA. Philadelphia (PA): AACR; Cancer Res 2017;77(2 Suppl):Abstract nr A14.
更多查看译文
AI 理解论文
溯源树
样例
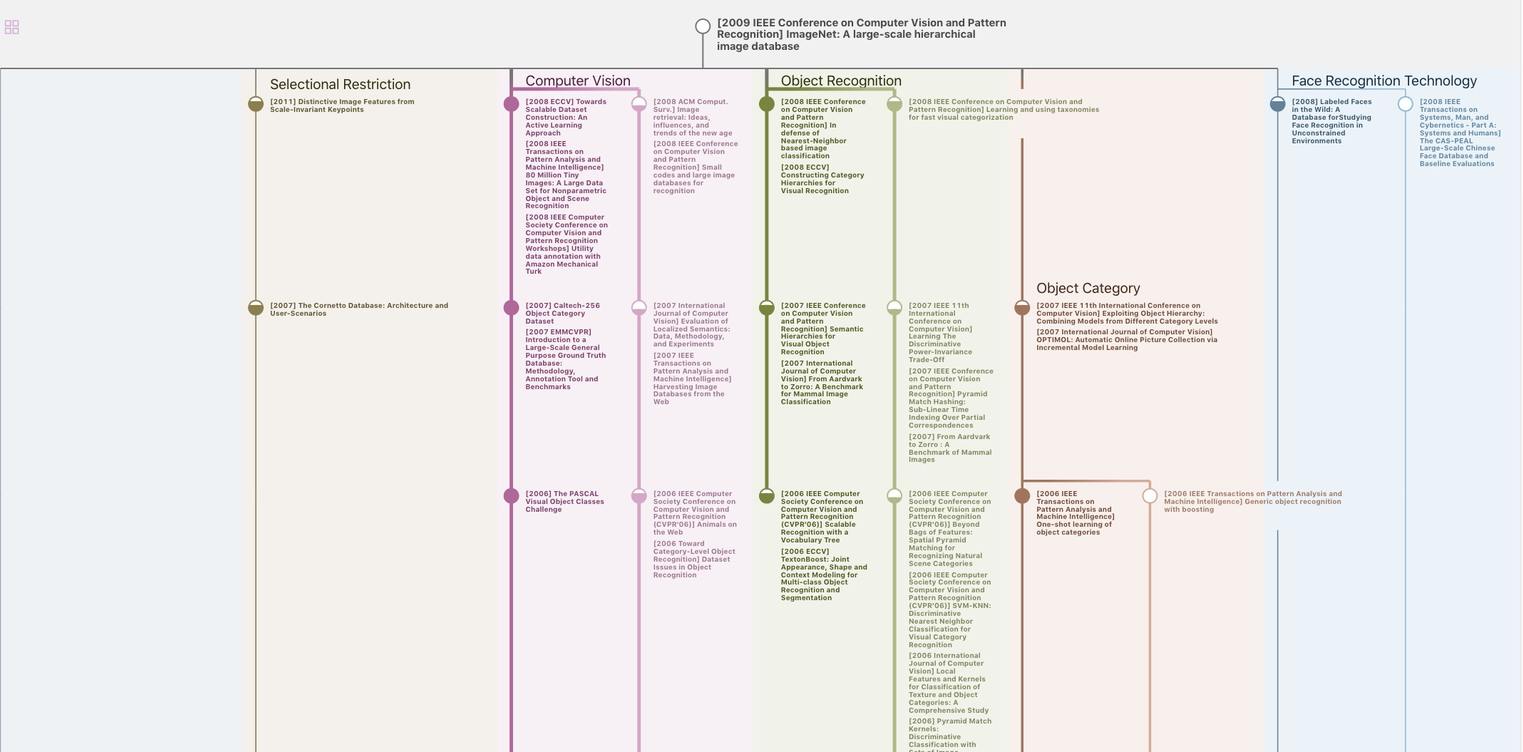
生成溯源树,研究论文发展脉络
Chat Paper
正在生成论文摘要