Faster Functional Clustering via Gaussian Mixture Models
arXiv: Computation(2016)
摘要
Functional data analysis (FDA) is an important modern paradigm for handling infinite-dimensional data. An important task in FDA is model-based clustering, which organizes functional populations into groups via subpopulation structures. The most common approach for model-based clustering of functional data is via mixtures of linear mixed-effects models. The mixture of linear mixed-effects models (MLMM) approach requires a computationally intensive algorithm for estimation. We provide a novel Gaussian mixture model (GMM) characterization of the model-based clustering problem. We demonstrate that this GMM-based characterization allows for improved computational speeds over the MLMM approach when applied via available functions in the R programming environment. Theoretical considerations for the GMM approach are discussed. An example application to a dataset based upon calcium imaging in the larval zebrafish brain is provided as a demonstration of the effectiveness of the simpler GMM approach.
更多查看译文
AI 理解论文
溯源树
样例
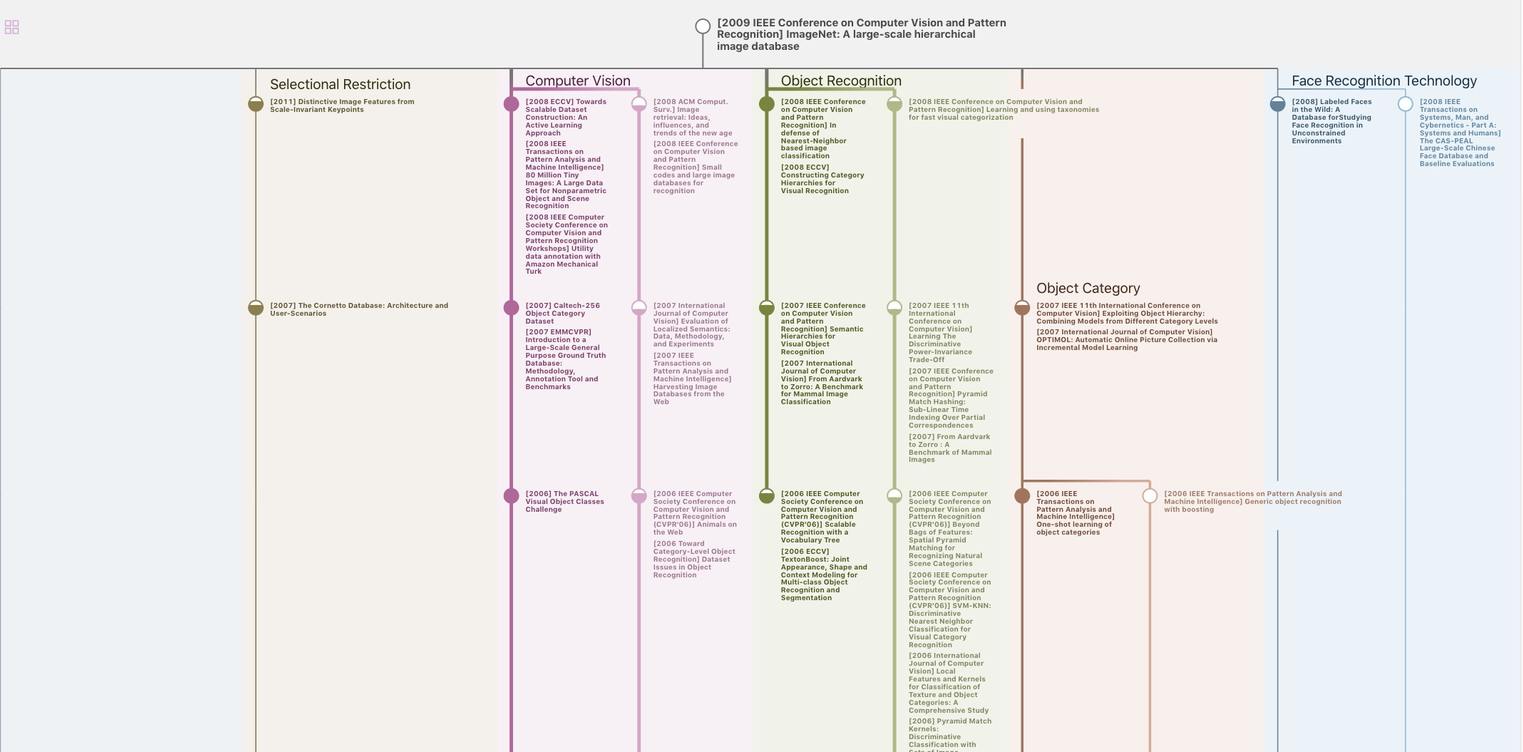
生成溯源树,研究论文发展脉络
Chat Paper
正在生成论文摘要