A Stream-Suitable Kolmogorov-Smirnov-Type Test for Big Data Analysis
arXiv: Computation(2017)
摘要
Big Data has become an ever more commonplace setting that is encountered by data analysts. In the Big Data setting, analysts are faced with very large numbers of observations as well as data that arrive as a stream, both of which are phenomena that many traditional statistical techniques are unable to contend with. Unfortunately, many of these traditional techniques are useful and cannot be discarded. One such technique is the Kolmogorov-Smirnov (KS) test for goodness-of-fit (GoF). A Big Data and stream-appropriate KS-type test is derived via the chunked-and-averaged (CA) estimator paradigm. The new test is termed the CAKS GoF test. The CAKS test statistic is proved to be asymptotically normal, allowing for the large sample testing of GoF. Furthermore, theoretical results demonstrate that the CAKS test is consistent against both fixed alternatives, where the null and the true data generating distribution are a fixed distance apart, and alternatives that approach the null at a slow enough rate. Numerical results demonstrate that the CAKS test is effective in identifying deviation in the distribution with respect to changes in mean, variance, and shape. Furthermore, it is found that the CAKS test is faster than the KS test, for large numbers of observation, and can be applied to sample sizes of 10^9 and beyond.
更多查看译文
AI 理解论文
溯源树
样例
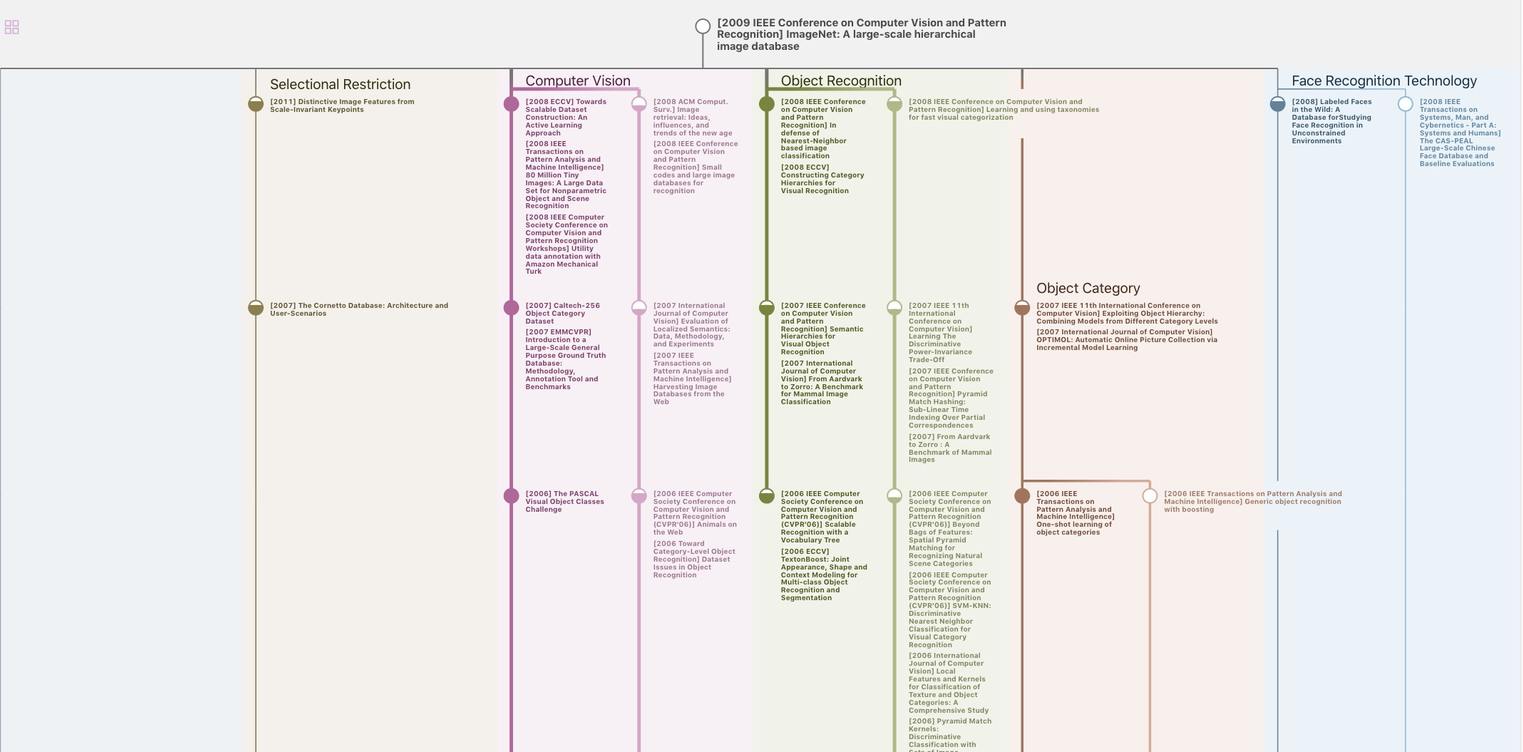
生成溯源树,研究论文发展脉络
Chat Paper
正在生成论文摘要