Bayesian System Identification using auxiliary stochastic dynamical systems
International Journal of Non-Linear Mechanics(2017)
摘要
Bayesian approaches to statistical inference and system identification became practical with the development of effective sampling methods like Markov Chain Monte Carlo (MCMC). However, because the size and complexity of inference problems has dramatically increased, improved MCMC methods are required. Dynamical systems based samplers are an effective extension of traditional MCMC methods. These samplers treat the posterior probability distribution as the potential energy function of a dynamical system, enabling them to better exploit the structure of the inference problem. We present an algorithm, Second-Order Langevin MCMC (SOL-MC), a stochastic dynamical system based MCMC algorithm, which uses the damped second-order Langevin stochastic differential equation (SDE) to sample a posterior distribution. We design the SDE such that the desired posterior probability distribution is its stationary distribution. Since this method is based upon an underlying dynamical system, we can utilize existing work to develop, implement, and optimize the sampler's performance. As such, we can choose parameters which speed up the convergence to the stationary distribution and reduce temporal state and energy correlations in the samples. We then apply this sampler to a system identification problem for a non-linear hysteretic structure model to investigate this method under globally identifiable and unidentifiable conditions.
更多查看译文
关键词
System identification,Bayesian methods,Markov Chain Monte Carlo,Stochastic differential equations,Masing hysteresis model
AI 理解论文
溯源树
样例
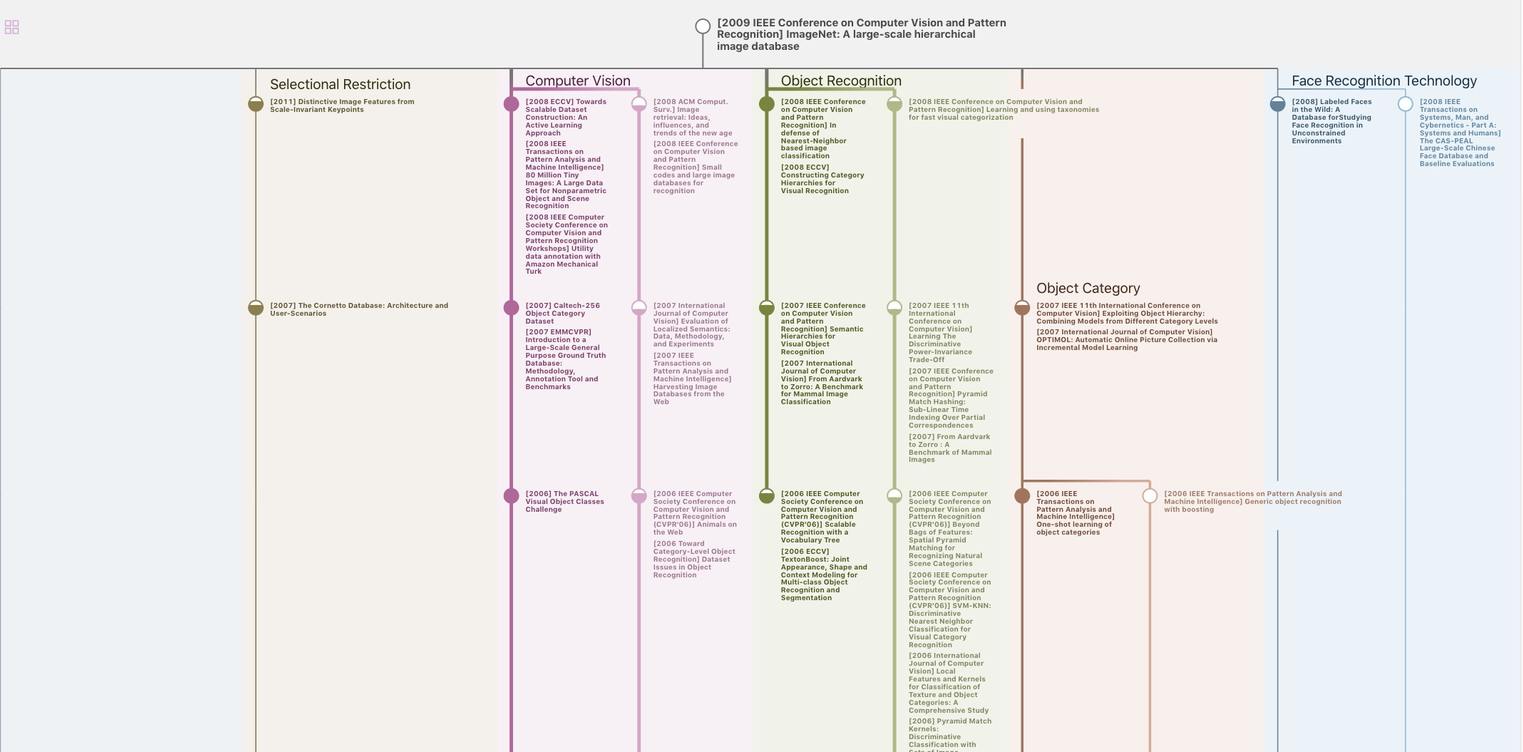
生成溯源树,研究论文发展脉络
Chat Paper
正在生成论文摘要