A component Markov regime-switching autoregressive conditional range model
Social Science Research Network(2022)
摘要
In this article, we develop one- and two-component Markov regime-switching conditional volatility models based on the intraday range and evaluate their performance in forecasting the daily volatility of the S&P 500 Index. We compare the performance of the models with that of several well-established return- and range-based volatility models, namely EWMA, GARCH, and FIGARCH models, the Markov regime-switching GARCH model, the hybrid EWMA model, and the CARR model. We evaluate the in-sample goodness of fit and out-of-sample forecast performance of the models using a comprehensive set of statistical and economic loss functions. To assess the statistical performance of the models, we use mean error metrics, directional predictive ability tests, forecast evaluation regressions, and pairwise and joint tests; and to appraise the economic performance of the models, we use value at risk coverage tests and risk management loss functions. We show that the proposed range-based Markov switching conditional volatility models produce more accurate out-of-sample forecasts, contain more information about true volatility, and exhibit similar or better performance when used for the estimation of value at risk. Our results are robust to the choice of volatility proxy, estimation sample size, out-of-sample evaluation period, and alternative error distributions.
更多查看译文
关键词
factor model, GARCH, intraday range, Markov regime-switching, multiplicative error model
AI 理解论文
溯源树
样例
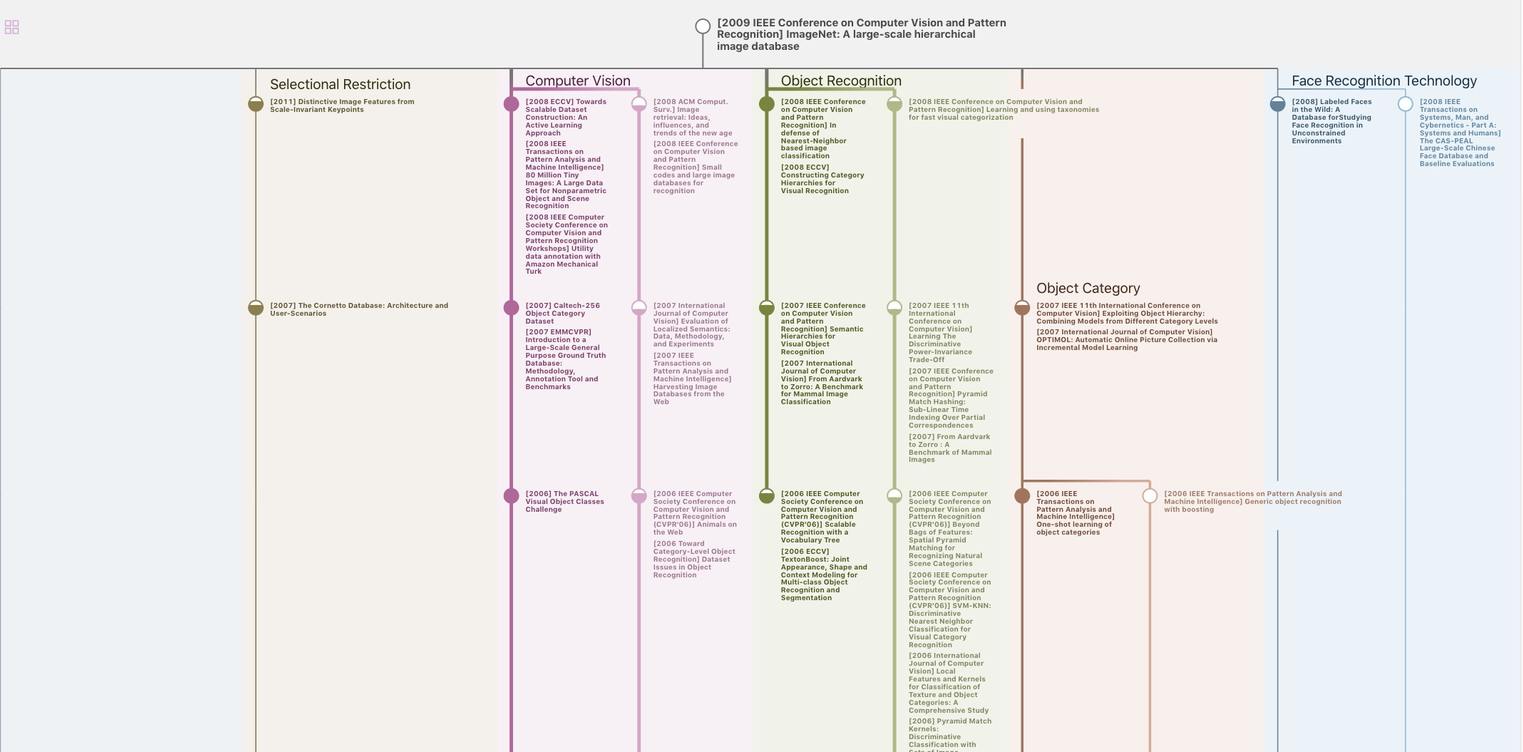
生成溯源树,研究论文发展脉络
Chat Paper
正在生成论文摘要