Global Modern Charcoal Dataset (GMCD): A tool for exploring proxy-fire linkages and spatial patterns of biomass burning
Quaternary International(2018)
摘要
Progresses in reconstructing Earth's history of biomass burning has motivated the development of a modern charcoal dataset covering the last decades through a community-based initiative called the Global Modern Charcoal Dataset (GMCD). As the frequency, intensity and spatial scale of fires are predicted to increase regionally and globally in conjunction with changing climate, anthropogenic activities and land-use patterns, there is an increasing need to further understand, calibrate and interrogate recent and past fire regimes as related to changing fire emissions and changing carbon sources and sinks. Discussions at the PAGES Global Paleofire Working Group workshop 2015, including paleoecologists, numerical modelers, statisticians, paleoclimatologists, archeologists, and anthropologists, identified an urgent need for an open, standardized, quality-controlled and globally representative dataset of modern sedimentary charcoal and other sediment-based fire proxies. This dataset fits into a gap between metrics of biomass burning indicators, current fire regimes and land cover, and carbon emissions inventories. The dataset will enable the calibration of paleofire data with other modern datasets including: data of satellite derived fire occurrence, vegetation patterns and species diversity, land cover change, and a range of sources capturing biochemical cycling. Standardized protocols are presented for collecting and analyzing sediment-based fire proxies, including charcoal, levoglucosan, black carbon, and soot. The GMCD will provide a publically-accessible repository of modern fire sediment surface samples in all terrestrial ecosystems. Sample collection and contributions to the dataset will be solicited from lacustrine, peat, marine, glacial, or other sediments, from a wide variety of ecosystems and geographic locations.
更多查看译文
关键词
Biomass burning,Standardized methodologies,Surface sample,Calibration,Dataset,Vegetation
AI 理解论文
溯源树
样例
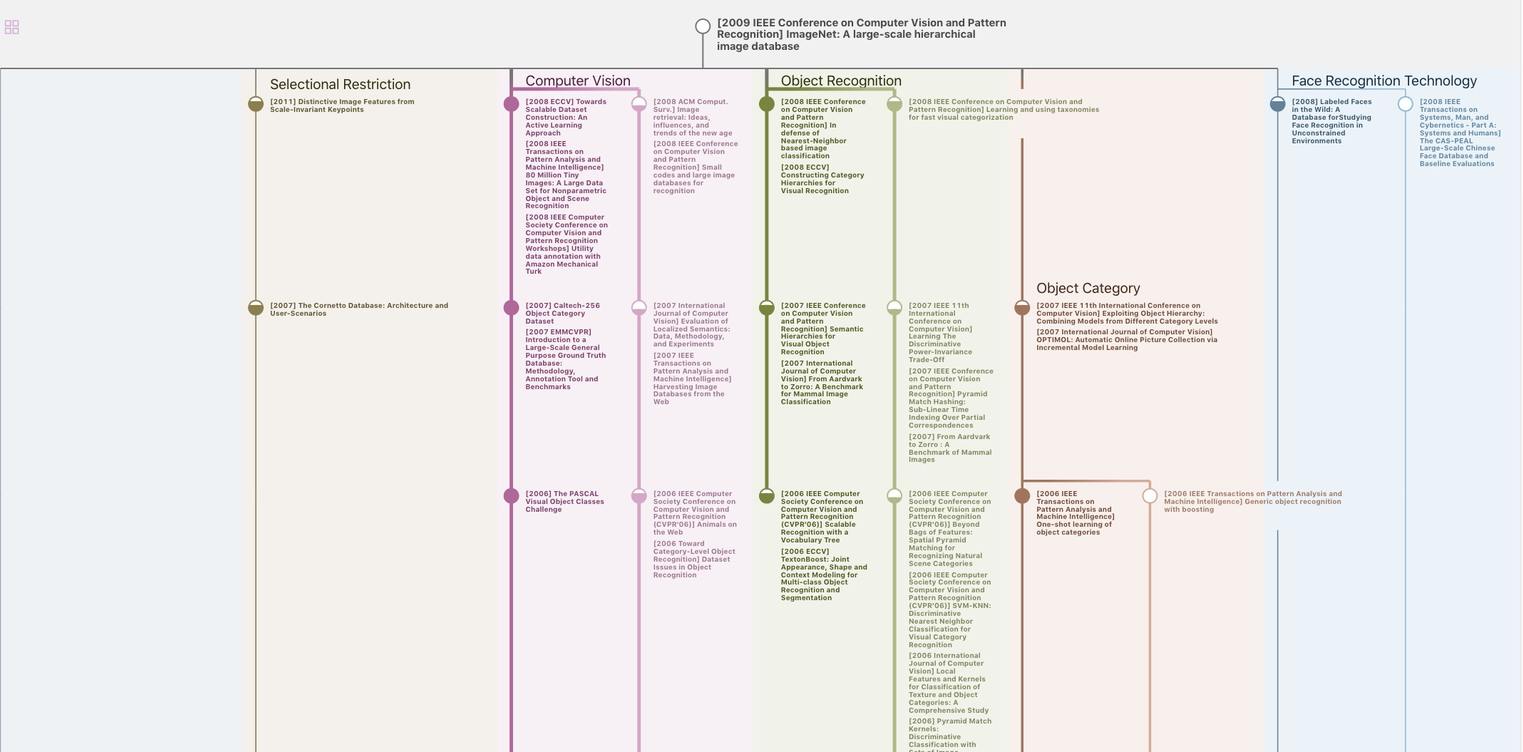
生成溯源树,研究论文发展脉络
Chat Paper
正在生成论文摘要