Nonlocal structured nonparametric Bayesian dictionary learning for image denoising
2016 IEEE 13th International Conference on Signal Processing (ICSP)(2016)
摘要
In this work, we propose a sparse Bayesian dictionary learning framework with structure prior which connects nonlocal self-similarity and sparse Bayesian dictionary learning. A Gamma-Gaussian prior is used to impose sparsity and a nonlocal beta process is utilized to introduce the nonlocal self-similarity as a structure prior for image denoising. Unlike most of the existing image denoising methods, our proposed method does not need to know noise variance in advance like an unsupervised learning. The experimental results demonstrate the effectiveness of our proposed model. It can be observed that the undesirable artifacts can be suppressed significantly and the structure of image can be preserved effectively.
更多查看译文
关键词
Nonparametric Bayesian,Image denoising,Nonlocal structure prior,Dictionary learning,Sparse coding
AI 理解论文
溯源树
样例
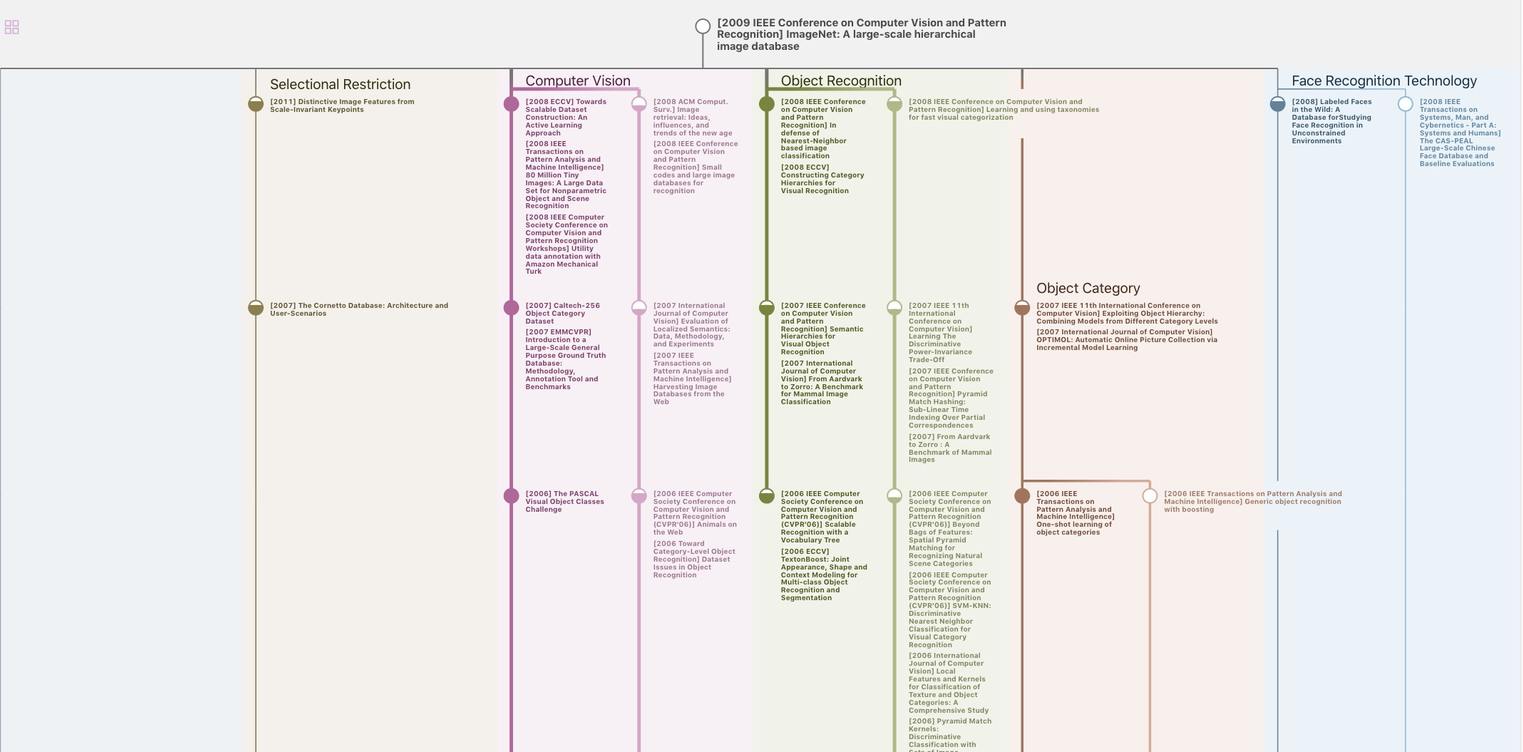
生成溯源树,研究论文发展脉络
Chat Paper
正在生成论文摘要