A kriging metamodel-assisted robust optimization method based on a reverse model
ENGINEERING OPTIMIZATION(2018)
摘要
The goal of robust optimization methods is to obtain a solution that is both optimum and relatively insensitive to uncertainty factors. Most existing robust optimization approaches use outer-inner nested optimization structures where a large amount of computational effort is required because the robustness of each candidate solution delivered from the outer level should be evaluated in the inner level. In this article, a kriging metamodel-assisted robust optimization method based on a reverse model (K-RMRO) is first proposed, in which the nested optimization structure is reduced into a single-loop optimization structure to ease the computational burden. Ignoring the interpolation uncertainties from kriging, K-RMRO may yield non-robust optima. Hence, an improved kriging-assisted robust optimization method based on a reverse model (IK-RMRO) is presented to take the interpolation uncertainty of kriging metamodel into consideration. In IK-RMRO, an objective switching criterion is introduced to determine whether the inner level robust optimization or the kriging metamodel replacement should be used to evaluate the robustness of design alternatives. The proposed criterion is developed according to whether or not the robust status of the individual can be changed because of the interpolation uncertainties from the kriging metamodel. Numerical and engineering cases are used to demonstrate the applicability and efficiency of the proposed approach.
更多查看译文
关键词
Robust optimization,reverse model,interval uncertainty,interpolation uncertainty,kriging
AI 理解论文
溯源树
样例
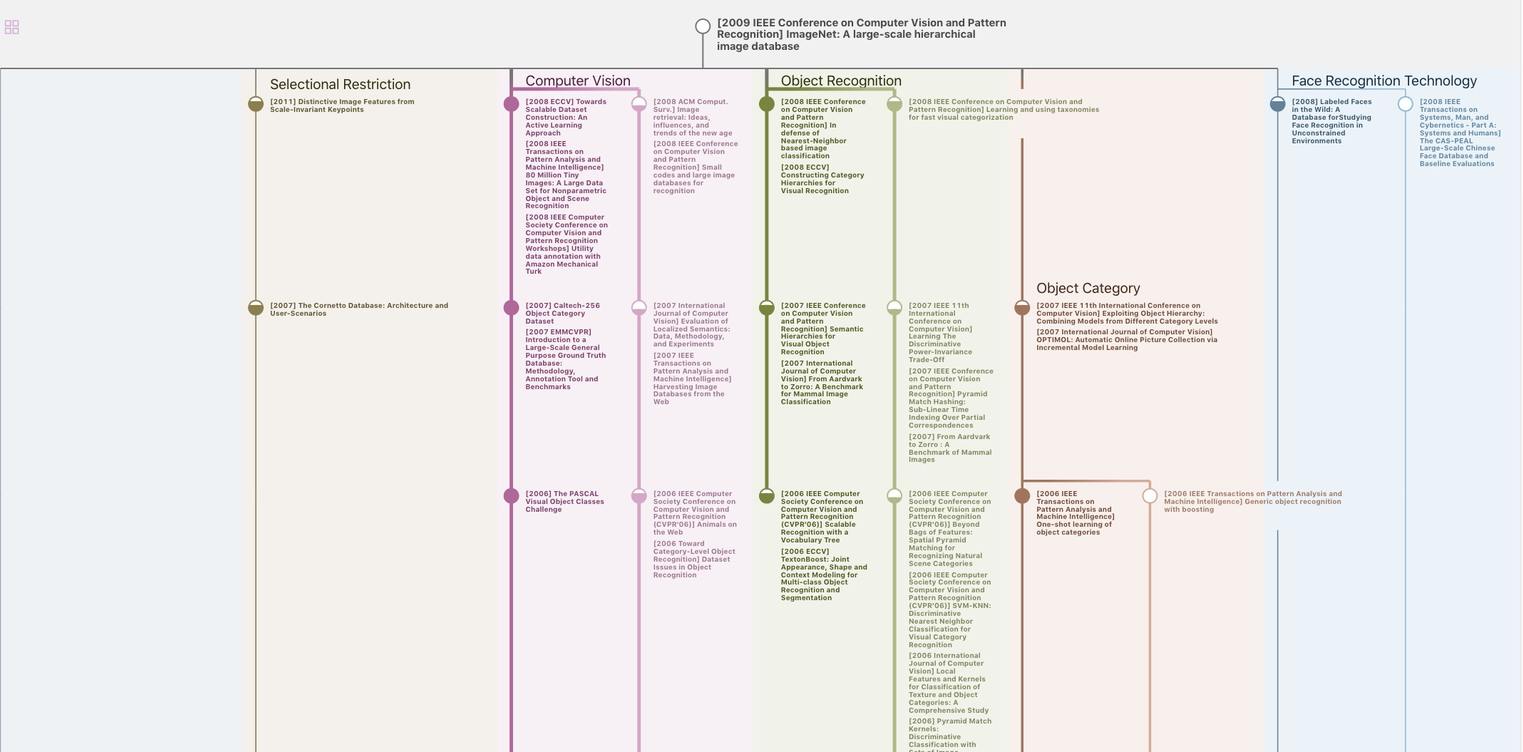
生成溯源树,研究论文发展脉络
Chat Paper
正在生成论文摘要